State of health and remaining useful life prediction of lithium-ion batteries with conditional graph convolutional network
EXPERT SYSTEMS WITH APPLICATIONS(2024)
摘要
Graph convolutional networks (GCNs) have been increasingly used to predict the state of health (SOH) and remaining useful life (RUL) of batteries. However, conventional GCNs have limitations. Firstly, the correlation between features and the SOH or RUL is not considered. Secondly, temporal relationships among features are not considered when projecting aggregated temporal features into another dimensional space. To address these issues, two types of undirected graphs are introduced to simultaneously consider the correlation among features and the correlation between features and the SOH or RUL. A conditional GCN is built to analyze these graphs. A dual spectral graph convolutional operation is introduced to analyze the topological structures of these graphs. Additionally, a dilated convolutional operation is integrated with the conditional GCN to consider the temporal correlation among the aggregated features. Two battery datasets are used to evaluate the effectiveness of the proposed method. Experimental results show that the proposed method outperforms other machine learning methods reported in the literature.
更多查看译文
关键词
Lithium-ion battery,State of health,Remaining useful life,Graph convolutional network,Temporal convolutional network
AI 理解论文
溯源树
样例
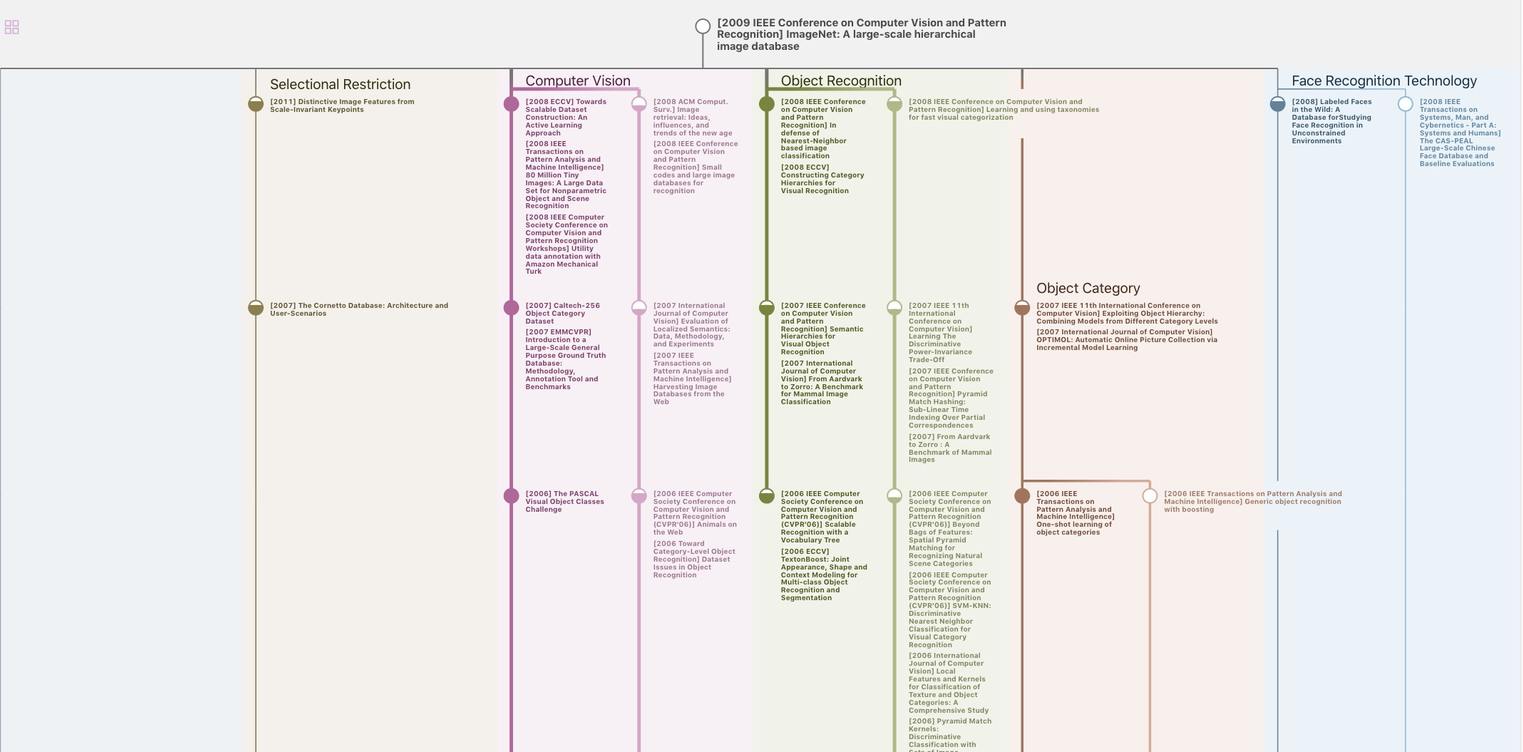
生成溯源树,研究论文发展脉络
Chat Paper
正在生成论文摘要