Open-Ended Instructable Embodied Agents with Memory-Augmented Large Language Models.
CoRR(2023)
摘要
Pre-trained and frozen LLMs can effectively map simple scene re-arrangement instructions to programs over a robot's visuomotor functions through appropriate few-shot example prompting. To parse open-domain natural language and adapt to a user's idiosyncratic procedures, not known during prompt engineering time, fixed prompts fall short. In this paper, we introduce HELPER, an embodied agent equipped with an external memory of language-program pairs that parses free-form human-robot dialogue into action programs through retrieval-augmented LLM prompting: relevant memories are retrieved based on the current dialogue, instruction, correction or VLM description, and used as in-context prompt examples for LLM querying. The memory is expanded during deployment to include pairs of user's language and action plans, to assist future inferences and personalize them to the user's language and routines. HELPER sets a new state-of-the-art in the TEACh benchmark in both Execution from Dialog History (EDH) and Trajectory from Dialogue (TfD), with 1.7x improvement over the previous SOTA for TfD. Our models, code and video results can be found in our project's website: https://helper-agent-llm.github.io.
更多查看译文
关键词
instructable embodied agents,large
AI 理解论文
溯源树
样例
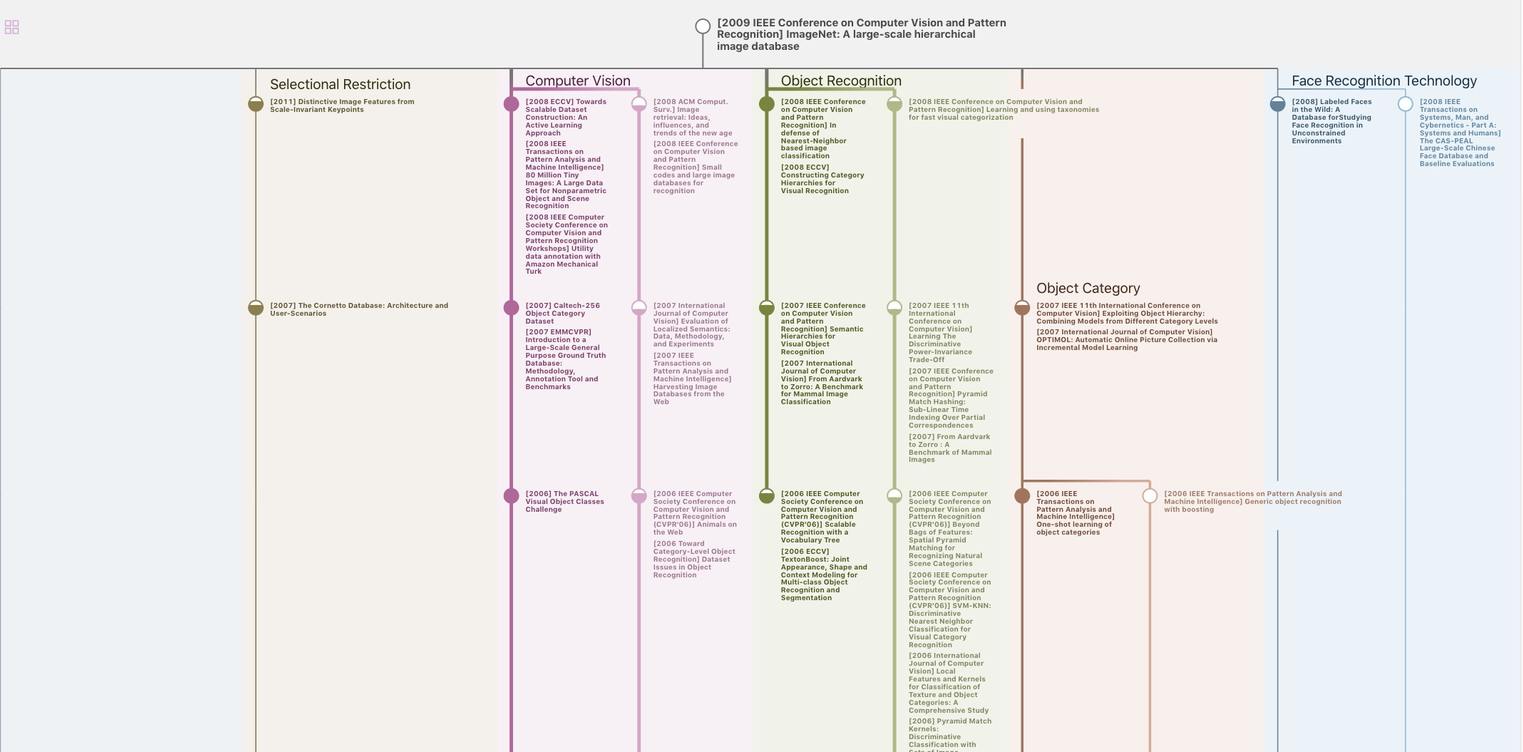
生成溯源树,研究论文发展脉络
Chat Paper
正在生成论文摘要