Multi-step and multi-task learning to predict quality-related variables in wastewater treatment processes
Process Safety and Environmental Protection(2023)
摘要
In wastewater treatment processes, lack of hardware sensors together with unacceptable dynamics, strong nonlinearity and large time delay often leads to a large number of key variables that are difficult to measure online accurately and timely, then frustrate safe operations of the processes. To accurately and timely capture the short-term behavior changes and trend development of critical variables, a novel neural network based soft-sensing model is proposed to take full use of multi-task learning, direct multi-step prediction strategy and evolutionary algorithm to formulate a novel multi-task multi-step evolution (MTMSE) neural network. Firstly, single-output MTMSE (SO-MTMSE) neural network is used to realize the dynamic monitoring of a single variable. Moreover, by considering the spatiotemporal interaction among the data, the model is extended to multi-output MTMSE (MO-MTMSE) neural network to simultaneously realize multi-step prediction of multiple variables, thus providing a desired reference for optimizing the wastewater treatment processes. Finally, the proposed model is applied to the benchmark simulation model 2 (BSM2) and a full-scale wastewater treatment plant in Shenzhen. And the results show that the proposed dynamic soft sensor model outperforms the standard methods, such as autoregressive moving average model (ARMA) and multiple output gaussian process regression (MGPR).
更多查看译文
关键词
wastewater treatment processes,learning,predict,multi-step,multi-task,quality-related
AI 理解论文
溯源树
样例
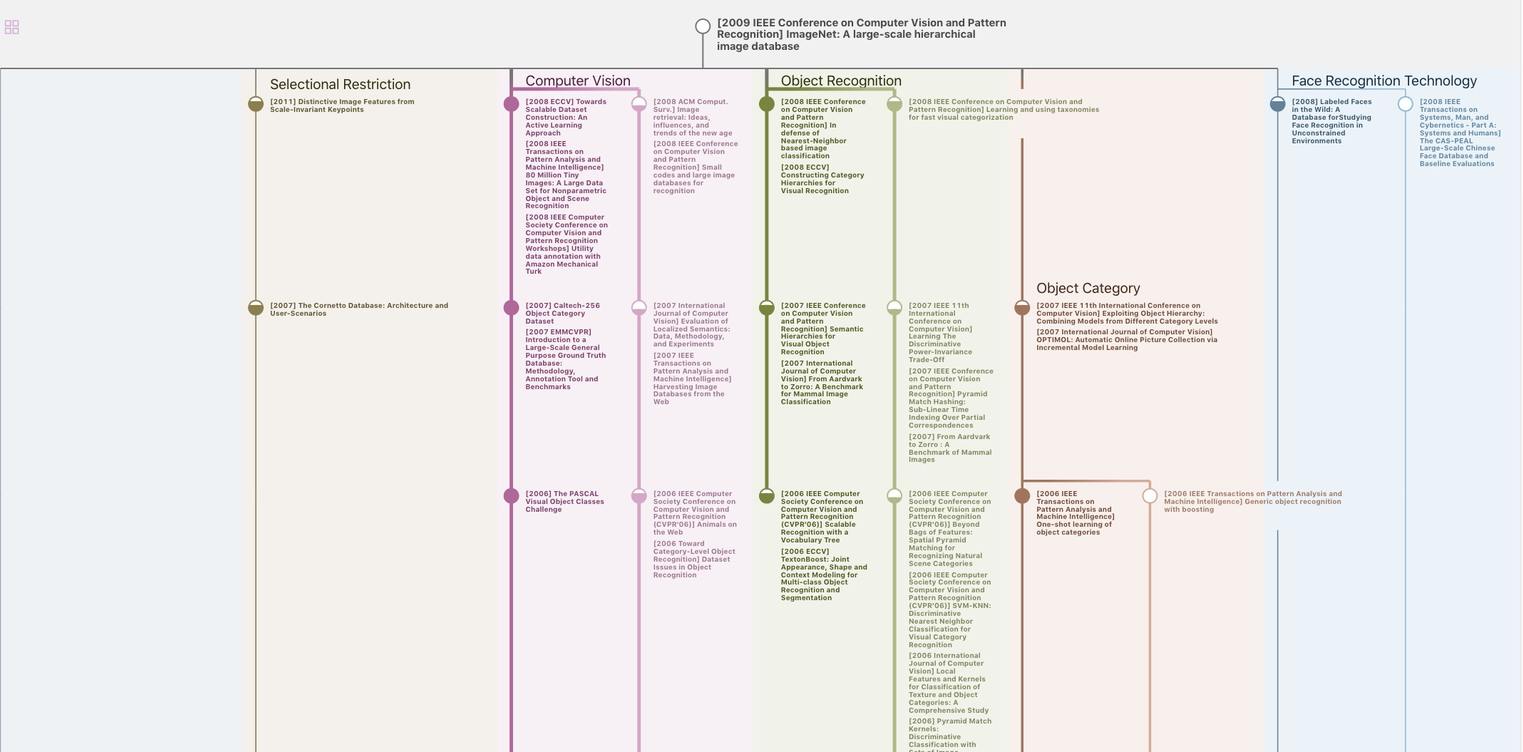
生成溯源树,研究论文发展脉络
Chat Paper
正在生成论文摘要