Enhanced Low-Dimensional Sensing Mapless Navigation of Terrestrial Mobile Robots Using Double Deep Reinforcement Learning Techniques
2023 LATIN AMERICAN ROBOTICS SYMPOSIUM, LARS, 2023 BRAZILIAN SYMPOSIUM ON ROBOTICS, SBR, AND 2023 WORKSHOP ON ROBOTICS IN EDUCATION, WRE(2023)
摘要
In this study, we present two distinct approaches of Deep Reinforcement Learning (Deep-RL) algorithms for a mobile robot. The research methodology primarily involves a comparative analysis between a Deep-RL strategy grounded in the foundational Deep Q-Network (DQN) algorithm, and the Double Deep Q-Network (DDQN) algorithm. The agents in these approaches leverage 24 measurements from laser range sampling, coupled with the agent's positional differentials and orientation relative to the target. This amalgamation of data influences the agents' determinations regarding navigation, ultimately dictating the robot's velocities. By embracing this parsimonious sensory framework as proposed, we successfully showcase the training of an agent for proficiently executing navigation tasks and adeptly circumventing obstacles. Notably, this accomplishment is attained without a dependency on intricate sensory inputs like those inherent to image-centric methodologies. The proposed methodology is evaluated in three different real environments, revealing that Double Deep structures significantly enhance the navigation capabilities of mobile robots compared to simple Q structures.
更多查看译文
关键词
deep reinforcement learning,mobile robots,sensing,low-dimensional
AI 理解论文
溯源树
样例
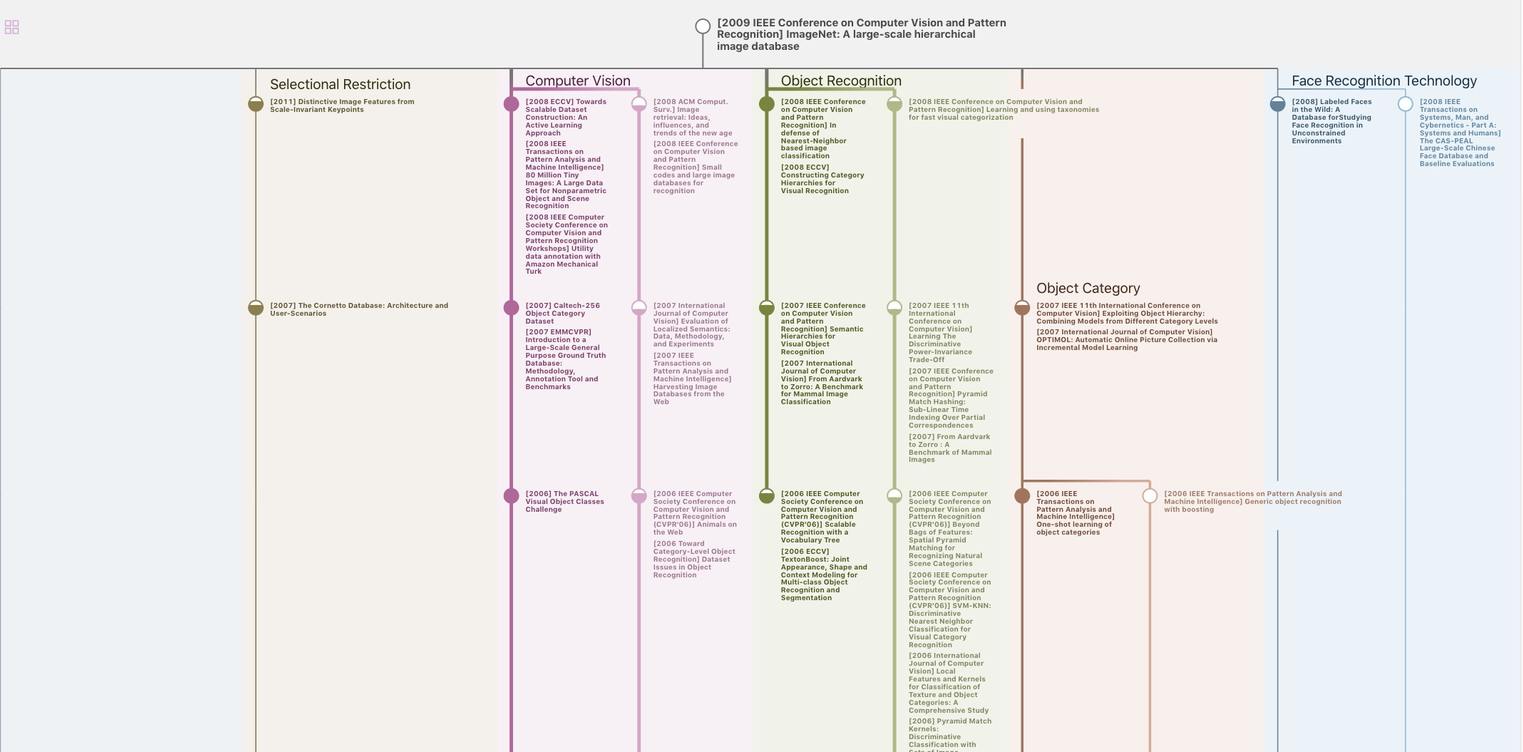
生成溯源树,研究论文发展脉络
Chat Paper
正在生成论文摘要