Decoupled spatio-temporal grouping transformer for skeleton-based action recognition
VISUAL COMPUTER(2023)
摘要
Capturing correlations between joints is crucial in skeleton-based action recognition tasks. Transformer has demonstrated its capability in capturing such correlations. However, conventional Transformer-based approaches model the relationships between joints in a unified spatio-temporal dimension, disregarding the unique semantic information that exists in both the spatial and temporal dimensions of skeleton sequences. To address this issue, we present a novel decoupled spatio-temporal grouping Transformer (DSTGFormer) model. The skeleton sequence is split into multiple spatio-temporal groups, each containing a set of consecutive frames. The spatio-temporal positional encoding (STPE) module assigns identity information to each element in the sequence. The spatio-temporal grouping self-attention (STGA) module captures the spatial and temporal relationships between different joints within a spatio-temporal group. This decoupling of the spatial and temporal dimensions enables the extraction of semantic information with different meanings in each dimension. Additionally, we propose a within-group spatial global regularization mechanism to learn more general spatial attention maps, and an inter-group feature aggregation (IGFA) module to enhance the differentiation between similar actions. Our proposed method outperforms the state-of-the-art methods on two large-scale datasets in terms of both recognition accuracy and computational efficiency.
更多查看译文
关键词
Skeleton-based action recognition,Transformer,Decoupled
AI 理解论文
溯源树
样例
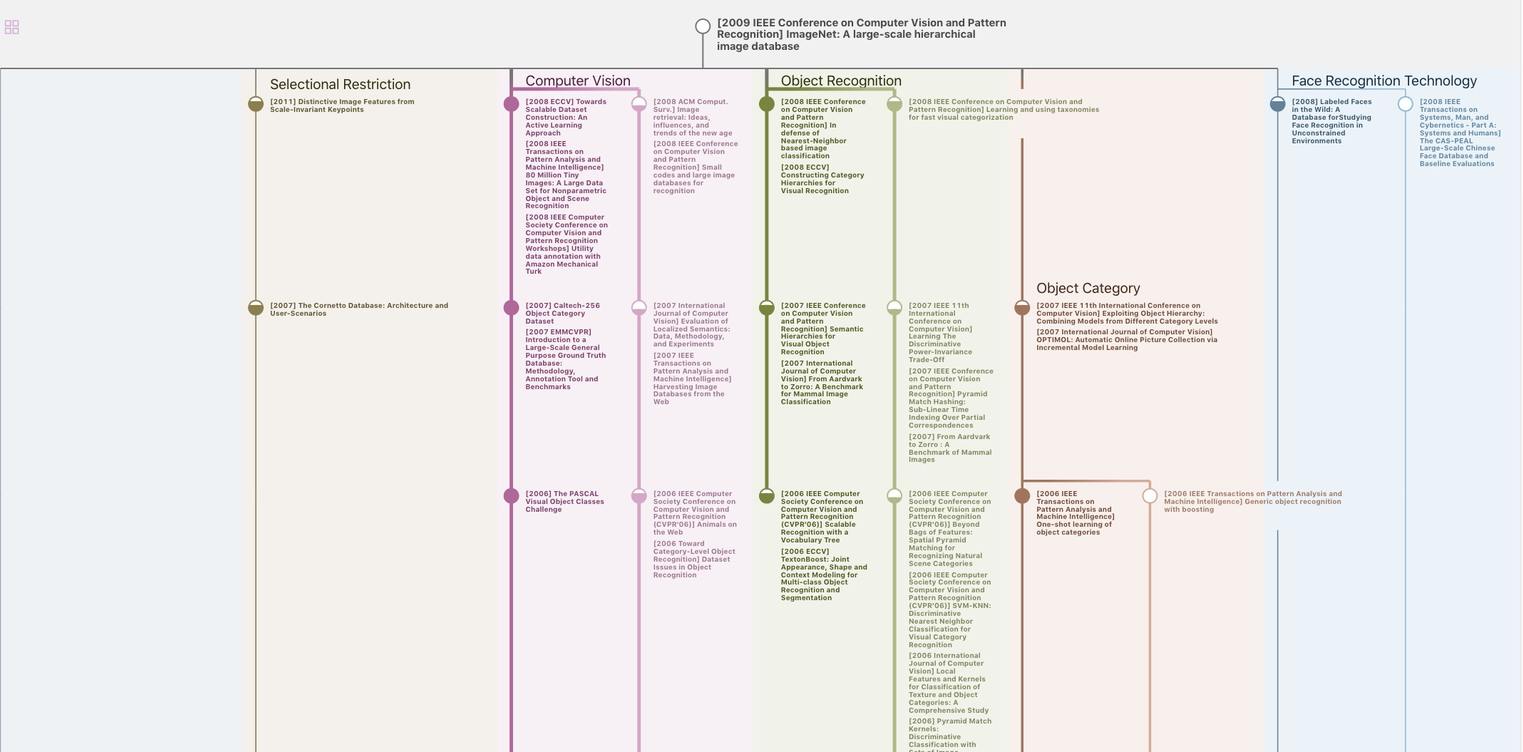
生成溯源树,研究论文发展脉络
Chat Paper
正在生成论文摘要