Co-evolution of neural architectures and features for stock market forecasting: A multi-objective decision perspective
Decision Support Systems(2023)
摘要
In a multi-objective setting, a portfolio manager's highly consequential decisions can benefit from assessing alternative forecasting models of stock index movement. The present investigation proposes a new approach to identify a set of non-dominated neural network models for further selection by the decision-maker. A new co -evolution approach is proposed to simultaneously select the features and topology of neural networks (collectively referred to as neural architecture), where the features are viewed from a topological perspective as input neurons. Further, the co-evolution is posed as a multi-criteria problem to evolve sparse and efficacious neural architectures. The well-known dominance and decomposition based multi-objective evolutionary algorithms are augmented with a non-geometric crossover operator to diversify and balance the search for neural architectures across conflicting criteria. Moreover, the co-evolution is augmented to accommodate the data-based implications of distinct market behaviors prior to and during the ongoing COVID-19 pandemic. A detailed comparative evaluation is carried out with the conventional sequential approach of feature selection followed by neural topology design, as well as a scalarized co-evolution approach. The results on three market indices (NASDAQ, NYSE, and S&P500) in pre-and peri-COVID time windows convincingly demonstrate that the proposed co-evolution approach can evolve a set of non-dominated neural forecasting models with better generalization capabilities.
更多查看译文
关键词
Feature selection,Financial forecasting,Neural architecture search,Multi-criteria decision making
AI 理解论文
溯源树
样例
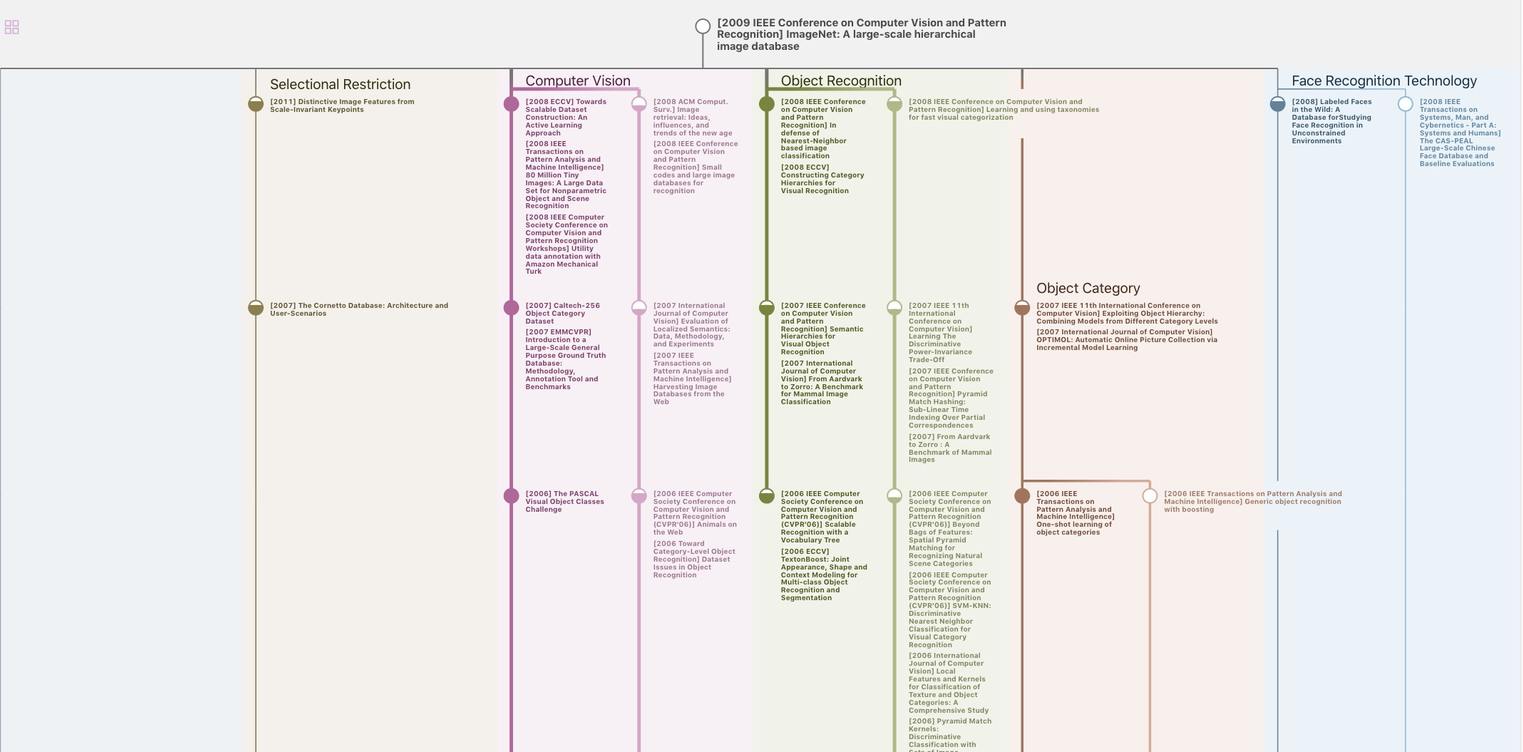
生成溯源树,研究论文发展脉络
Chat Paper
正在生成论文摘要