An Ensemble Link Prediction Framework With AUC-Guided Leaderboard Probing For Volunteer Collaboration Prediction Challenge
2023 IEEE 33rd International Workshop on Machine Learning for Signal Processing (MLSP)(2023)
摘要
This paper presents the first-place solution to the Volunteer Collaboration Prediction Challenge, addressing two primary challenges: the time-evolving nature of the network and the multi-step prediction task. To effectively capture the timeevolving characteristics of the collaboration network, we design an ensemble link prediction framework that leverages diverse topological features derived from a sequence of network snapshots. Additionally, in order to gather valuable insights for different prediction time steps, we propose an AUC-guided leaderboard probing strategy based on a unique characteristic of the testing set. The ensemble framework demonstrates exceptional performance in this competition, showcasing its effectiveness in practical volunteer collaboration prediction scenarios. Furthermore, the AUC-guided leaderboard probing strategy provides empirical evidence for potential vulnerabilities in the evaluation settings of Kaggle challenges.
更多查看译文
关键词
Kaggle competition,volunteer collaboration prediction,link prediction,ensemble learning,leader-board probing
AI 理解论文
溯源树
样例
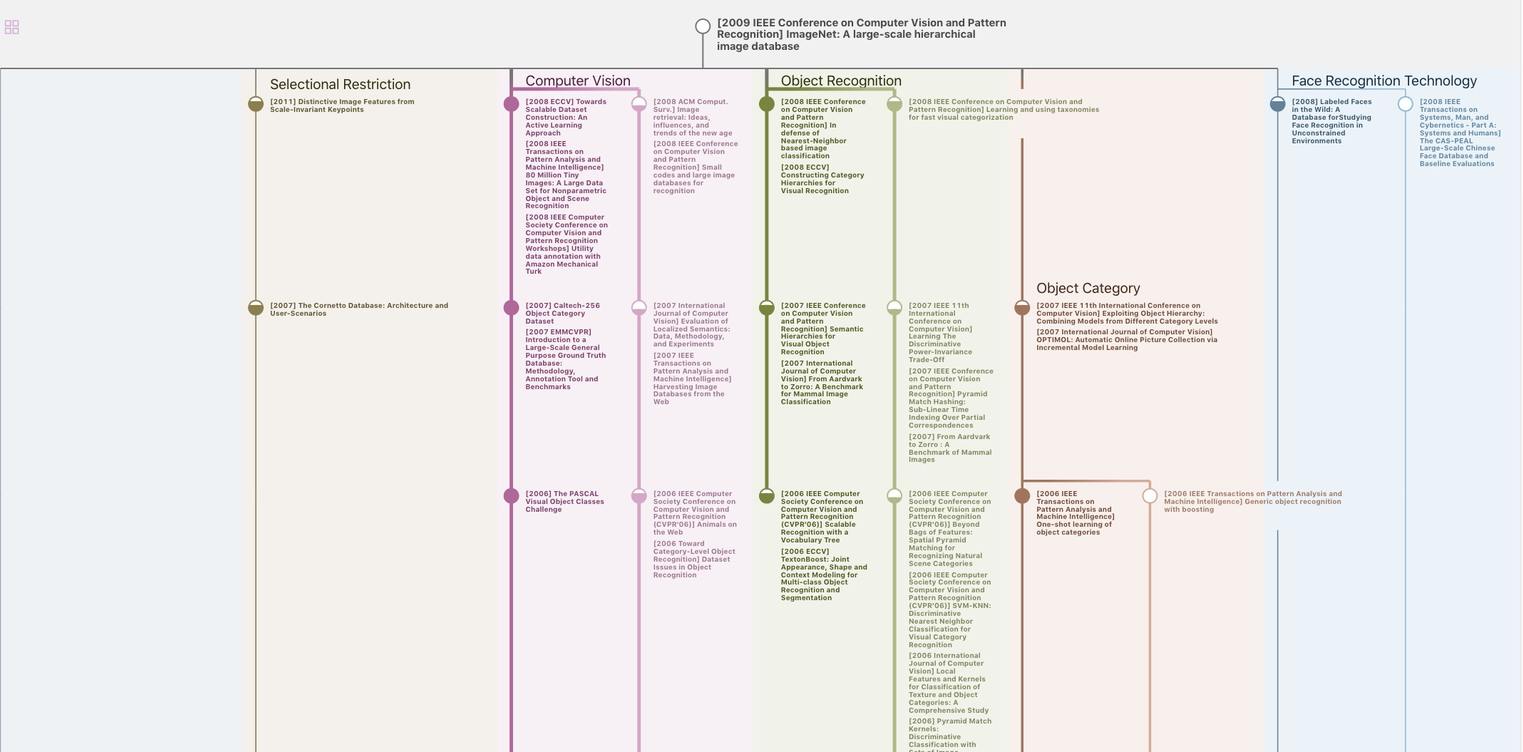
生成溯源树,研究论文发展脉络
Chat Paper
正在生成论文摘要