Application of sentinel-1 and dem data to shoreline detection based on u-net method
IGARSS 2023 - 2023 IEEE INTERNATIONAL GEOSCIENCE AND REMOTE SENSING SYMPOSIUM(2023)
摘要
With the characteristics of high resolution, strong penetration ability, all-day observation and wide spatial coverage, Synthetic Aperture Radar (SAR) image has been widely used in shoreline detection. However, the shoreline detection will be affected by the shadows of geometric distortion caused by the side-looking of SAR especially in areas with large terrain fluctuations, such as the eastern coast of Taiwan. Therefore, this study proposed an efficient shoreline detection method using dual-polarization Sentinel-1 SAR and digital elevation model (DEM) data based on deep learning methods. In this research, two common self-built datasets were introduced, which covered all coastal areas of the study area in Taiwan island. The datasets included a total of 4,029 and 3,522 images, respectively. One contains VH polarization images and the other consists of a three-layer stack with VH, VV polarization images and DEM data. The training images of the dataset were labeled by manual inspection and morphological processing. In this study, the shoreline detection was based on the semantic segmentation U-Net model with batch normalization (BN) module. The segmentation results of the U-Net model were then processed by morphological postprocessing and edge detection for shoreline detection. Experimental results show that the combination of dual-polarization SAR and DEM data significantly improves shoreline detection results compared to those using Sentinel-1 VH imagery.
更多查看译文
关键词
shoreline detection,Synthetic Aperture Radar,semantic segmentation,U-Net
AI 理解论文
溯源树
样例
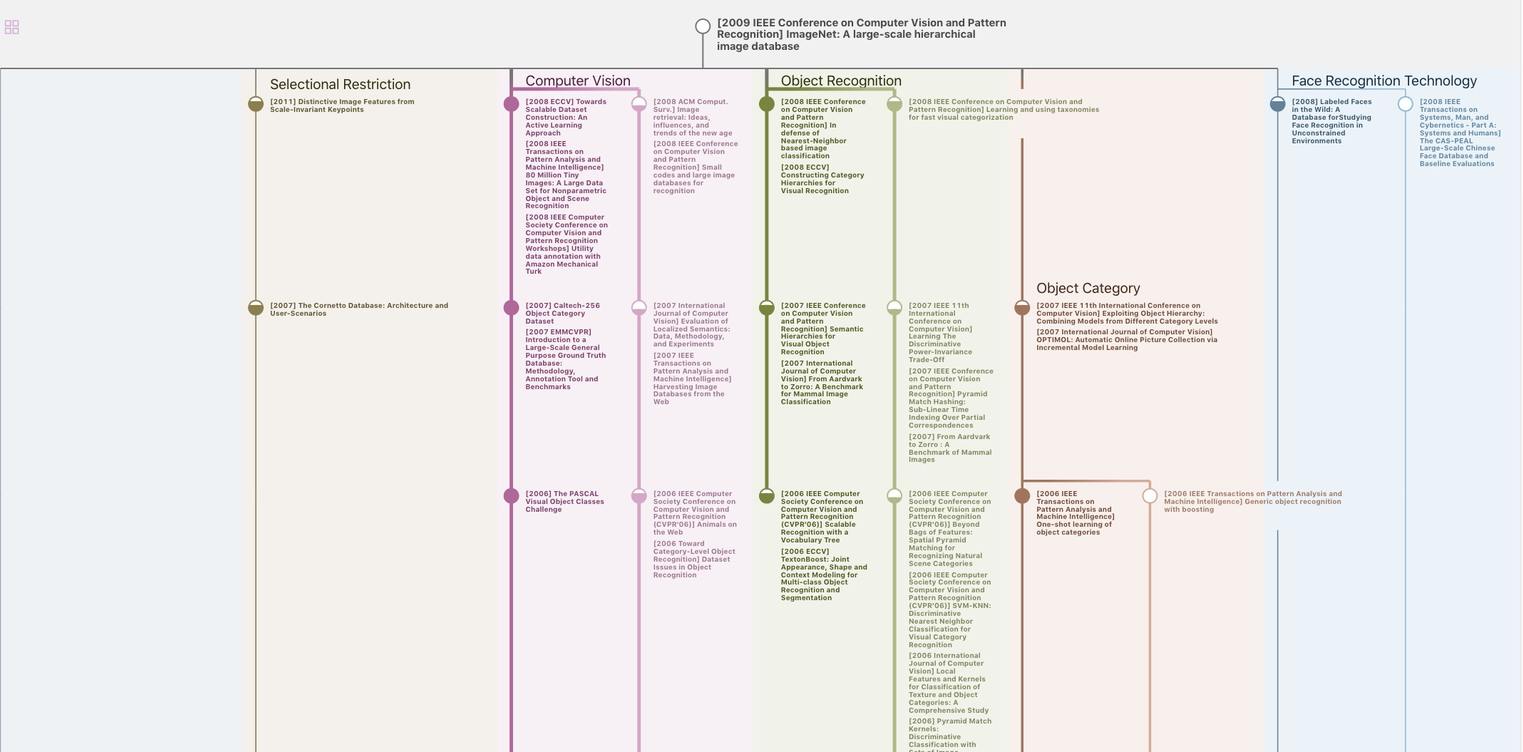
生成溯源树,研究论文发展脉络
Chat Paper
正在生成论文摘要