DEMRL: Dynamic estimation meta reinforcement learning for path following on unseen unmanned surface vehicle
Ocean Engineering(2023)
摘要
Reinforcement learning has been widely used for unmanned surface vehicle (USV) control tasks. However, the requirement of numerous training samples limits its transferability to new USVs. In this article, we propose a dynamic estimation meta reinforcement learning (DEMRL) approach that enables few-shot learning for the path following control policy. We first present a dynamic estimation method to learn a latent dynamic context feature. The learned context contains the hidden information of USV dynamics with only a few estimation samples. We then propose a meta reinforcement learning based training framework to learn the generalizable path following control policy. After that, given the prior knowledge from dynamic context, the well-trained policy can easily adapt to the target USV during the rapid adaptation process. This proposed method represents the initial effort in tackling the few-shot learning challenge associated with training reinforcement learning based USV path-following policies. Extensive experiments demonstrate that the proposed method can achieve promising path following performance for unseen USV with very few training data and training volume.
更多查看译文
关键词
reinforcement learning,path
AI 理解论文
溯源树
样例
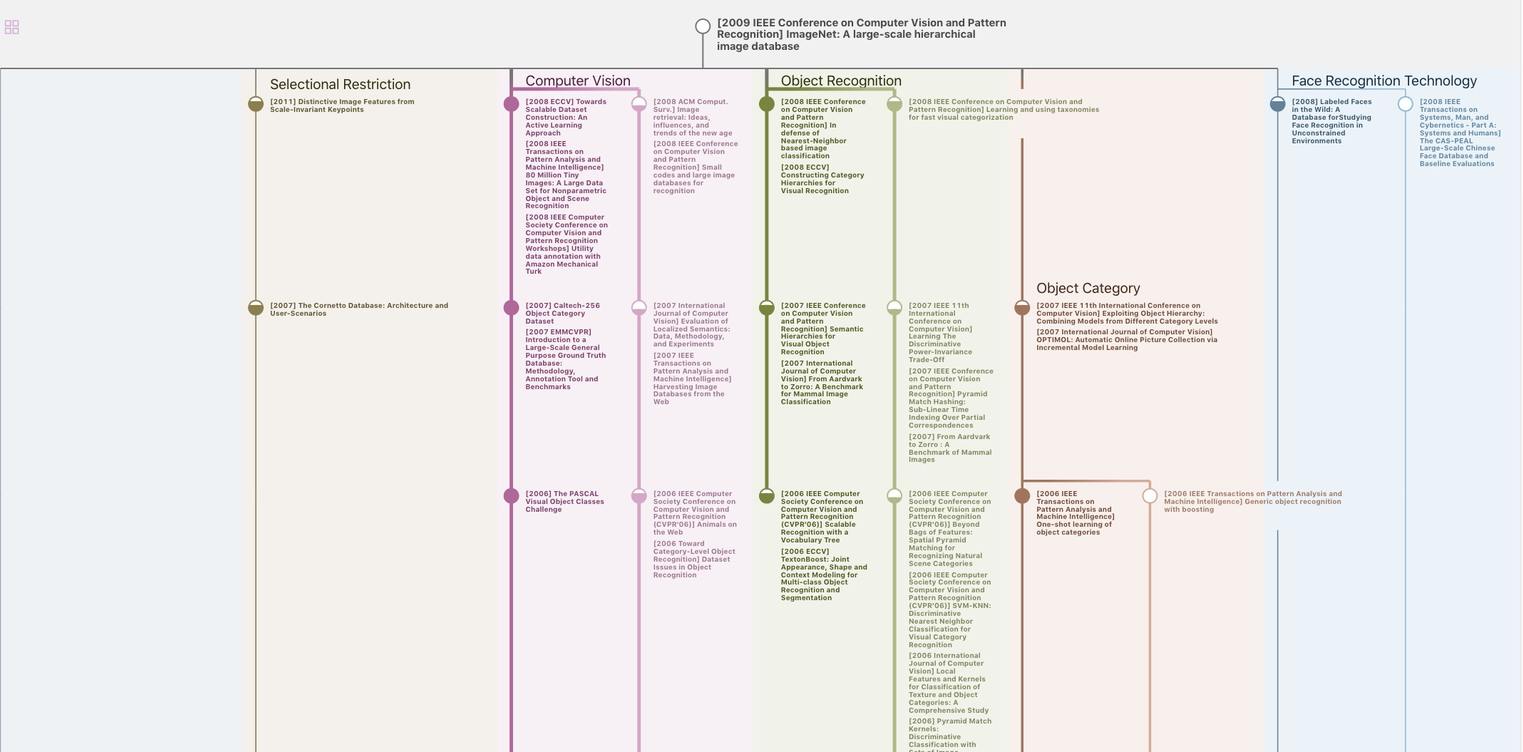
生成溯源树,研究论文发展脉络
Chat Paper
正在生成论文摘要