A Flash Attention Transformer for Multi-Behaviour Recommendation
PROCEEDINGS OF THE 32ND ACM INTERNATIONAL CONFERENCE ON INFORMATION AND KNOWLEDGE MANAGEMENT, CIKM 2023(2023)
摘要
Recently, modelling heterogeneous interactions in recommender systems has attracted research interest. Real-world scenarios involve sequential multi-type user-item interactions such as "view", "add-to-favourites", "add-to-cart" and "purchase". Graph Neural Network (GNN) methods have been widely adopted in Representation Learning of similar sequential user-item interactions. Promising results have been achieved by the integration of GNNs and transformers for self-attention. However, GNN based methods suffer from limited capability in handling global user-item interaction dependencies, particularly for long sequences. Moreover, these models require high computational cost of transformers, due to the quadratic memory and time complexity with respect to sequence length. This results in memory bottlenecks and slow training especially in computational resource-constrained environments. To address these challenges, we propose the FATH model which employs Flash Attention mechanism to reduce the high-bandwidth memory usage over higher-order user-item interaction sequences. Experimental results show that our model improves the training speed and reduces the memory usage with better recommendation performance in comparison with the state-of the art baselines.
更多查看译文
关键词
Flash Attention,Transformer,Multi-behaviour Recommendation,Graph Neural Networks
AI 理解论文
溯源树
样例
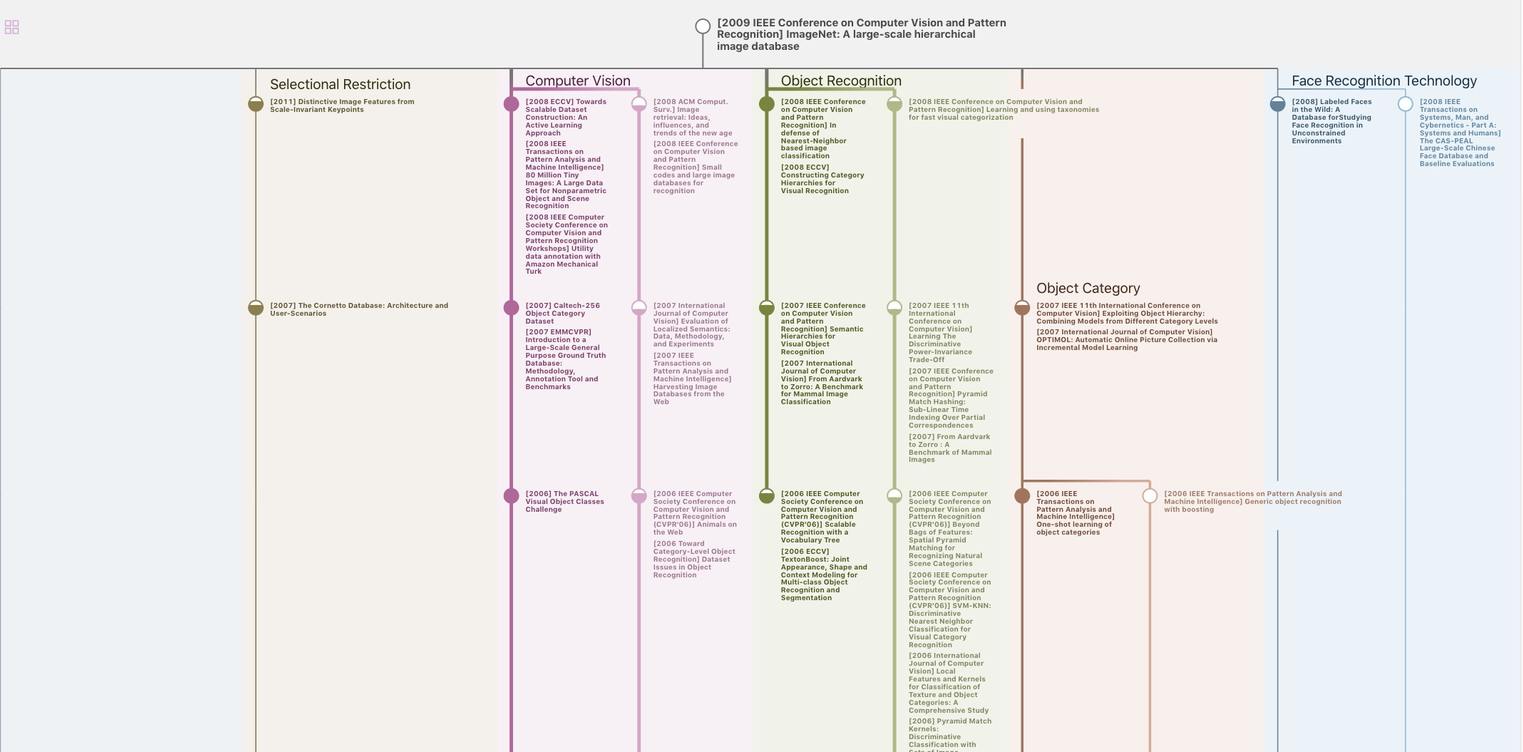
生成溯源树,研究论文发展脉络
Chat Paper
正在生成论文摘要