Deep Learning Based Segmentation for Pulmonary Embolism Detection in Real-World CT Angiography: Classification Performance
medRxiv (Cold Spring Harbor Laboratory)(2023)
摘要
Objectives To develop a deep learning-based algorithm that automatically and accurately classifies patients as either having pulmonary emboli or not in CT pulmonary angiography (CTPA) examinations with high accuracy.
Materials and Methods Seven hundred CTPA examinations from 652 patients (median age 72 years, range 16-100 years; interquartile range 18 years; 353 women) performed at a single institution between 2014 and 2018, of which 149 examinations contained PE, were used for model development. The nnU-Net deep learning-based segmentation framework was trained using 5-fold cross-validation. To enhance classification, we applied logical rules based on PE volume and probability thresholds. External model evaluation was performed in 770 and 34 CTPAs from two independent datasets.
Results A total of 1483 CTPA examinations were evaluated. In internal cross-validation and test set, the trained model correctly classified 123 of 128 examinations as positive for PE (sensitivity 96.1%; 95% C.I. 91-98%; P < .05) and 521 of 551 as negative (specificity 94.6%; 95% C.I. 92-96%; P < .05). In the first external test dataset, the trained model correctly classified 31 of 32 examinations as positive (sensitivity 96.9%; 95% C.I. 84-99%; P < .05) and 2 of 2 as negative (specificity 100%; 95% C.I. 34-100%; P < .05). In the second external test dataset, the trained model correctly classified 379 of 385 examinations as positive (sensitivity 98.4%; 95% C.I. 97-99%; P < .05) and 346 of 385 as negative (specificity 89.9%; 95% C.I. 86-93%; P < .05).
Conclusion Beyond state-of-art classification for PE in CTPA was achieved using nnU-Net for deep learning-based segmentation in combination with volume-and probability-based classification.
Clinical relevance statement A rapid and fully automated classification of patients as having or not having PE in CTPA examinations using deep learning techniques can help prioritize patients with PE for rapid review in emergency radiology.
Key Points
### Competing Interest Statement
The authors have declared no competing interest.
### Funding Statement
The project was supported by a grant from Analytic Imaging Diagnostic Arena (AIDA), https://medtech4health.se/aida-en/, to Tobias Sjöblom. Tomas Fröding and Dimitrios Toumpanakis were supported by clinical fellowships from AIDA. Tomas Fröding was supported by the Centre for Clinical Research Sörmland, Uppsala University, Eskilstuna, Sweden.
### Author Declarations
I confirm all relevant ethical guidelines have been followed, and any necessary IRB and/or ethics committee approvals have been obtained.
Yes
The details of the IRB/oversight body that provided approval or exemption for the research described are given below:
Swedish Ethical Review Authority (EPN Uppsala Dnr 2015/023 and 2015/023/1).
I confirm that all necessary patient/participant consent has been obtained and the appropriate institutional forms have been archived, and that any patient/participant/sample identifiers included were not known to anyone (e.g., hospital staff, patients or participants themselves) outside the research group so cannot be used to identify individuals.
Yes
I understand that all clinical trials and any other prospective interventional studies must be registered with an ICMJE-approved registry, such as ClinicalTrials.gov. I confirm that any such study reported in the manuscript has been registered and the trial registration ID is provided (note: if posting a prospective study registered retrospectively, please provide a statement in the trial ID field explaining why the study was not registered in advance).
Yes
I have followed all appropriate research reporting guidelines, such as any relevant EQUATOR Network research reporting checklist(s) and other pertinent material, if applicable.
Yes
Data generated or analyzed during the study are available from the corresponding author by request.
更多查看译文
关键词
high performance embolism detection,deep learning,real-world
AI 理解论文
溯源树
样例
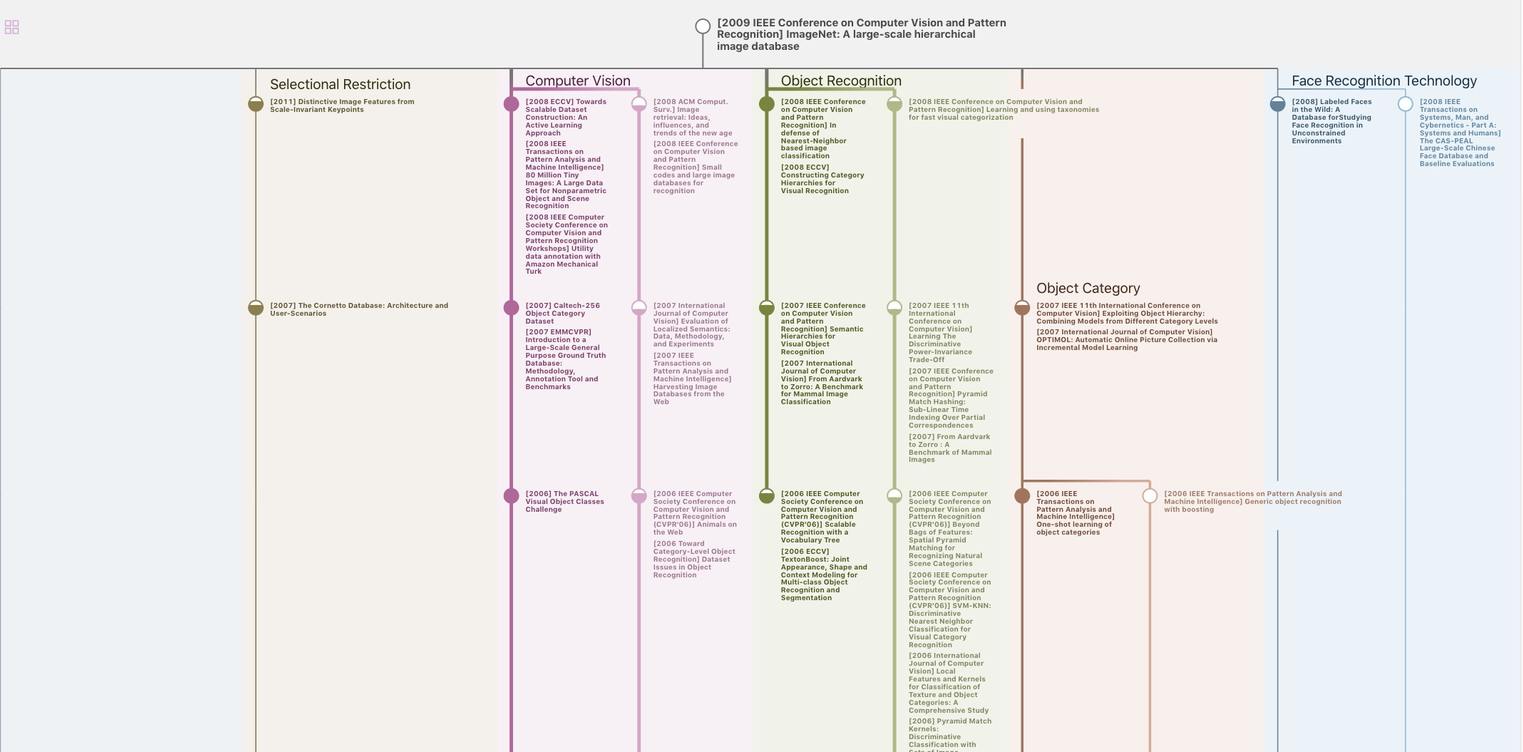
生成溯源树,研究论文发展脉络
Chat Paper
正在生成论文摘要