Transcriptomics profiling of Parkinson’s disease progression subtypes reveals distinctive patterns of gene expression
medRxiv (Cold Spring Harbor Laboratory)(2023)
摘要
Parkinson’s Disease (PD) exhibits significant individual variability, and recent Artificial Intelligence advancements have identified three distinct progression subtypes, each with known clinical features but unexplored gene expression profiles. This study aimed to identify the transcriptomics characteristics of PD progression subtypes, and assess the utility of gene expression data in subtype prediction at baseline. Differentially expressed genes were subtype-specific, and not typically found in other PD studies. Pathway analysis showed distinct and shared features among subtypes. Two had opposing expression patterns for shared pathways, and the third had a more unique profile with respect to the others. Machine Learning revealed that the progression subtype with the worst prognosis can be predicted at baseline with 0.877 AUROC, yet the contribution of gene expression was marginal for the prediction of the subtypes. This study offers insights into PD subtypes transcriptomics, fostering precision medicine for improved diagnosis and prognosis.
### Competing Interest Statement
The authors have declared no competing interest.
### Funding Statement
This work was partially supported by Ricerca Corrente grants (Italian Ministry of Health) from the Santa Lucia Foundation IRCCS - Linea di Ricerca A: Neurologia Clinica e Comportamentale.
### Author Declarations
I confirm all relevant ethical guidelines have been followed, and any necessary IRB and/or ethics committee approvals have been obtained.
Yes
The details of the IRB/oversight body that provided approval or exemption for the research described are given below:
The Parkinson's Progression Markers Initiative (PPMI) study was approved by the Institutional Review Boards of respective institutions (a full list is available as a supplementary file or at the following link ). Written informed consent were obtained from each participant at enrollment, in accordance with the Declaration of Helsinki. All methods were performed in accordance with the relevant guidelines and regulations.
I confirm that all necessary patient/participant consent has been obtained and the appropriate institutional forms have been archived, and that any patient/participant/sample identifiers included were not known to anyone (e.g., hospital staff, patients or participants themselves) outside the research group so cannot be used to identify individuals.
Yes
I understand that all clinical trials and any other prospective interventional studies must be registered with an ICMJE-approved registry, such as ClinicalTrials.gov. I confirm that any such study reported in the manuscript has been registered and the trial registration ID is provided (note: if posting a prospective study registered retrospectively, please provide a statement in the trial ID field explaining why the study was not registered in advance).
Yes
I have followed all appropriate research reporting guidelines, such as any relevant EQUATOR Network research reporting checklist(s) and other pertinent material, if applicable.
Yes
Data used in the preparation of this article were obtained from the Parkinson's Progression Markers Initiative (PPMI) database ([www.ppmi-info.org/access-data-specimens/download-data][1]), RRID:SCR_006431. The PPMI IDs of the subjects in the disease subtypes were obtained from the GitHub repository related to the following article: Zhang, X., Chou, J., Liang, J. et al. Data-Driven Subtyping of Parkinson's Disease Using Longitudinal Clinical Records: A Cohort Study. Sci Rep 9, 797 (2019).
[1]: http://www.ppmi-info.org/access-data-specimens/download-data
更多查看译文
关键词
parkinson disease,disease progression subtypes,gene expression
AI 理解论文
溯源树
样例
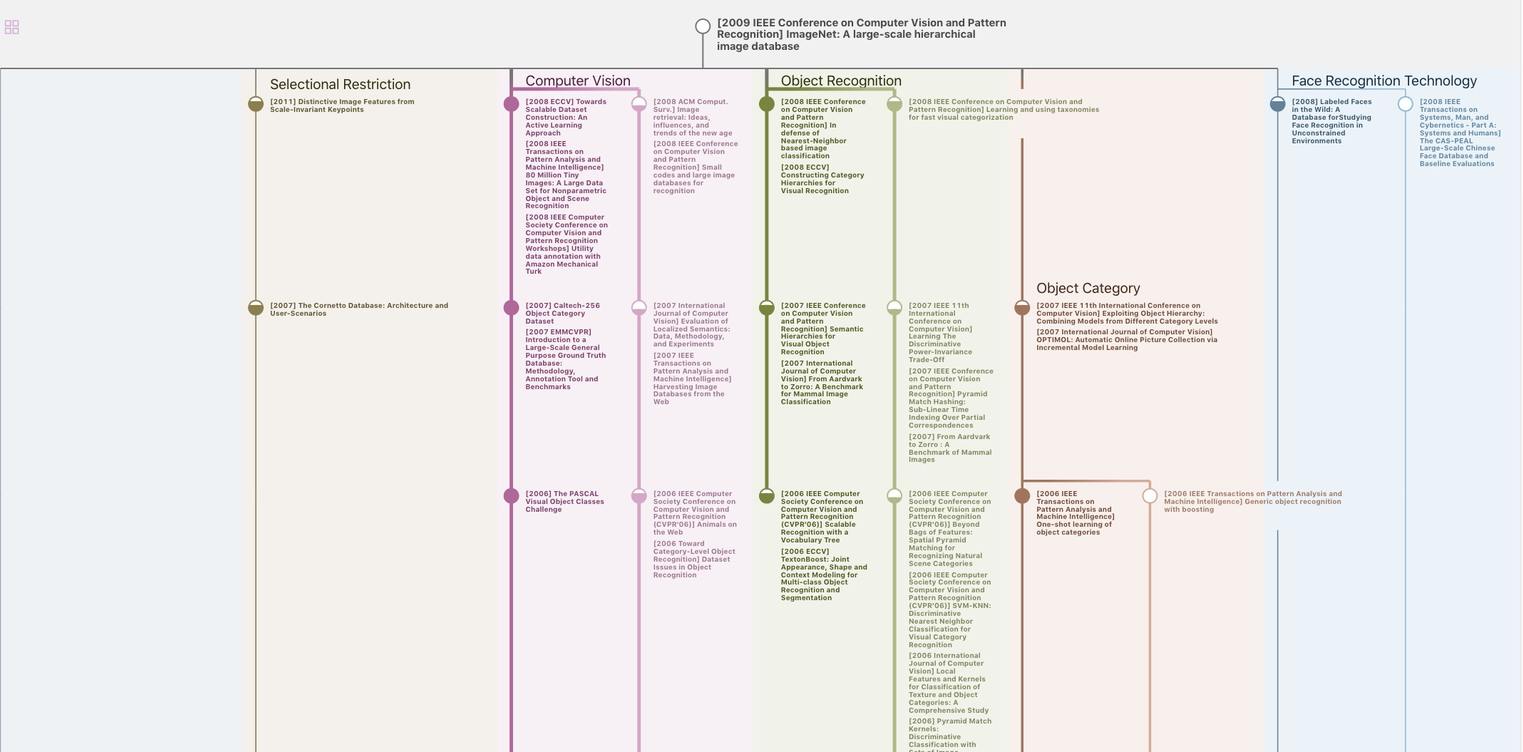
生成溯源树,研究论文发展脉络
Chat Paper
正在生成论文摘要