Cold Diffusion on the Replay Buffer: Learning to Plan from Known Good States
CoRR(2023)
摘要
Learning from demonstrations (LfD) has successfully trained robots to exhibit remarkable generalization capabilities. However, many powerful imitation techniques do not prioritize the feasibility of the robot behaviors they generate. In this work, we explore the feasibility of plans produced by LfD. As in prior work, we employ a temporal diffusion model with fixed start and goal states to facilitate imitation through in-painting. Unlike previous studies, we apply cold diffusion to ensure the optimization process is directed through the agent's replay buffer of previously visited states. This routing approach increases the likelihood that the final trajectories will predominantly occupy the feasible region of the robot's state space. We test this method in simulated robotic environments with obstacles and observe a significant improvement in the agent's ability to avoid these obstacles during planning.
更多查看译文
关键词
replay buffer,diffusion,learning
AI 理解论文
溯源树
样例
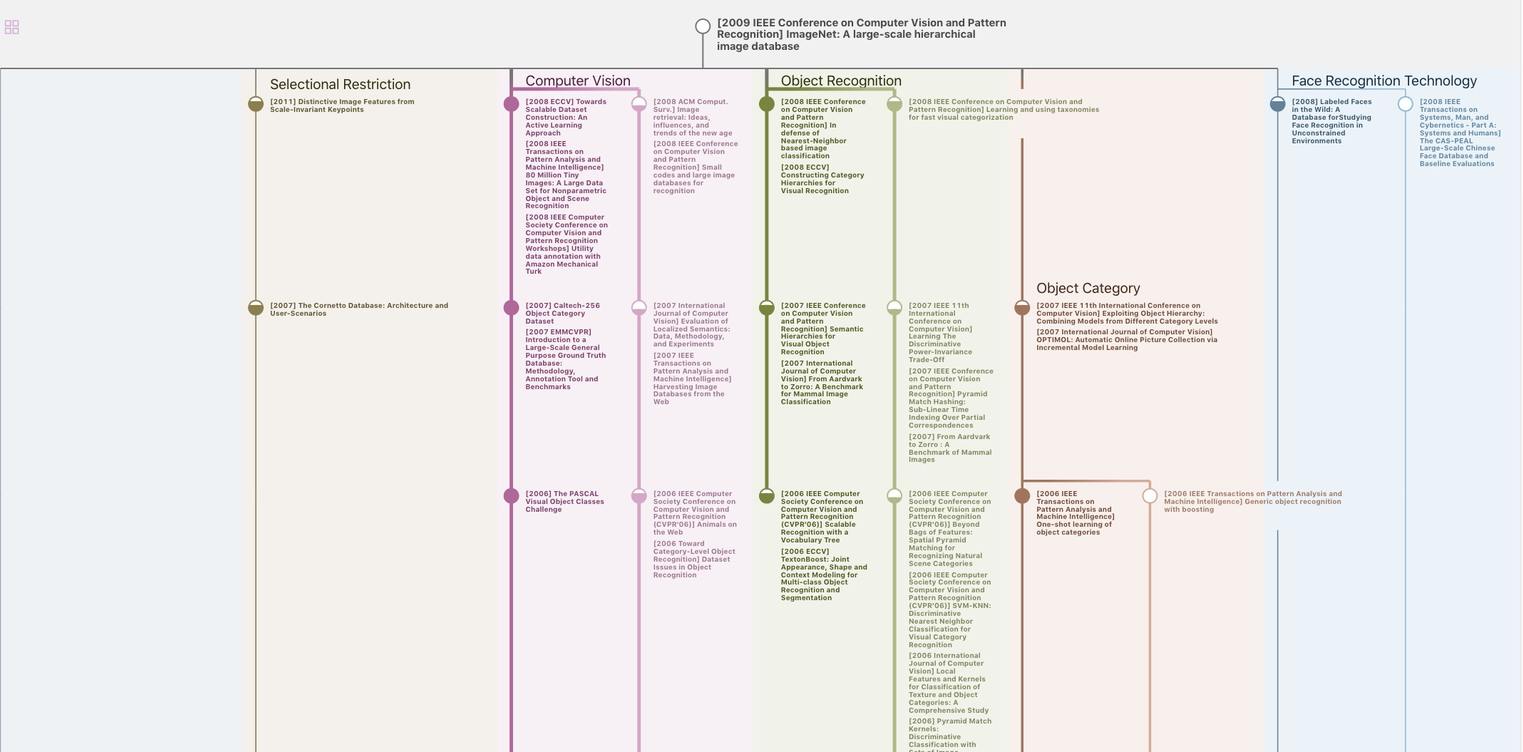
生成溯源树,研究论文发展脉络
Chat Paper
正在生成论文摘要