Integrating Priors into Domain Adaptation Based on Evidence Theory
PROCEEDINGS OF THE 32ND ACM INTERNATIONAL CONFERENCE ON INFORMATION AND KNOWLEDGE MANAGEMENT, CIKM 2023(2023)
摘要
Domain adaptation aims to build up a learning model for target domain by leveraging transferable knowledge from different but related source domains. Existing domain adaptation methods generally transfer the knowledge from source domain to target domain through measuring the consistency between the different domains. Under this strategy, if the data of source domain is not sufficient to guarantee the consistency, the transferable knowledge will be very limited. On the other hand, we often have priors about target domain which facilitate knowledge transfer but are neglected in the extant domain adaptation methods. To tackle the problems, we integrate the priors of target domain into transfer process and propose a domain adaptation method based on evidence evidence theory. We represent the priors with evidential belief function and reformulate the domain adaptation objective based on likelihood principle, in which the priors are used to adjust transferred knowledge to suit for target domain. Based on this, we propose an improved coordinate ascent algorithm to optimize likelihood objective of domain adaption. Experimental results on both text and image datasets validate that the proposed method is effective to improve the knowledge transferability in domain adaptation, especially when the source domain is limited.
更多查看译文
关键词
Domain Adaptation,Transfer Learning,Evidence Theory,Prior Knowledge
AI 理解论文
溯源树
样例
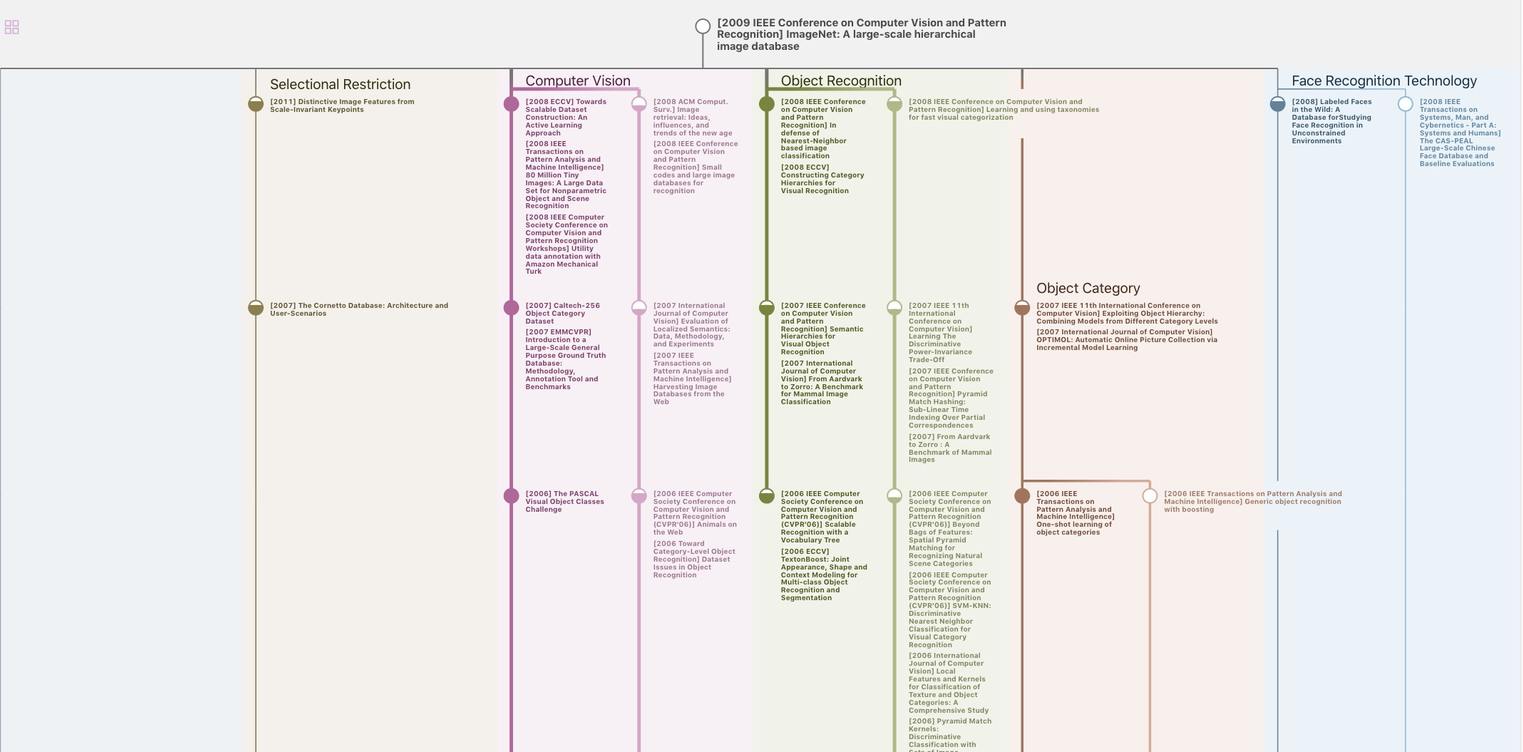
生成溯源树,研究论文发展脉络
Chat Paper
正在生成论文摘要