Spatial-Temporal Graph Boosting Networks: Enhancing Spatial-Temporal Graph Neural Networks via Gradient Boosting
PROCEEDINGS OF THE 32ND ACM INTERNATIONAL CONFERENCE ON INFORMATION AND KNOWLEDGE MANAGEMENT, CIKM 2023(2023)
摘要
Spatial-temporal graph neural networks (STGNNs) are promising in solving real-world spatial-temporal forecasting problems. Recognizing the inherent sequential relationship of spatial-temporal data, it is natural to explore the integration of boosting training mechanism to further enhance the performance of STGNNs. However, few studies have touched this research area. To bridge this gap, in this work, we propose spatial-temporal graph boosting networks, namely STGBN, which to the best of our knowledge is the first attempt to leverage gradient boosting for enhancing STGNNs. STGBN follows the general training procedure of conventional gradient boosting, but incorporates two distinctive designs to improve its efficiency in training on spatial-temporal graphs. Specifically, we design an incremental learning strategy that progressively includes spatial-temporal data into training. Additionally, we enforce an identical architecture for the base learner in all boosting iterations with each base learner inheriting from the one in the previous iteration. These designs facilitate rapid convergence of the base learner and expedite the overall training process. The base learner in STGBN is designed as a Transformer sandwich, which consists of two temporal Transformers on the top and bottom and a spatial Transformer in the middle. Structuring them in such a way helps the model capture long-range temporal dynamics, global spatial dependencies, and deep spatial-temporal interactions. We perform extensive spatial-temporal forecasting experiments on four spatial-temporal graph benchmarks. Promising results demonstrate the outstanding performance of STGBN against a wide range of state-of-the-art baseline models.
更多查看译文
关键词
spatial-temporal graphs,graph neural networks,gradient boosting
AI 理解论文
溯源树
样例
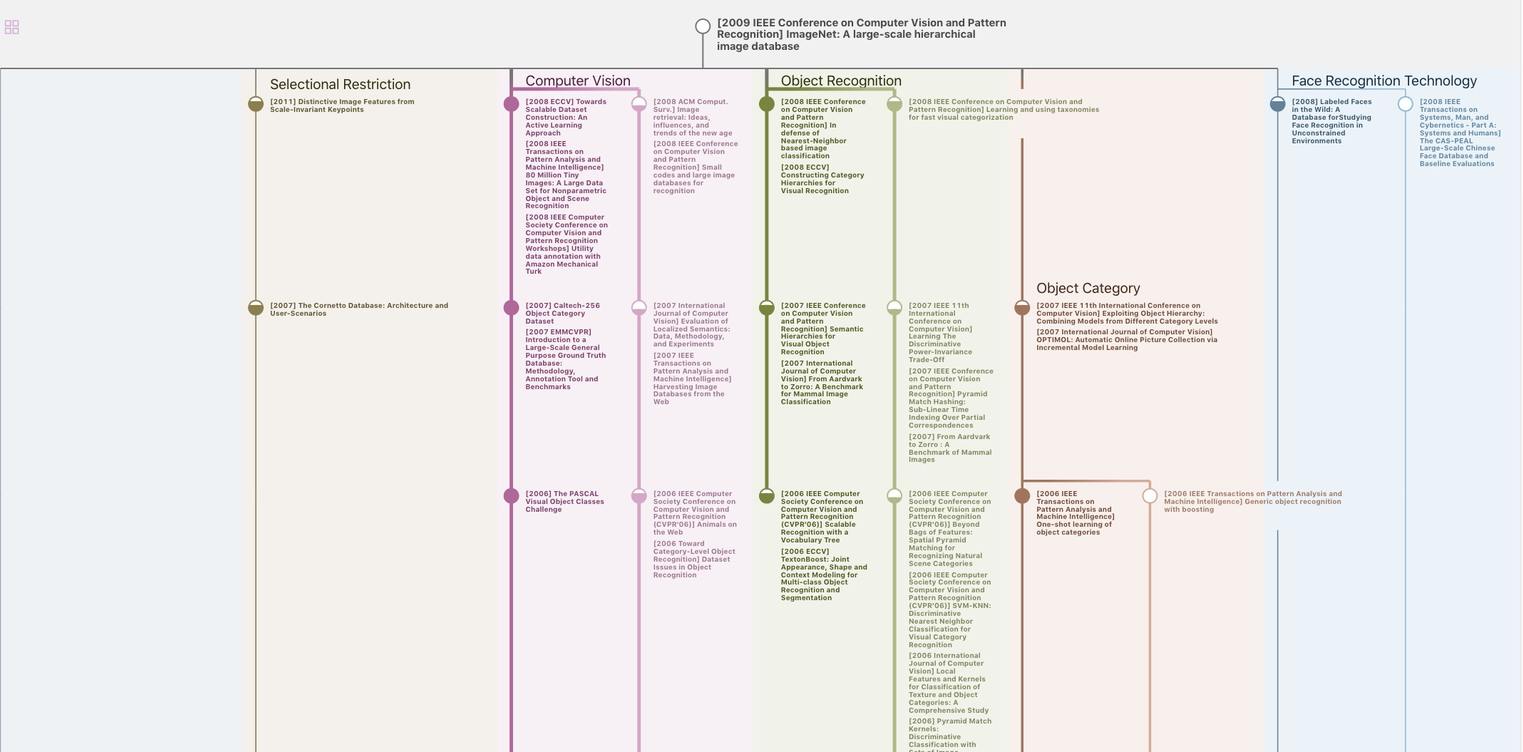
生成溯源树,研究论文发展脉络
Chat Paper
正在生成论文摘要