Adaptive-MAML: Few-shot metal surface defects diagnosis based on model-agnostic meta-learning
MEASUREMENT(2023)
摘要
The rapid development of artificial intelligence has further increased the level of intelligence in the field of metal surface defect diagnosis. In general, metal surface defects are difficult to collect. Considering the problem of insufficient sample size of defects, we propose a framework for metal surface defect diagnosis: Adaptive-MAML, which consists of an improved MAML framework and neural network model. Adaptive-MAML proposes a meta-augmentation method (MetaAug) to automatically generate virtual samples during the training process to alleviate the defect sample shortage problem and overcome the overfitting problem. It also uses a hyperparametric adaptive strategy based on gradient descent (HASGD) to improve the stability and scalability of the training process. Experimental results on the FSC-20 and NEU-CLS-64 datasets show that the system exhibits better results in surface defect classification compared to other state-of-the-art methods. In addition, we further validate the generalization of the framework by applying it to the synthetic DAGM.
更多查看译文
关键词
Few-shot learning,Model-agnostic meta-learning,Meta-augmentation,Hyperparameter adaptive,Metal surface defect diagnosis
AI 理解论文
溯源树
样例
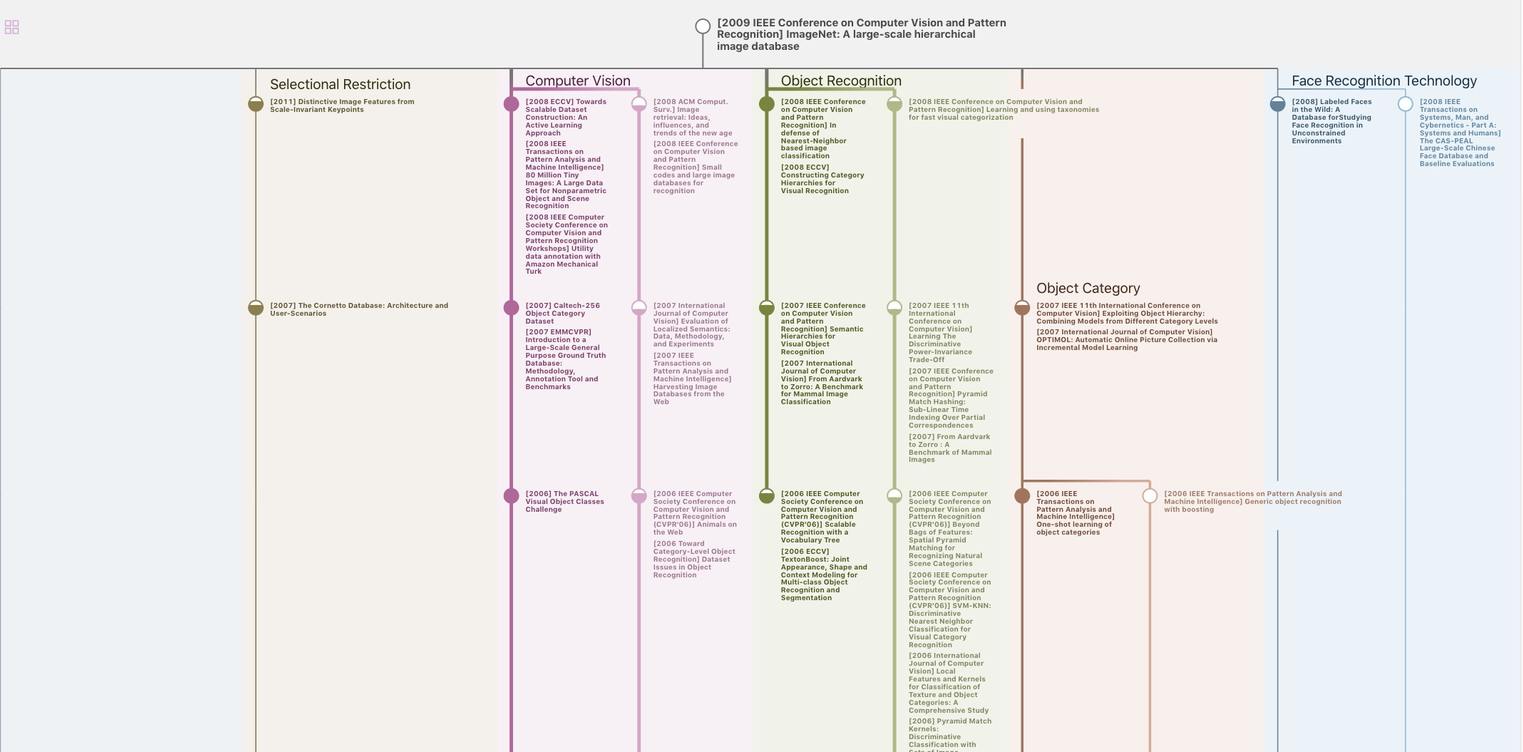
生成溯源树,研究论文发展脉络
Chat Paper
正在生成论文摘要