Model-based real-time prediction of surface roughness in fused deposition modeling with graph convolutional network-based error correction
Journal of Manufacturing Systems(2023)
摘要
Additively manufactured parts usually have relatively rough surface finish due to the layer-by-layer process. Extensive research has been conducted on the effect of process parameters on surface roughness as well as on real-time monitoring of surface roughness using sensor data. However, very few studies consider both process parameters and condition monitoring data to perform real-time prediction of surface roughness. To address this research gap, we introduce an augmented deep learning framework that integrates a model-based predictor with a deep learning-based error corrector to predict the surface roughness of parts fabricated via fused deposition modeling. The model-based predictor predicts surface roughness by considering the effects of process parameters on surface roughness, while the deep learning-based error corrector estimates the prediction errors between the true surface roughness and predicted surface roughness using real-time sensor data collected during additive manufacturing. To consider both correlations among features extracted from sensor signals and temporal correlations of each sensor signal, we construct multiple graphs to reveal these correlations and introduce a multi-graph convolutional network to analyze these undirected graphs. Experimental results show that the proposed framework outperforms existing data-driven methods reported in the literature.
更多查看译文
关键词
Additive manufacturing, Surface roughness, Graph convolutional network, Error correction, Fused deposition modeling
AI 理解论文
溯源树
样例
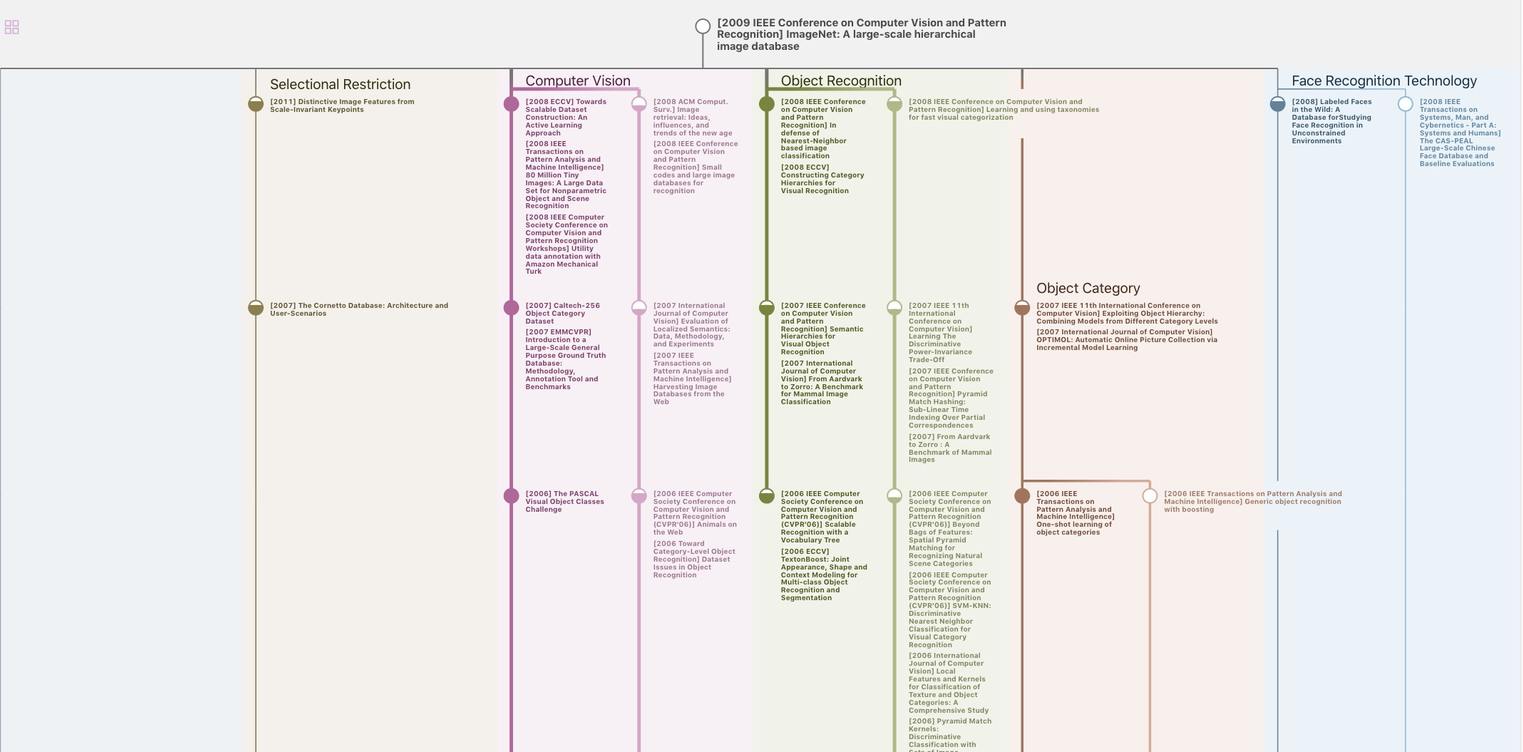
生成溯源树,研究论文发展脉络
Chat Paper
正在生成论文摘要