A Specialized Semismooth Newton Method for Kernel-Based Optimal Transport
CoRR(2023)
摘要
Kernel-based optimal transport (OT) estimators offer an alternative,
functional estimation procedure to address OT problems from samples. Recent
works suggest that these estimators are more statistically efficient than
plug-in (linear programming-based) OT estimators when comparing probability
measures in high-dimensions . Unfortunately, that
statistical benefit comes at a very steep computational price: because their
computation relies on the short-step interior-point method (SSIPM), which comes
with a large iteration count in practice, these estimators quickly become
intractable w.r.t. sample size n. To scale these estimators to larger n, we
propose a nonsmooth fixed-point model for the kernel-based OT problem, and show
that it can be efficiently solved via a specialized semismooth Newton (SSN)
method: We show, exploring the problem's structure, that the per-iteration cost
of performing one SSN step can be significantly reduced in practice. We prove
that our SSN method achieves a global convergence rate of O(1/√(k)), and
a local quadratic convergence rate under standard regularity conditions. We
show substantial speedups over SSIPM on both synthetic and real datasets.
更多查看译文
关键词
specialized semismooth newton method,transport,optimal
AI 理解论文
溯源树
样例
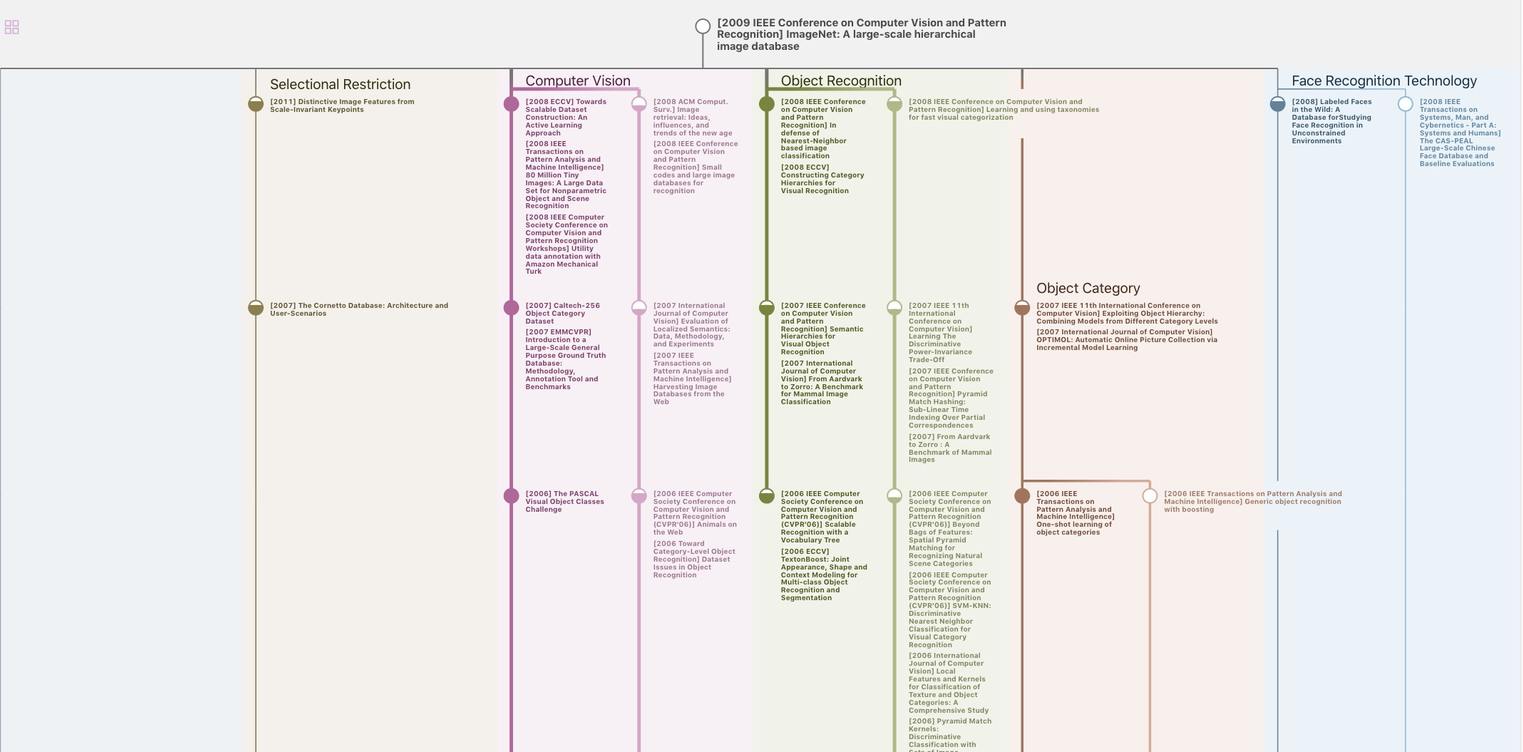
生成溯源树,研究论文发展脉络
Chat Paper
正在生成论文摘要