Model Merging by Uncertainty-Based Gradient Matching
arXiv (Cornell University)(2023)
摘要
Models trained on different datasets can be merged by a weighted-averaging of their parameters, but why does it work and when can it fail? Here, we connect the inaccuracy of weighted-averaging to mismatches in the gradients and propose a new uncertainty-based scheme to improve the performance by reducing the mismatch. The connection also reveals implicit assumptions in other schemes such as averaging, task arithmetic, and Fisher-weighted averaging. Our new method gives consistent improvements for large language models and vision transformers, both in terms of performance and robustness to hyperparameters.
更多查看译文
关键词
matching,model,gradient,uncertainty-based
AI 理解论文
溯源树
样例
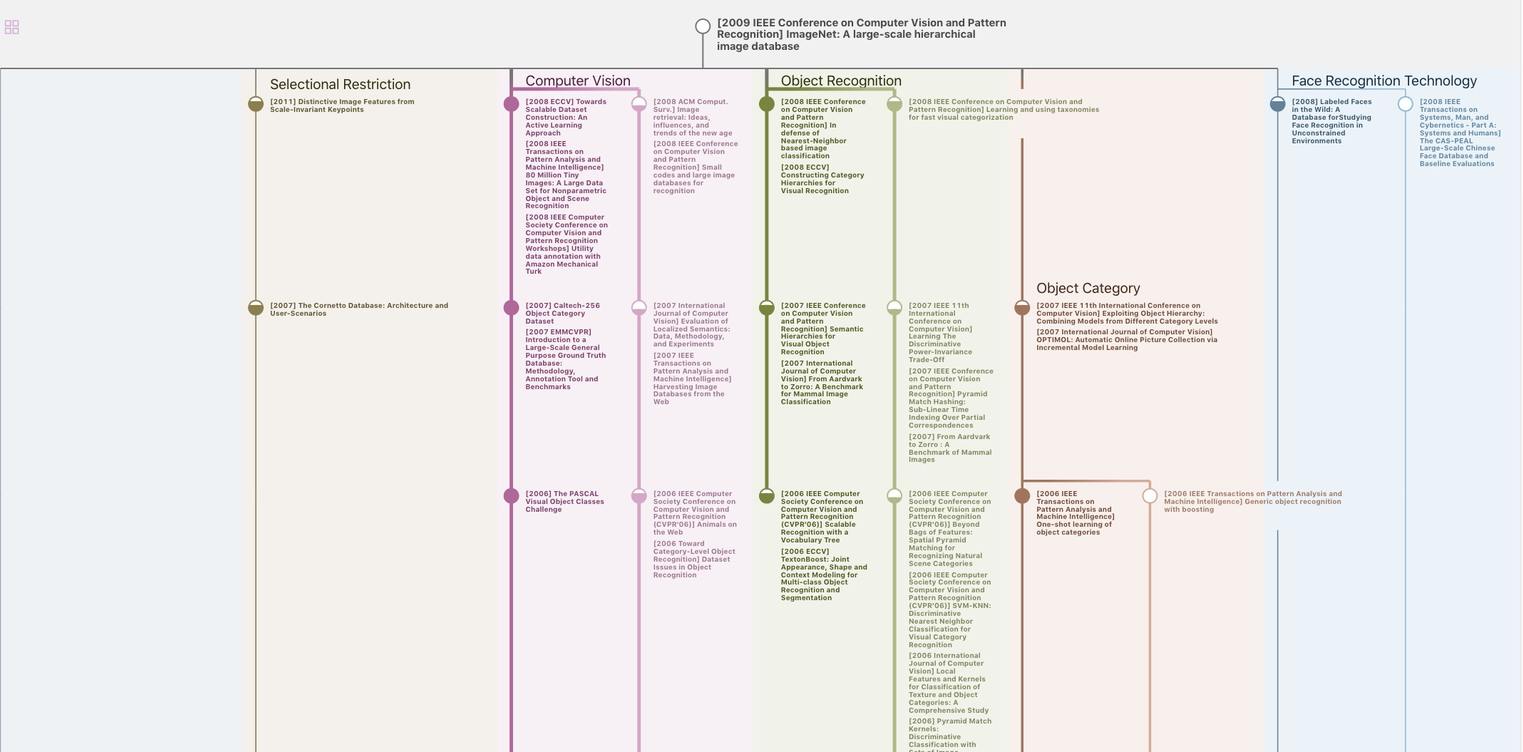
生成溯源树,研究论文发展脉络
Chat Paper
正在生成论文摘要