Exploring the impact of trip patterns on spatially aggregated crashes using floating vehicle trajectory data and graph Convolutional Networks
ACCIDENT ANALYSIS AND PREVENTION(2024)
摘要
In recent years, increased attention has been given to understanding the spatial pattern of crashes in urban areas. Accurately capturing the spatial relationship between crash counts and variables requires extracting hidden information from multiple data sources. In this study, we propose a machine learning model to explore the spatial impact of activity patterns on spatially aggregated crash counts. Our paper introduces a two-step framework: (a) the Latent Dirichlet Allocation (LDA) model, an unsupervised method for mining hidden activity patterns from floating vehicle trajectory data, and (b) the Graph Convolutional Network (GCN) model, which builds the spatial relationship between multi-source data. The data and hidden activity patterns were aggregated into 175 Traffic Analysis Zones (TAZs) in San Francisco using spatial partitioning. The GCN model provided higher prediction accuracy than commonly used machine learning algorithms that did not consider combined spatial relationships and those that only considered traditional vehicle counts data. Furthermore, we used attribution algorithms to obtain the respective weight scores of each factor. Our results reveal that daily vehicle kilometers traveled, road density, population density, commercial activity during weekends, and residential activity during morning peak hours on weekdays are factors associated with crashes.
更多查看译文
关键词
Activity Patterns,Floating Vehicle Trajectory Data,Spatial Analysis of Crashes,Latent Dirichlet Allocation,Graph Convolutional Network,Attribution Algorithms
AI 理解论文
溯源树
样例
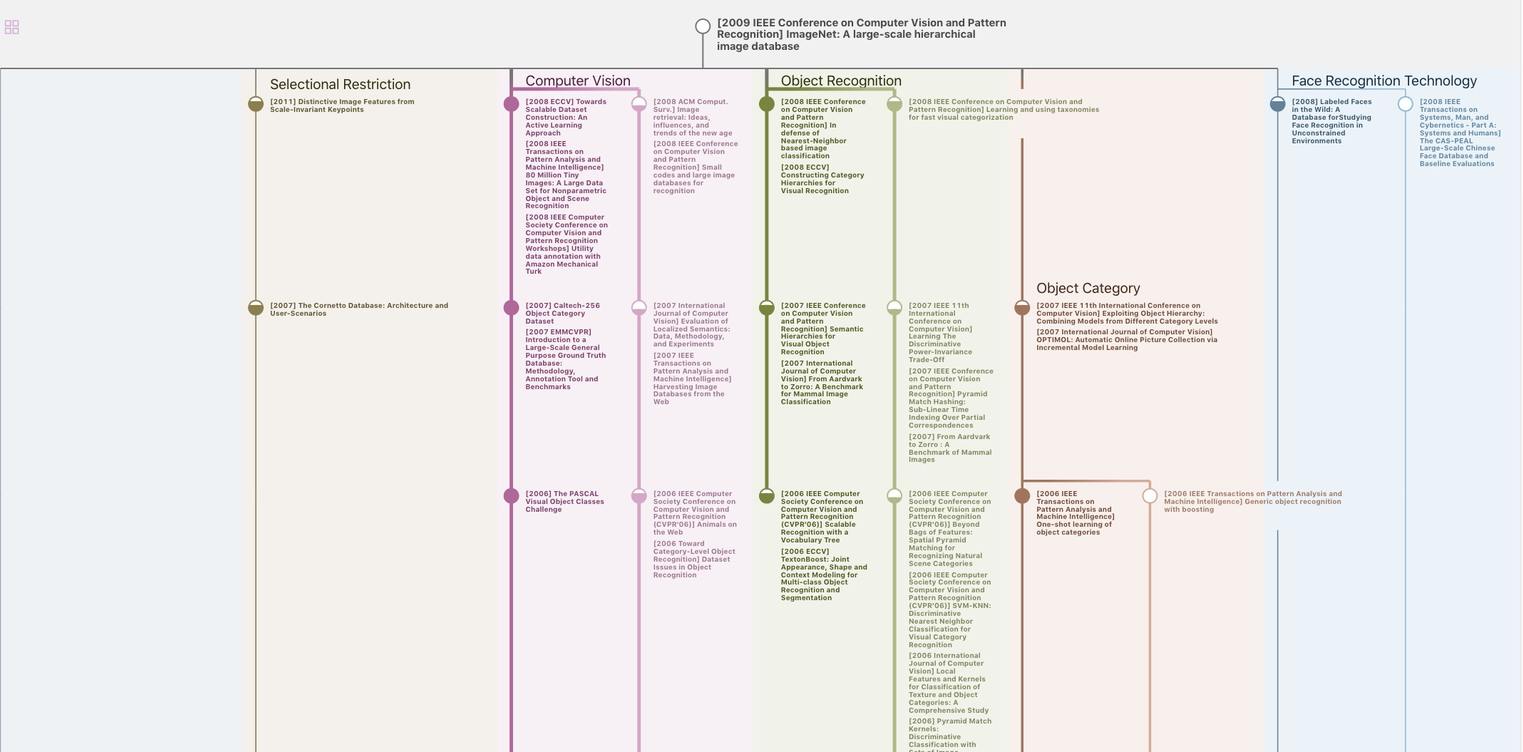
生成溯源树,研究论文发展脉络
Chat Paper
正在生成论文摘要