TimewarpVAE: Simultaneous Time-Warping and Representation Learning of Trajectories
CoRR(2023)
摘要
Human demonstrations of trajectories are an important source of training data for many machine learning problems. However, the difficulty of collecting human demonstration data for complex tasks makes learning efficient representations of those trajectories challenging. For many problems, such as for handwriting or for quasistatic dexterous manipulation, the exact timings of the trajectories should be factored from their spatial path characteristics. In this work, we propose TimewarpVAE, a fully differentiable manifold-learning algorithm that incorporates Dynamic Time Warping (DTW) to simultaneously learn both timing variations and latent factors of spatial variation. We show how the TimewarpVAE algorithm learns appropriate time alignments and meaningful representations of spatial variations in small handwriting and fork manipulation datasets. Our results have lower spatial reconstruction test error than baseline approaches and the learned low-dimensional representations can be used to efficiently generate semantically meaningful novel trajectories.
更多查看译文
关键词
trajectories,representation learning,time-warping
AI 理解论文
溯源树
样例
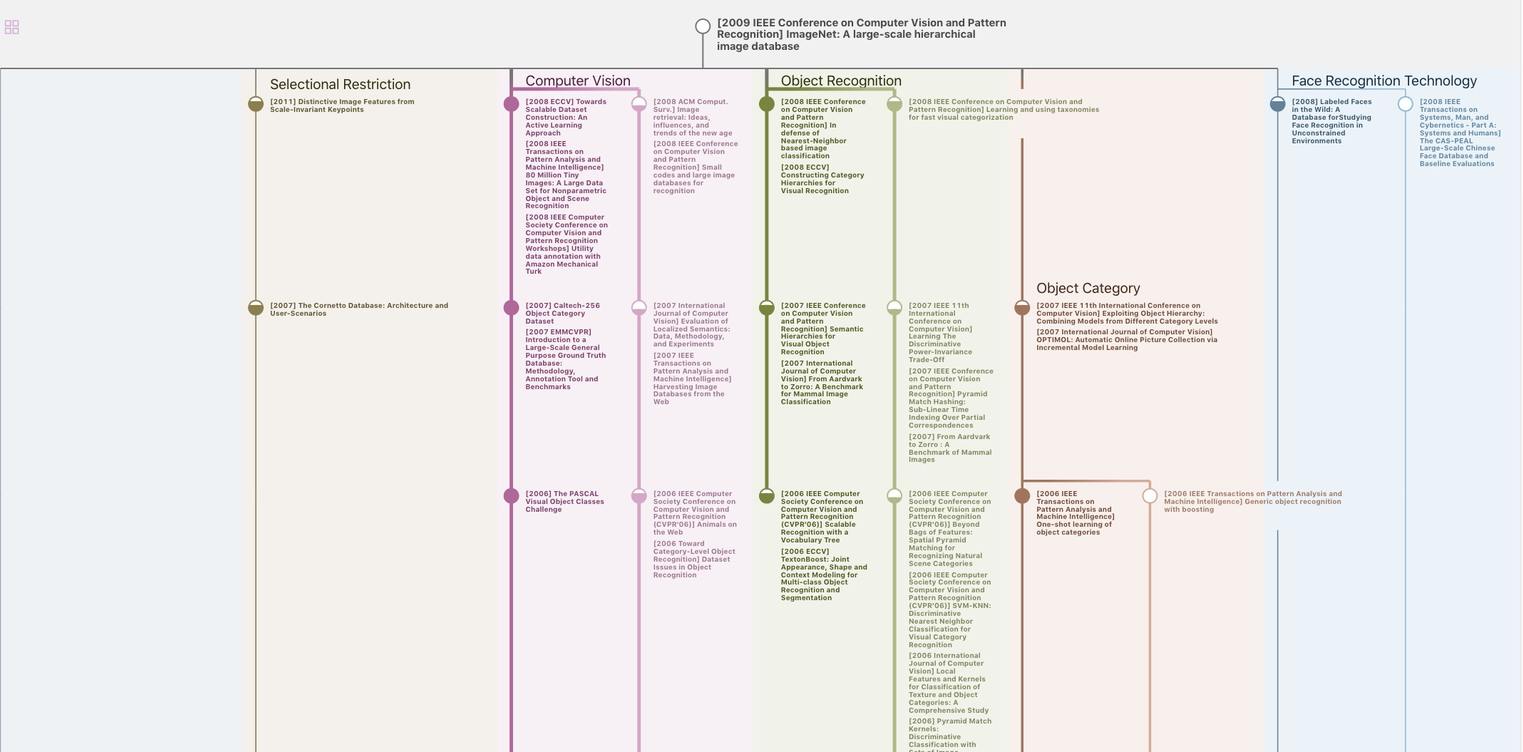
生成溯源树,研究论文发展脉络
Chat Paper
正在生成论文摘要