Knowledge-driven Meta-learning for CSI Feedback
arXiv (Cornell University)(2023)
摘要
Accurate and effective channel state information (CSI) feedback is a key technology for massive multiple-input and multiple-output systems. Recently, deep learning (DL) has been introduced for CSI feedback enhancement through massive collected training data and lengthy training time, which is quite costly and impractical for realistic deployment. In this article, a knowledge-driven meta-learning approach is proposed, where the DL model initialized by the meta model obtained from meta training phase is able to achieve rapid convergence when facing a new scenario during target retraining phase. Specifically, instead of training with massive data collected from various scenarios, the meta task environment is constructed based on the intrinsic knowledge of spatial-frequency characteristics of CSI for meta training. Moreover, the target task dataset is also augmented by exploiting the knowledge of statistical characteristics of wireless channel, so that the DL model can achieve higher performance with small actually collected dataset and short training time. In addition, we provide analyses of rationale for the improvement yielded by the knowledge in both phases. Simulation results demonstrate the superiority of the proposed approach from the perspective of feedback performance and convergence speed.
更多查看译文
关键词
CSI feedback,massive MIMO,deep learning,meta-learning,knowledge-driven
AI 理解论文
溯源树
样例
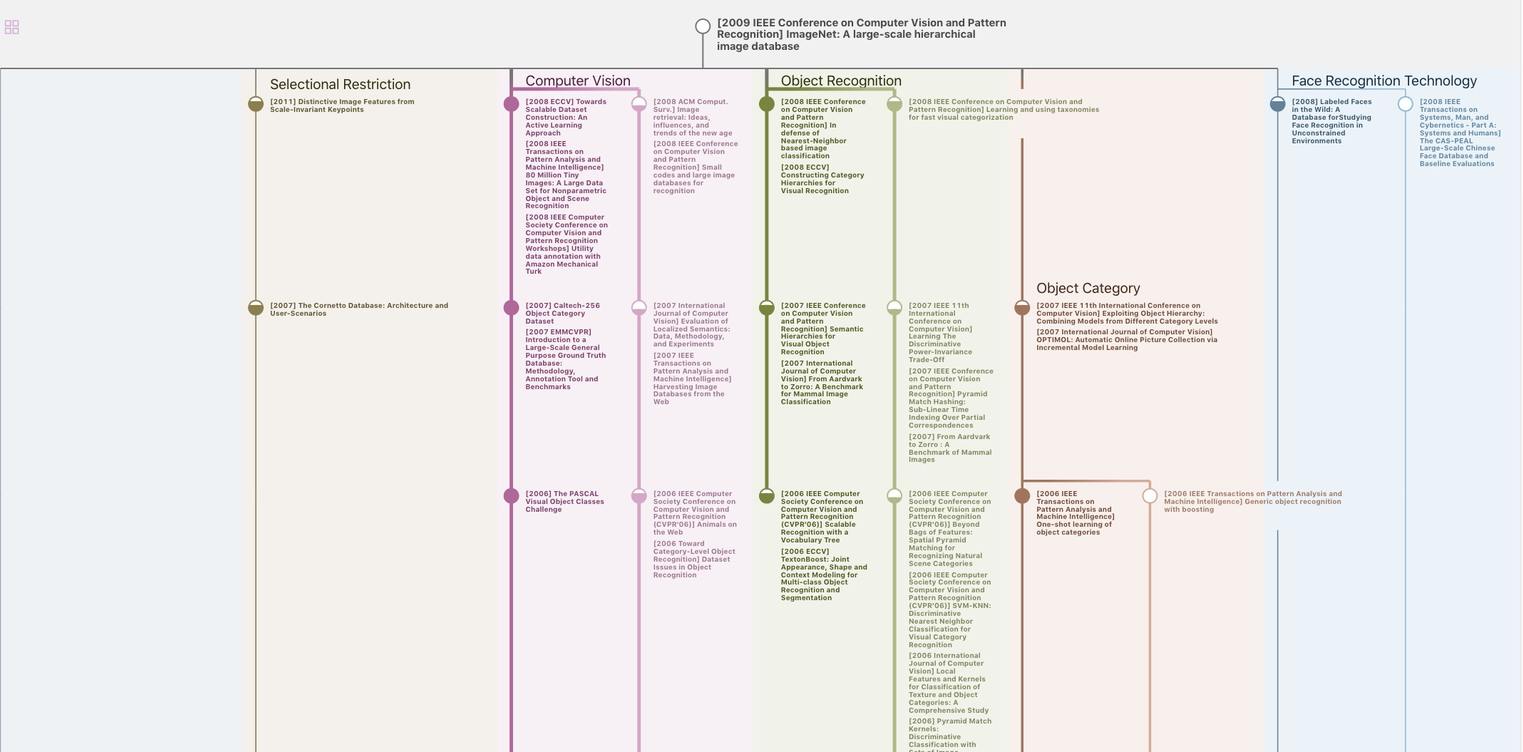
生成溯源树,研究论文发展脉络
Chat Paper
正在生成论文摘要