Impact of Guidance and Interaction Strategies for LLM Use on Learner Performance and Perception
CoRR(2023)
摘要
Personalized chatbot-based teaching assistants can be crucial in addressing
increasing classroom sizes, especially where direct teacher presence is
limited. Large language models (LLMs) offer a promising avenue, with increasing
research exploring their educational utility. However, the challenge lies not
only in establishing the efficacy of LLMs but also in discerning the nuances of
interaction between learners and these models, which impact learners'
engagement and results. We conducted a formative study in an undergraduate
computer science classroom (N=145) and a controlled experiment on Prolific
(N=356) to explore the impact of four pedagogically informed guidance
strategies on the learners' performance, confidence and trust in LLMs. Direct
LLM answers marginally improved performance, while refining student solutions
fostered trust. Structured guidance reduced random queries as well as instances
of students copy-pasting assignment questions to the LLM. Our work highlights
the role that teachers can play in shaping LLM-supported learning environments.
更多查看译文
关键词
learner
AI 理解论文
溯源树
样例
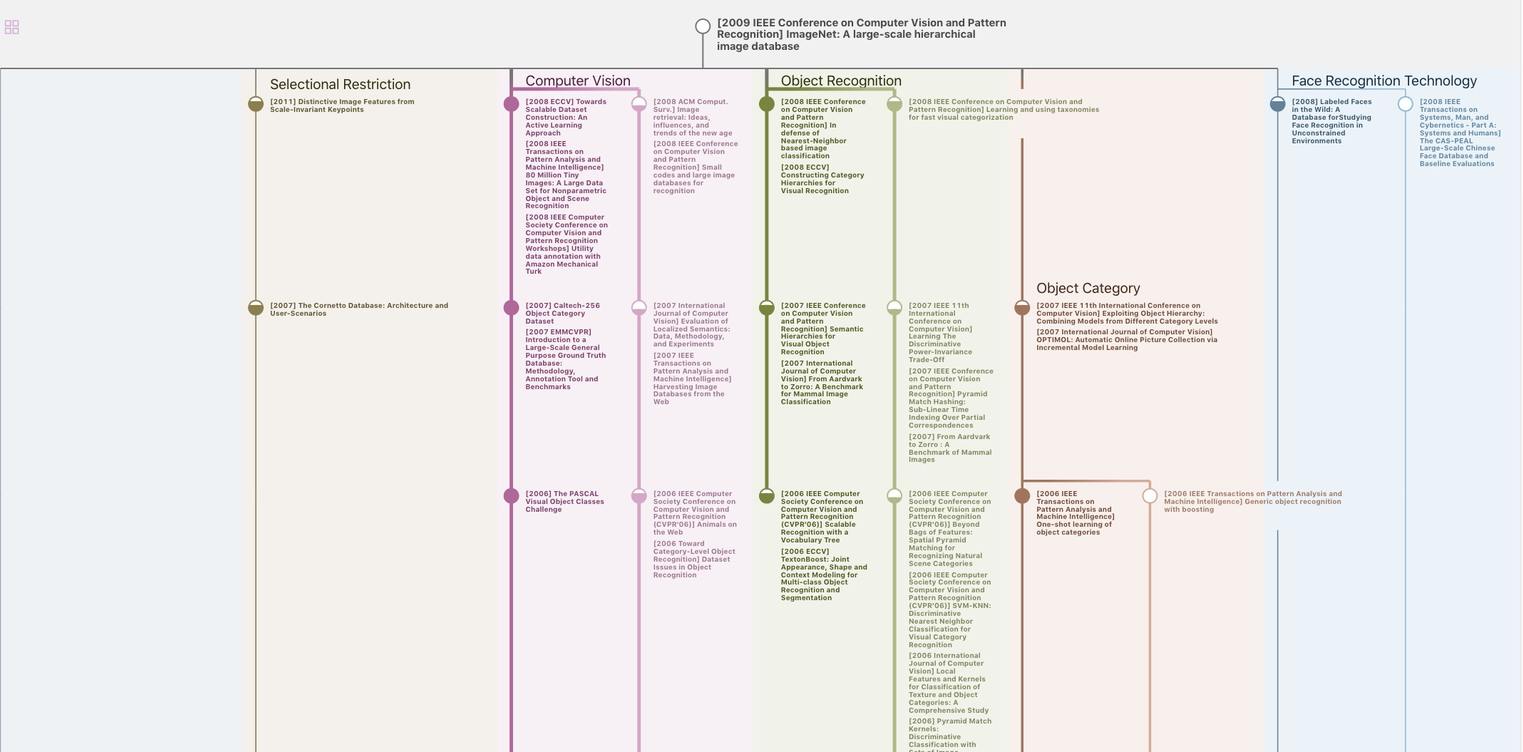
生成溯源树,研究论文发展脉络
Chat Paper
正在生成论文摘要