ManiCast: Collaborative Manipulation with Cost-Aware Human Forecasting
CoRR(2023)
摘要
Seamless human-robot manipulation in close proximity relies on accurate forecasts of human motion. While there has been significant progress in learning forecast models at scale, when applied to manipulation tasks, these models accrue high errors at critical transition points leading to degradation in downstream planning performance. Our key insight is that instead of predicting the most likely human motion, it is sufficient to produce forecasts that capture how future human motion would affect the cost of a robot's plan. We present ManiCast, a novel framework that learns cost-aware human forecasts and feeds them to a model predictive control planner to execute collaborative manipulation tasks. Our framework enables fluid, real-time interactions between a human and a 7-DoF robot arm across a number of real-world tasks such as reactive stirring, object handovers, and collaborative table setting. We evaluate both the motion forecasts and the end-to-end forecaster-planner system against a range of learned and heuristic baselines while additionally contributing new datasets. We release our code and datasets at https://portal-cornell.github.io/manicast/.
更多查看译文
关键词
collaborative manipulation,forecasting,cost-aware
AI 理解论文
溯源树
样例
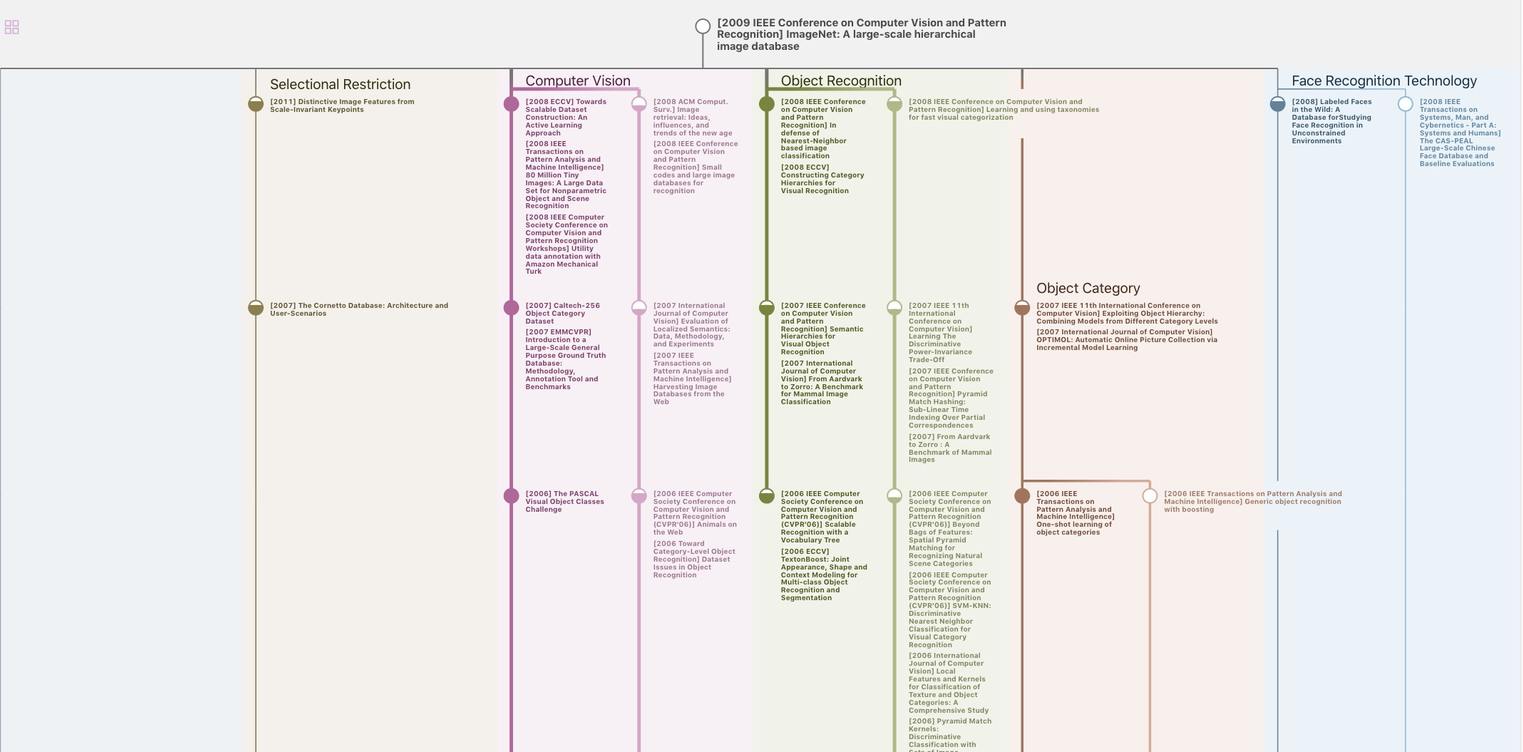
生成溯源树,研究论文发展脉络
Chat Paper
正在生成论文摘要