Observing supraglacial lakes using deep learning and planetscope imagery
IGARSS 2023 - 2023 IEEE INTERNATIONAL GEOSCIENCE AND REMOTE SENSING SYMPOSIUM(2023)
摘要
Supraglacial lakes (SGL)s result from melt water accumulation in topographic depressions on the surface of glaciers. SGLs primarily affect glacial dynamics through a positive feedback loop in which the albedo-lowering effect of SGLs can escalate surface melt leading to increases in lake extent and depth, amplifying the aforementioned albedo-lowering effect. The implications of accelerated glacial melt include increased sea level rise and modifications to ocean primary productivity. SGLs are critical indicators of surface melt and its downstream impacts and should be monitored efficiently. In situ observations and measurements of SGLs are time consuming, cost-prohibitive and difficult to scale. Earth observation data and machine learning enable scalable monitoring of SGLs through pattern detection and quantification of lake evolution over time [1]. This work presents a model developed by training a convolutional neural network with imagery and labels from NASA Operation IceBridge and predicting SGLs in high temporal and spatial resolution PlanetScope imagery.
更多查看译文
关键词
Supraglacial lake,cryosphere,small satellites,remote sensing,deep learning,segmentation
AI 理解论文
溯源树
样例
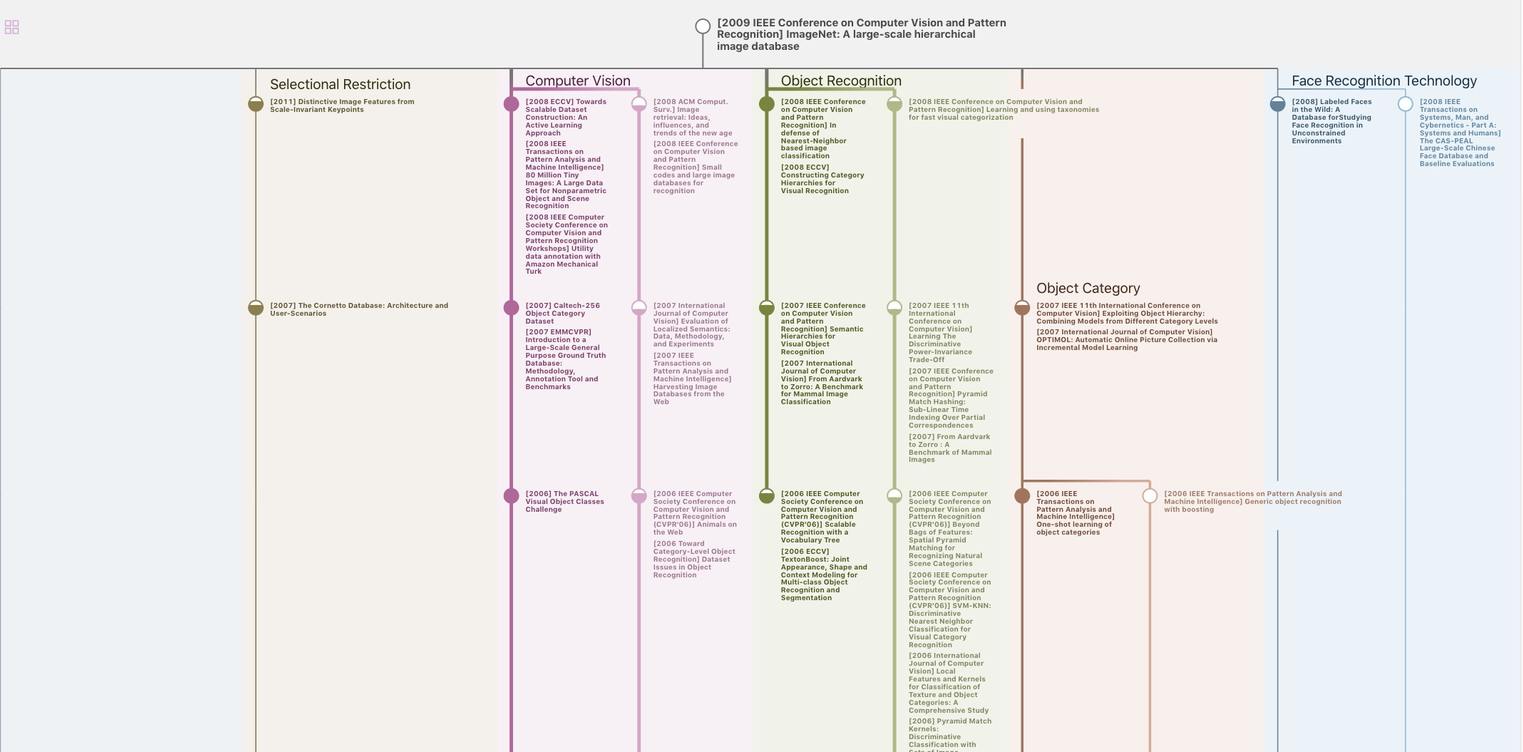
生成溯源树,研究论文发展脉络
Chat Paper
正在生成论文摘要