Simulating doctors' thinking logic for chest X-ray report generation via Transformer-based Semantic Query learning
MEDICAL IMAGE ANALYSIS(2024)
摘要
Medical report generation can be treated as a process of doctors' observing, understanding, and describing images from different perspectives. Following this process, this paper innovatively proposes a Transformer -based Semantic Query learning paradigm (TranSQ). Briefly, this paradigm is to learn an intention embedding set and make a semantic query to the visual features, generate intent-compliant sentence candidates, and form a coherent report. We apply a bipartite matching mechanism during training to realize the dynamic correspon-dence between the intention embeddings and the sentences to induct medical concepts into the observation intentions. Experimental results on two major radiology reporting datasets (i.e., IU X-ray and MIMIC-CXR) demonstrate that our model outperforms state-of-the-art models regarding generation effectiveness and clinical efficacy. In addition, comprehensive ablation experiments fully validate the TranSQ model's innovation and interpretation. The code is available at https://github.com/zjukongming/TranSQ.
更多查看译文
关键词
Medical report generation,Semantic query,query Transformer,Computer-aided diagnosis,Deep learning
AI 理解论文
溯源树
样例
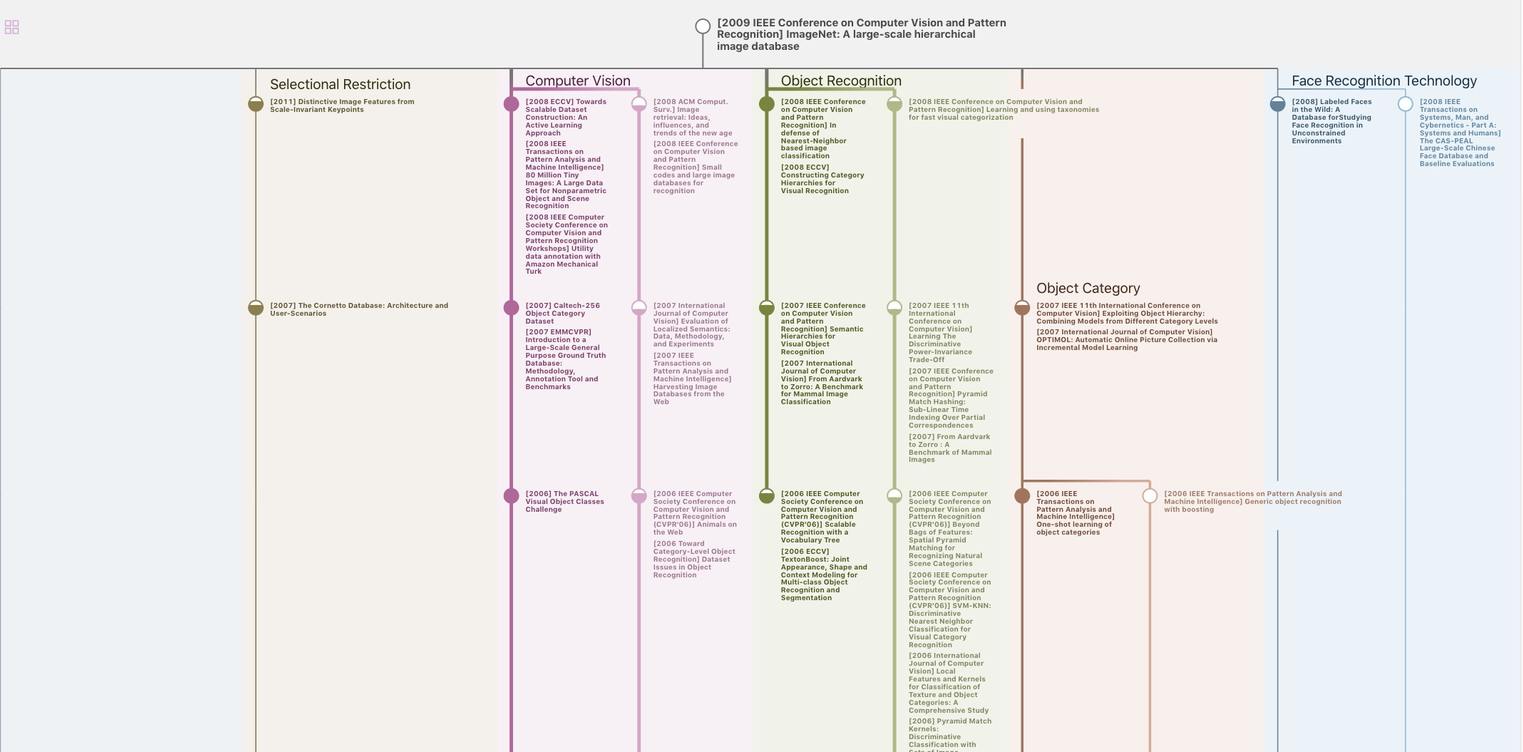
生成溯源树,研究论文发展脉络
Chat Paper
正在生成论文摘要