DDFusion: An efficient multi-exposure fusion network with dense pyramidal convolution and de-correlation fusion
Journal of Visual Communication and Image Representation(2023)
摘要
In this work, we propose DDFusion, a novel multi-exposure image fusion network. DDFusion addresses the limitations of existing methods by effectively recovering details near extremely bright regions and learning associations between non-contiguous regions. To achieve this, our network incorporates a dense pyramidal (DensePy) convolution block in the encoder for multi-scale feature extraction, and a de-correlation fusion (DF) block for enabling structurally coherent and edge-preserving multi-scale feature fusion. It facilitates a smoother transition from highlighted areas to adjacent regions in the fused image. Experimental results demonstrate the superiority of DDFusion over state-of-the-art deep methods in terms of both visual quality and quantitative evaluation. Moreover, DDFusion achieves stronger multi-scale feature extraction capability with smaller computational complexity.
更多查看译文
关键词
Multi-exposure fusion,Dense-pyramidal convolution,De-correlation convolution
AI 理解论文
溯源树
样例
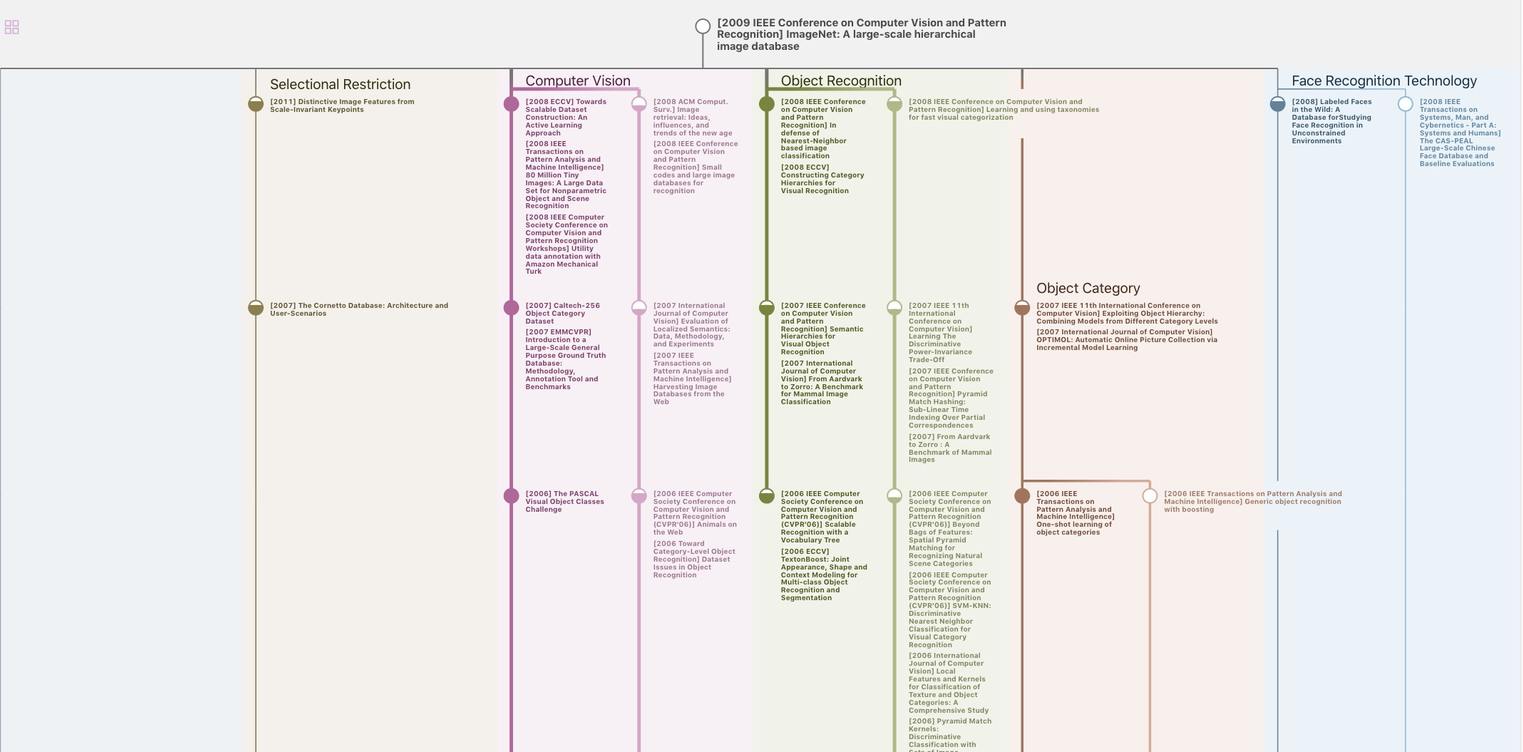
生成溯源树,研究论文发展脉络
Chat Paper
正在生成论文摘要