Turn-Level Active Learning for Dialogue State Tracking.
CoRR(2023)
摘要
Dialogue state tracking (DST) plays an important role in task-oriented dialogue systems. However, collecting a large amount of turn-by-turn annotated dialogue data is costly and inefficient. In this paper, we propose a novel turn-level active learning framework for DST to actively select turns in dialogues to annotate. Given the limited labelling budget, experimental results demonstrate the effectiveness of selective annotation of dialogue turns. Additionally, our approach can effectively achieve comparable DST performance to traditional training approaches with significantly less annotated data, which provides a more efficient way to annotate new dialogue data.
更多查看译文
关键词
dialogue state tracking,learning,turn-level
AI 理解论文
溯源树
样例
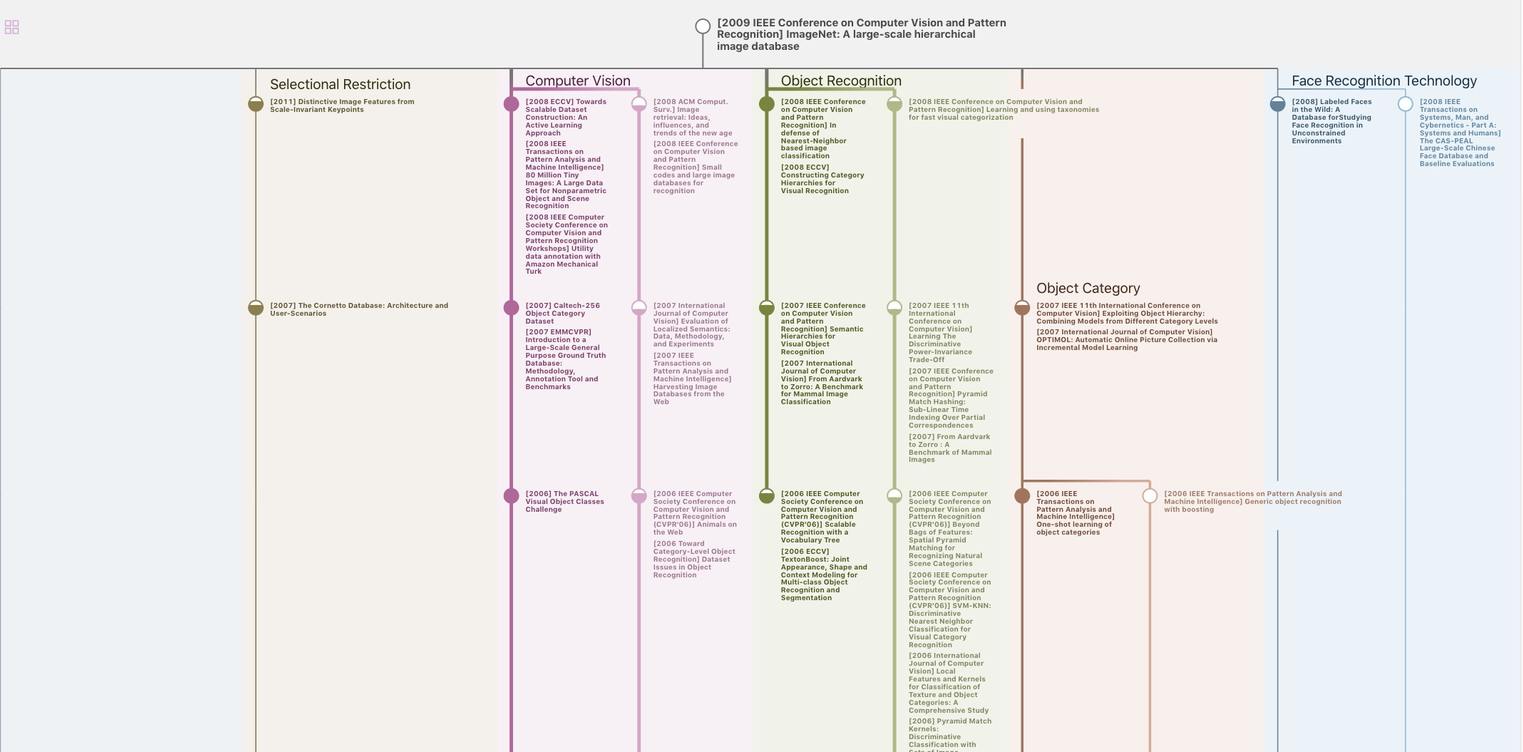
生成溯源树,研究论文发展脉络
Chat Paper
正在生成论文摘要