DRIVE: Dynamic Resource Introspection and VNF Embedding for 5G Using Machine Learning
IEEE INTERNET OF THINGS JOURNAL(2023)
摘要
The network slicing (NS) technique is comprehensively reshaping the next-generation communication networks (e.g., 5G and 6G). Software-defined networking (SDN) and network functions virtualization (NFV) predominantly control the flow of service functions on NS to incorporate versatile applications as per user demands. In the virtualized-SDN (vSDN) environment, a chain of well-defined virtual network functions (VNFs) are installed on service function chains (SFCs) by multiple Internet service providers (ISPs) concurrently. Generation, allocation, reallocation, release, and destroying associative VNFs on SFC is an extremely difficult task while keeping high selection accuracy. Toward solving this fundamental issue, in this work, we have proposed a multilayered SFC formation for adaptive VNF allocation on dynamic slices. We have formulated an ILP to address the VNF-embedding and allocation problem (VNF-EAP) over real network topology (AT&T Topology). Leveraging machine learning techniques we have shown an intelligent VNF selection mechanism to optimize resource utilization. The performance evaluation shows remarkable efficiency on ML-driven dynamic VNF selections over static allocations on SFCs by halving resource usage. Further, we have also studied a VNF typecasting technique for service backup on outage slices in the field of disaster management activities.
更多查看译文
关键词
5G and beyond,machine learning,network functions virtualization (NFV),service function chain (SFC),software-defined networking (SDN),TSPs,virtual network function (VNF)
AI 理解论文
溯源树
样例
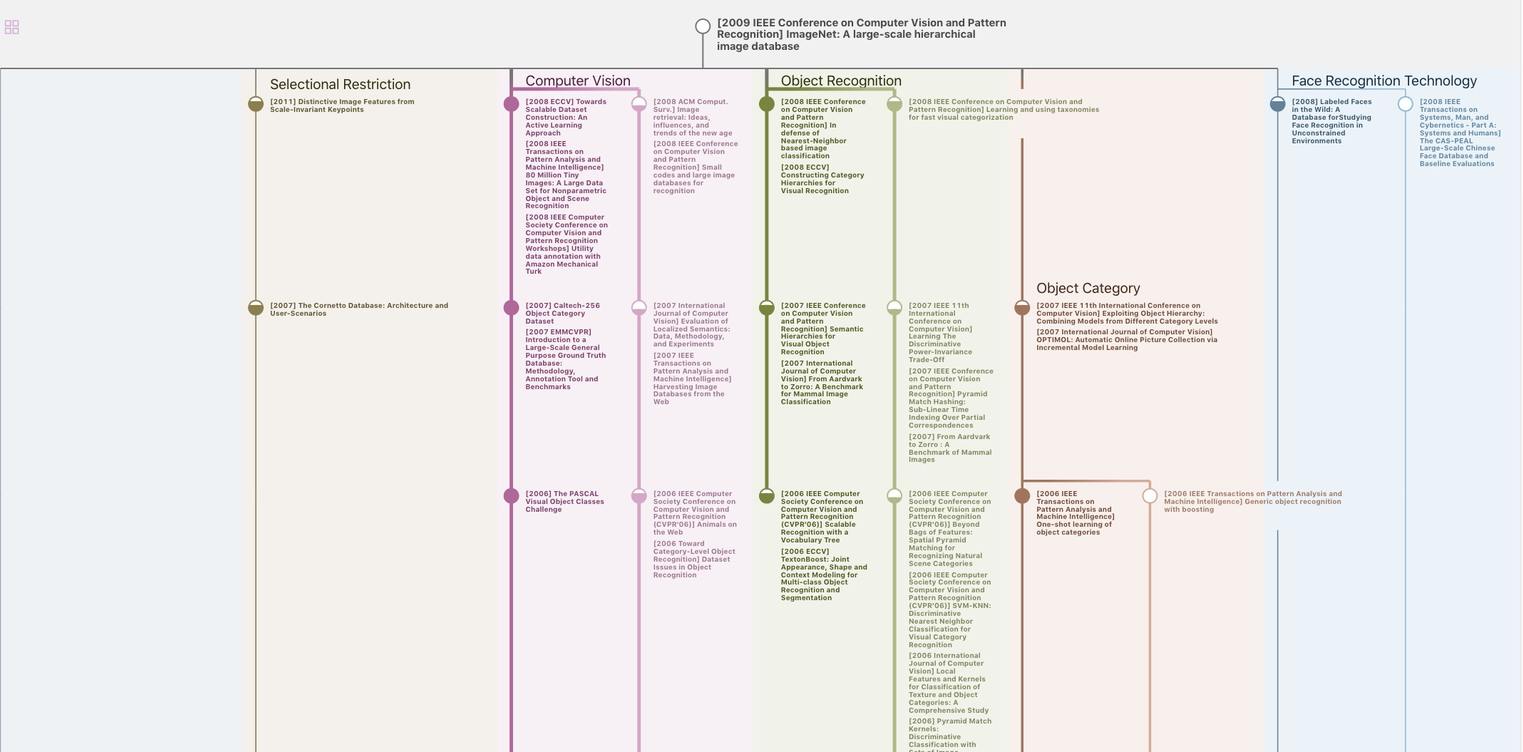
生成溯源树,研究论文发展脉络
Chat Paper
正在生成论文摘要