Meta-Transfer-Learning for Time Series Data with Extreme Events: An Application to Water Temperature Prediction
PROCEEDINGS OF THE 32ND ACM INTERNATIONAL CONFERENCE ON INFORMATION AND KNOWLEDGE MANAGEMENT, CIKM 2023(2023)
摘要
This paper proposes a meta-transfer-learning method for predicting daily maximum water temperature in stream networks with explicit modeling of extreme events. Accurate prediction of these extreme events is challenging because of their sparsity in the training data and their distinct responses to external drivers when compared to non-extreme observations. To overcome these challenges, we propose a sample reweighting strategy to escalate the importance of extreme events in the training process while preserving the predictive performance in normal time periods. The sample weight for each training data point is estimated as the similarity with the target test data point using contextual information and physical simulation. The obtained sample weight values are then used to fine-tune the initial model to transfer it to the test data. This method is further enhanced by an extreme value theory-based loss function to enforce the distribution of extreme data points and accelerated by a clustering algorithm based on the estimated similarities. Additionally, we introduce an online learning strategy to further refine the predictive model using newly collected observed data. The experimental results using real stream data from the Delaware River Basin over the past 36 years demonstrate that our meta-transfer-learning method produces more accurate predictions in both normal and extreme time periods when compared to baselines without the sample re-weighting scheme. The similarity learning method can reveal meaningful relationships amongst data points. We also show that the clustering algorithm can be used to accelerate the prediction while not compromising the predictive performance. The online learning strategy is shown to further improve predictive performance using recently observed data.
更多查看译文
关键词
Meta-transfer-learning,extreme event,stream temperature
AI 理解论文
溯源树
样例
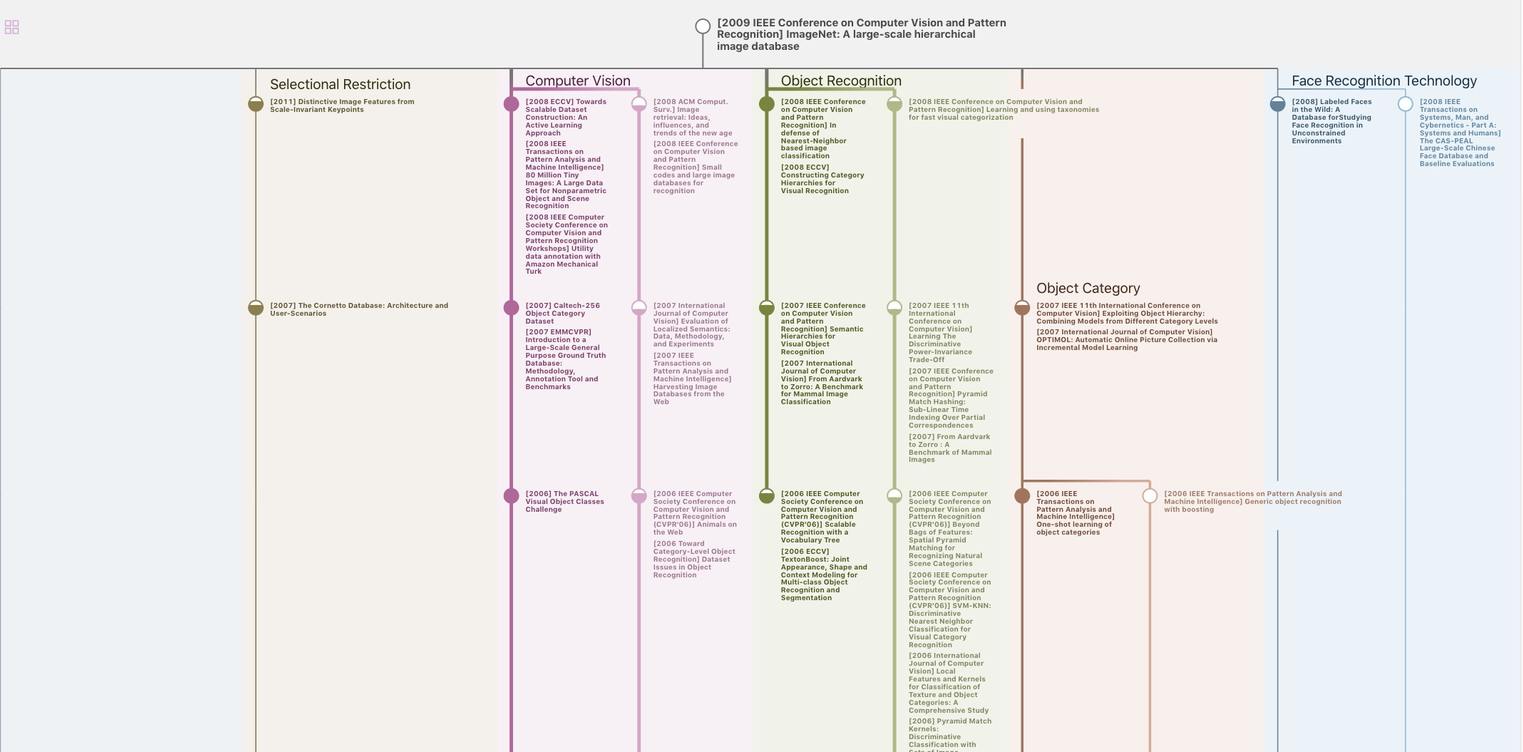
生成溯源树,研究论文发展脉络
Chat Paper
正在生成论文摘要