A feature disentanglement and unsupervised domain adaptation of remaining useful life prediction for sensor-equipped machines
RELIABILITY ENGINEERING & SYSTEM SAFETY(2024)
摘要
Accurately predicting a sensor-equipped machine's remaining useful life (RUL) is vital for prognostics and health management (PHM). Existing methods often assume that the model trained with training data can be directly transformed into new machines. However, each machine operates under unique conditions, making it challenging to use a model trained for one condition to predict RUL for other conditions. Moreover, machines often have large amounts of unlabeled data, and fully labeling the data can be costly. In this paper, we propose an unsupervised domain adaptation model for predicting the machine's RUL, i.e., feature disentanglement and multiscale residual temporal convolutional networks with attention mechanism and dense connection strategy. The model consists of five modules: a shared feature extractor, a domain adaptation module, a degradation correction module, a health state assessment, and an RUL predictor. In the model, a shared feature extractor divides the private domain features from the extracted features and extracts the domain-invariant shared features. The degradation correction module regulates the prediction results. The HS assessment evaluates the machine's health status. Moreover, a new activation function is introduced in the RUL predictor. Finally, the proposed model is verified on two public datasets and compared to state-of-the-art prediction models.
更多查看译文
关键词
Remaining useful life,Prognostics and health management,Domain adaptation,Feature disentanglement
AI 理解论文
溯源树
样例
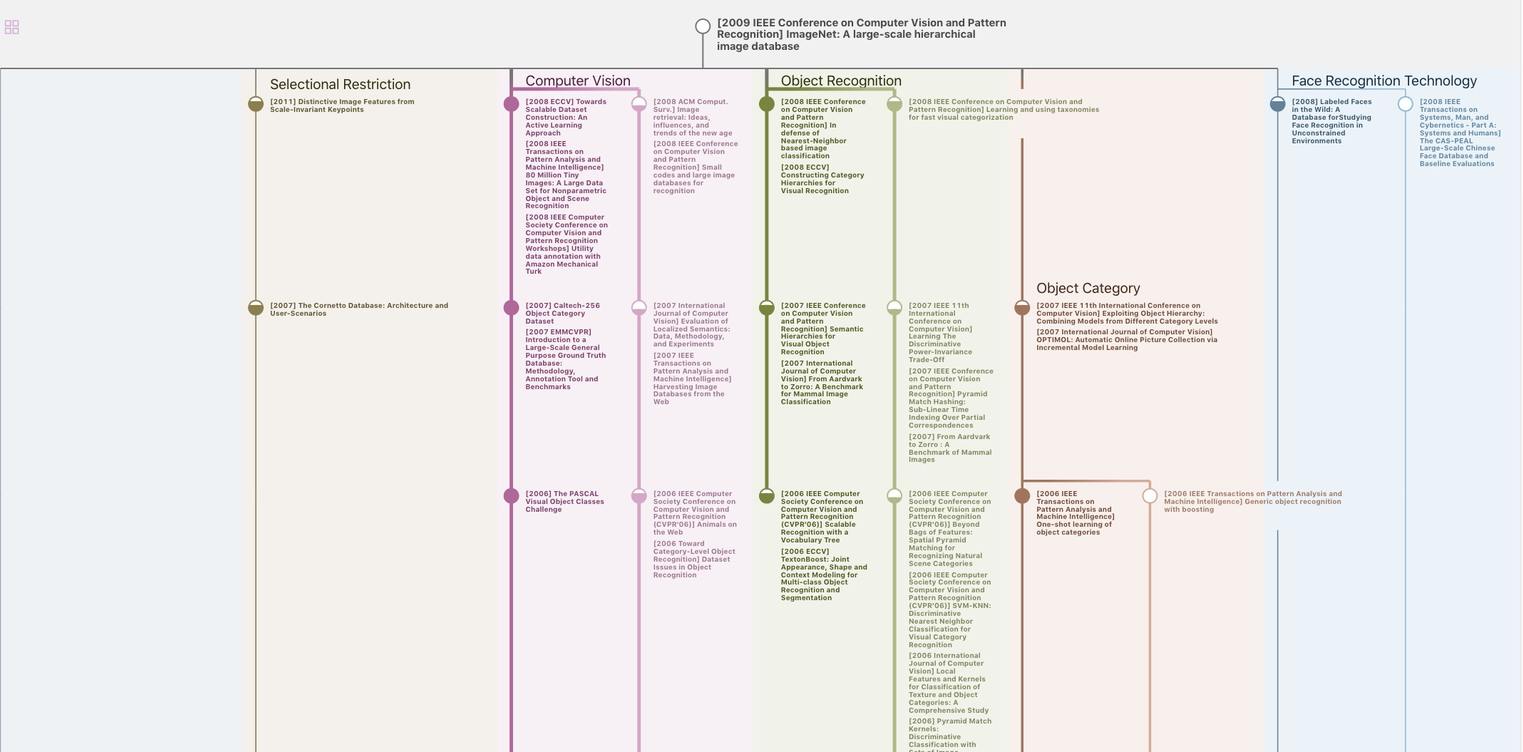
生成溯源树,研究论文发展脉络
Chat Paper
正在生成论文摘要