Adaptive, Doubly Optimal No-Regret Learning in Strongly Monotone and Exp-Concave Games with Gradient Feedback
arxiv(2023)
摘要
Online gradient descent (OGD) is well known to be doubly optimal under strong
convexity or monotonicity assumptions: (1) in the single-agent setting, it
achieves an optimal regret of Θ(log T) for strongly convex cost
functions; and (2) in the multi-agent setting of strongly monotone games, with
each agent employing OGD, we obtain last-iterate convergence of the joint
action to a unique Nash equilibrium at an optimal rate of
Θ(1/T). While these finite-time guarantees highlight its merits,
OGD has the drawback that it requires knowing the strong convexity/monotonicity
parameters. In this paper, we design a fully adaptive OGD algorithm,
, that does not require a priori knowledge of these parameters.
In the single-agent setting, our algorithm achieves O(log^2(T)) regret under
strong convexity, which is optimal up to a log factor. Further, if each agent
employs in strongly monotone games, the joint action converges
in a last-iterate sense to a unique Nash equilibrium at a rate of
O(log^3 T/T), again optimal up to log factors. We illustrate our
algorithms in a learning version of the classical newsvendor problem, where due
to lost sales, only (noisy) gradient feedback can be observed. Our results
immediately yield the first feasible and near-optimal algorithm for both the
single-retailer and multi-retailer settings. We also extend our results to the
more general setting of exp-concave cost functions and games, using the online
Newton step (ONS) algorithm.
更多查看译文
AI 理解论文
溯源树
样例
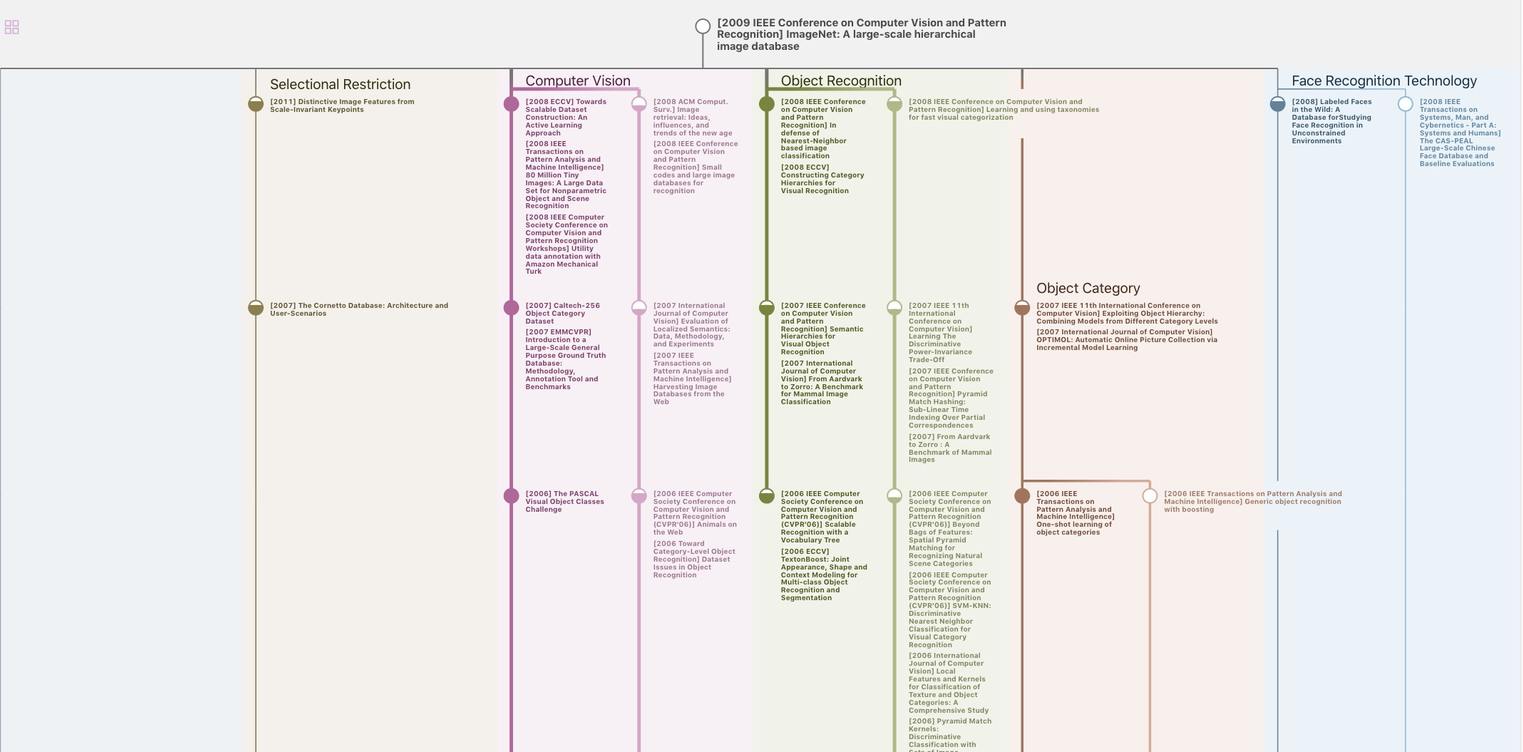
生成溯源树,研究论文发展脉络
Chat Paper
正在生成论文摘要