An efficient astronomical seeing forecasting method by random convolutional Kernel transformation
ENGINEERING APPLICATIONS OF ARTIFICIAL INTELLIGENCE(2024)
摘要
Astronomical seeing is the primary indicator of the observation quality of large-scale optical telescopes. Forecasting astronomical seeing is an essential and challenging task in the operation of large optical telescopes, as it can help observers more efficiently arrange observation tasks as well as providing hints for mechanism optimization of optical telescopes. In this paper, we propose an efficient data-driven astronomical seeing forecasting method by leveraging the environment monitoring data from multiple sensors deployed with optical telescopes. The basic idea is to use a large number of random yet fixed convolution kernels to extract features from the original data, and then train a simple tree-based predictor based on these features. In particular, the raw monitoring data is transformed by differential operations, enabling a more explicit representation of spatial and temporal features; then an elaborate convolution mechanism, including the convolution kernel and the pooling operator, is designed to extract a variety set of features of the input time series, which serve as the foundation for constructing the time series forecasting model. In contrast to existing deep learning models, there is no need to calculate and back-propagate the gradients during the training procedure, making the proposed method much more efficient. We collect real-world monitoring data from Large Sky Area Multi-Object Fiber Spectroscopy Telescope (LAMOST), one of the world's largest optical reflecting telescopes, and conduct extensive empirical evaluations. The experimental results demonstrate that the proposed method can achieve state-of-the-art prediction accuracy, i.e., 0.1125 in MAE, 0.0252 in MSE, and 0.0322 in MAPE, outperforming most deep-learning-based forecasting models. Moreover, it surpasses both machine learning and deep learning approaches in terms of training efficiency with a significant margin.
更多查看译文
关键词
Astronomical seeing,Seeing forecasting,Time series,Convolution kernel
AI 理解论文
溯源树
样例
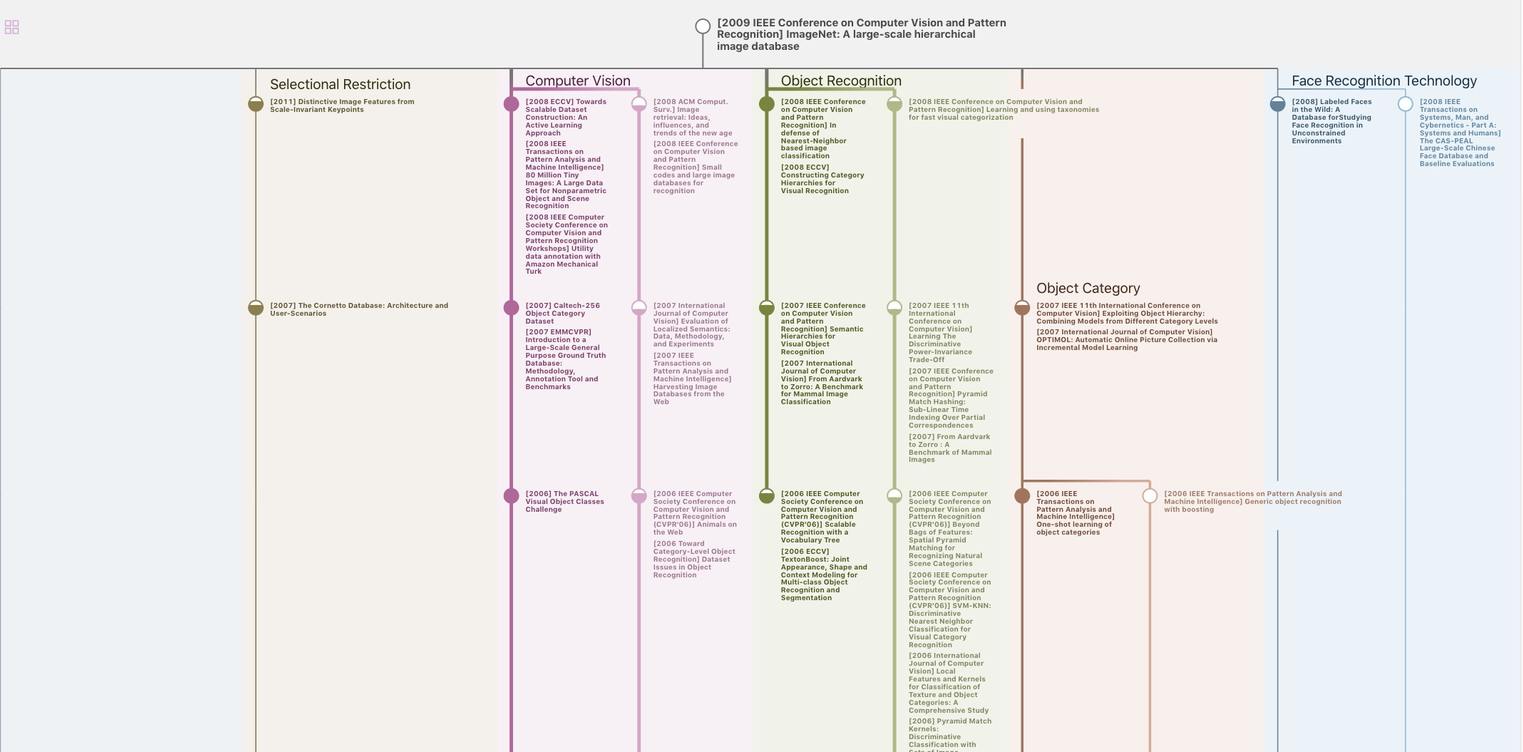
生成溯源树,研究论文发展脉络
Chat Paper
正在生成论文摘要