Modality Dropout for Multimodal Device Directed Speech Detection using Verbal and Non-Verbal Features
CoRR(2023)
摘要
Device-directed speech detection (DDSD) is the binary classification task of distinguishing between queries directed at a voice assistant versus side conversation or background speech. State-of-the-art DDSD systems use verbal cues, e.g acoustic, text and/or automatic speech recognition system (ASR) features, to classify speech as device-directed or otherwise, and often have to contend with one or more of these modalities being unavailable when deployed in real-world settings. In this paper, we investigate fusion schemes for DDSD systems that can be made more robust to missing modalities. Concurrently, we study the use of non-verbal cues, specifically prosody features, in addition to verbal cues for DDSD. We present different approaches to combine scores and embeddings from prosody with the corresponding verbal cues, finding that prosody improves DDSD performance by upto 8.5% in terms of false acceptance rate (FA) at a given fixed operating point via non-linear intermediate fusion, while our use of modality dropout techniques improves the performance of these models by 7.4% in terms of FA when evaluated with missing modalities during inference time.
更多查看译文
关键词
speech detection,multimodal device,modality,non-verbal
AI 理解论文
溯源树
样例
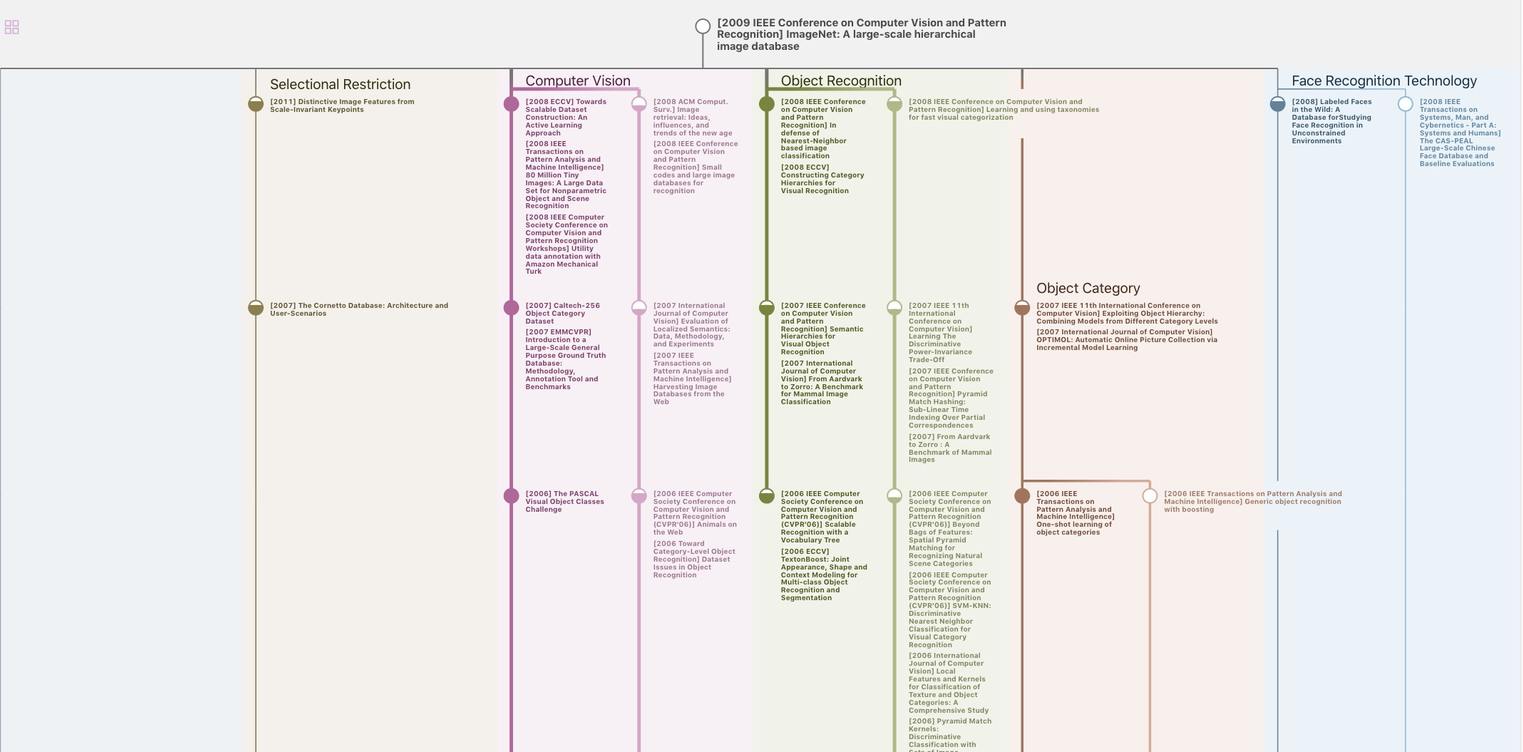
生成溯源树,研究论文发展脉络
Chat Paper
正在生成论文摘要