A robust change detection methodology for flood events using sar images
IGARSS 2023 - 2023 IEEE INTERNATIONAL GEOSCIENCE AND REMOTE SENSING SYMPOSIUM(2023)
摘要
Accurate flood mapping plays a critical role in disaster management, allowing for effective response and mitigation efforts. Thus, researchers seek to boost the accuracy of flood mapping algorithms, especially in terms of generalization capability and minimizing False Positive and False Negative detection. This paper presents a robust flood mapping algorithm from SAR images via Deep Convolutional Neural Network (DCNN) that follows encoder-decoder scheme. By introducing Bidirectional Convolutional LSTM (ConvLSTM) layers into its architecture, the proposed Temporal-Spatial Encoder-Decoder Network (TSEDN) network is able to extract temporal information and produce more accurate change maps. The training and testing are carried using OMBRIA dataset, which is known to be challenging to train. The proposed network is evaluated and compared to other state-of-the-art approaches in terms of Overall Accuracy (OA), Precision, Recall, and mean Intersection over Union (mIoU).
更多查看译文
关键词
SAR,change detection,flood mapping,encoder-decoder,Bidirectional LSTM
AI 理解论文
溯源树
样例
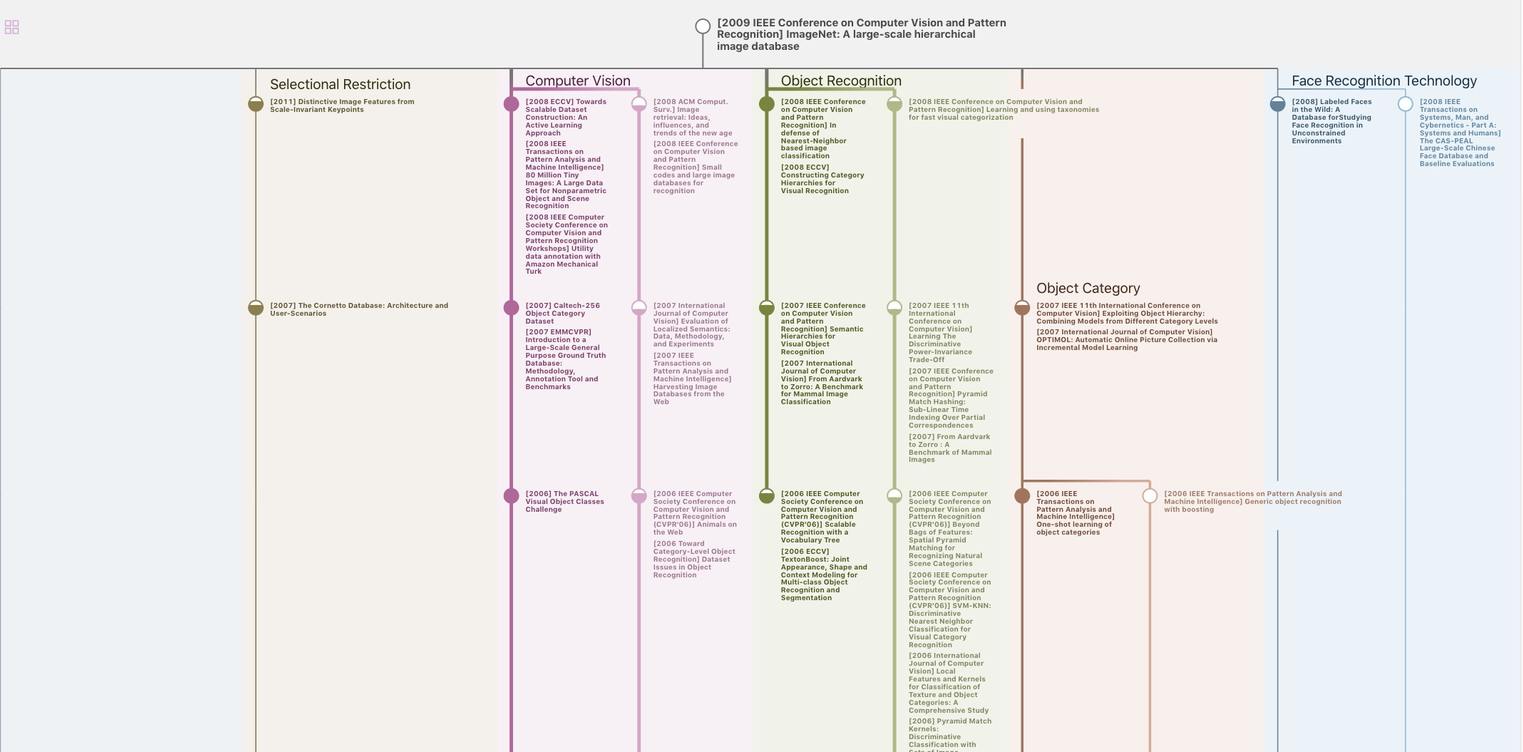
生成溯源树,研究论文发展脉络
Chat Paper
正在生成论文摘要