Deep learning denoiser assisted roughness measurements extraction from thin resists with low Signal-to-Noise Ratio (SNR) SEM images: analysis with SMILE
CoRR(2023)
摘要
The technological advance of High Numerical Aperture Extreme Ultraviolet Lithography (High NA EUVL) has opened the gates to extensive researches on thinner photoresists (below 30 similar to nm), necessary for the industrial implementation of High NA EUVL. Consequently, images from Scanning Electron Microscopy (SEM) suffer from reduced imaging contrast and low Signal-to-Noise Ratio (SNR), impacting the measurement of unbiased Line Edge Roughness (uLER) and Line Width Roughness (uLWR). Thus, the aim of this work is to enhance the SNR of SEM images by using a Deep Learning denoiser and enable robust roughness extraction of the thin resist. For this study, we acquired SEM images of Line-Space (L/S) patterns with a Chemically Amplified Resist (CAR) with different thicknesses (15 similar to nm, 20 similar to nm, 25 similar to nm, 30 similar to nm), underlayers (Spin-On-Glass - SOG, Organic Underlayer - OUL) and frames of averaging (4, 8, 16, 32, and 64 similar to Fr). After denoising, a systematic analysis has been carried out on both noisy and denoised images using an open-source metrology software, SMILE 2.3.2, for investigating mean CD, SNR improvement factor, biased and unbiased LWR/LER Power Spectral Density (PSD). Denoised images with lower number of frames present unaltered Critical Dimensions (CDs), enhanced SNR (especially for low number of integration frames), and accurate measurements of uLER and uLWR, with the same accuracy as for noisy images with a consistent higher number of frames. Therefore, images with a small number of integration frames and with SNR similar to< 2 can be successfully denoised, and advantageously used in improving metrology throughput while maintaining reliable roughness measurements for the thin resist.
更多查看译文
关键词
Denoising,machine learning,deep learning,thin resist,High NA EUVL,e-beam metrology,line edge/width roughness,Power Spectral Density (PSD)
AI 理解论文
溯源树
样例
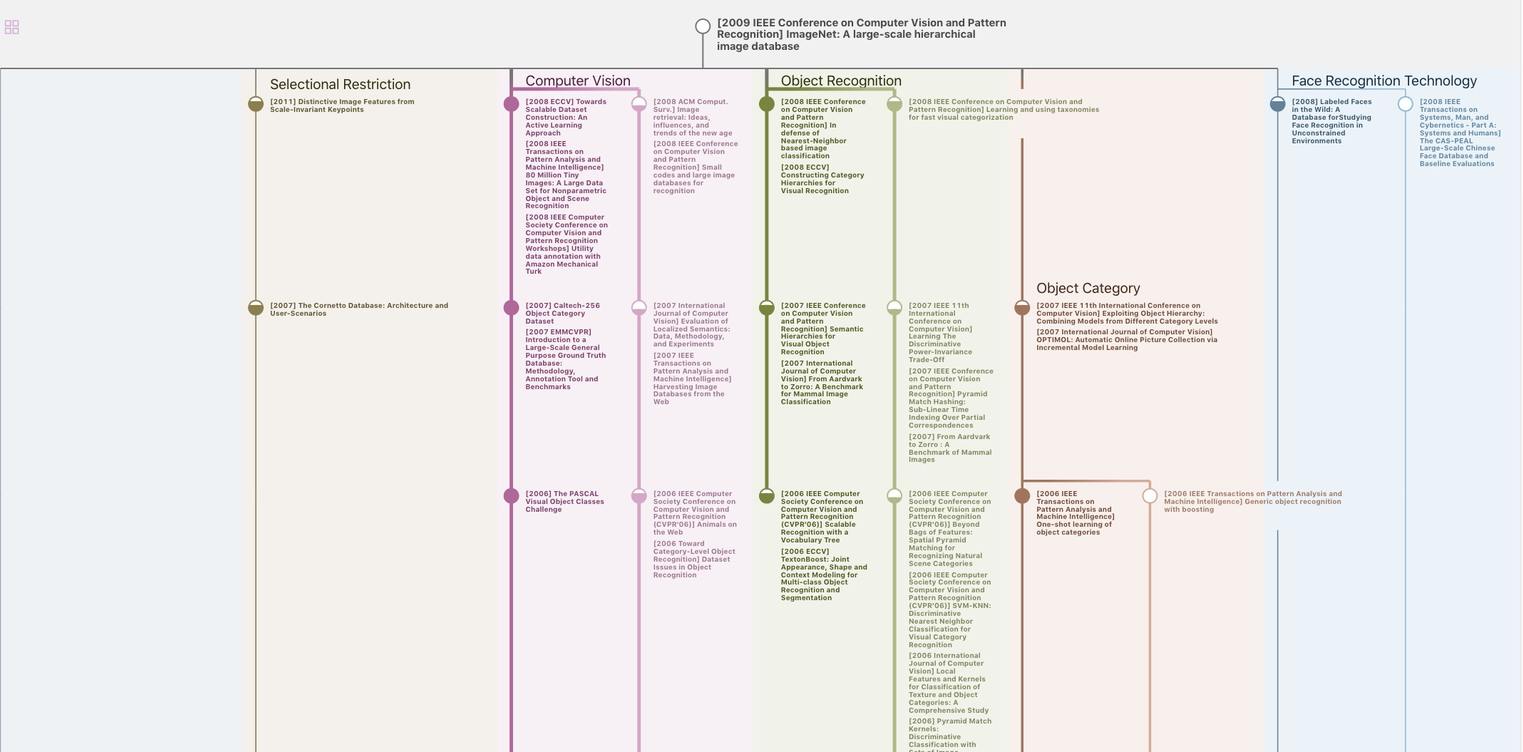
生成溯源树,研究论文发展脉络
Chat Paper
正在生成论文摘要