An Online Learning Theory of Brokerage
International Joint Conference on Autonomous Agents & Multiagent Systems(2023)
摘要
We investigate brokerage between traders from an online learning perspective. At any round $t$, two traders arrive with their private valuations, and the broker proposes a trading price. Unlike other bilateral trade problems already studied in the online learning literature, we focus on the case where there are no designated buyer and seller roles: each trader will attempt to either buy or sell depending on the current price of the good. We assume the agents' valuations are drawn i.i.d. from a fixed but unknown distribution. If the distribution admits a density bounded by some constant $M$, then, for any time horizon $T$: $\bullet$ If the agents' valuations are revealed after each interaction, we provide an algorithm achieving regret $M \log T$ and show this rate is optimal, up to constant factors. $\bullet$ If only their willingness to sell or buy at the proposed price is revealed after each interaction, we provide an algorithm achieving regret $\sqrt{M T}$ and show this rate is optimal, up to constant factors. Finally, if we drop the bounded density assumption, we show that the optimal rate degrades to $\sqrt{T}$ in the first case, and the problem becomes unlearnable in the second.
更多查看译文
AI 理解论文
溯源树
样例
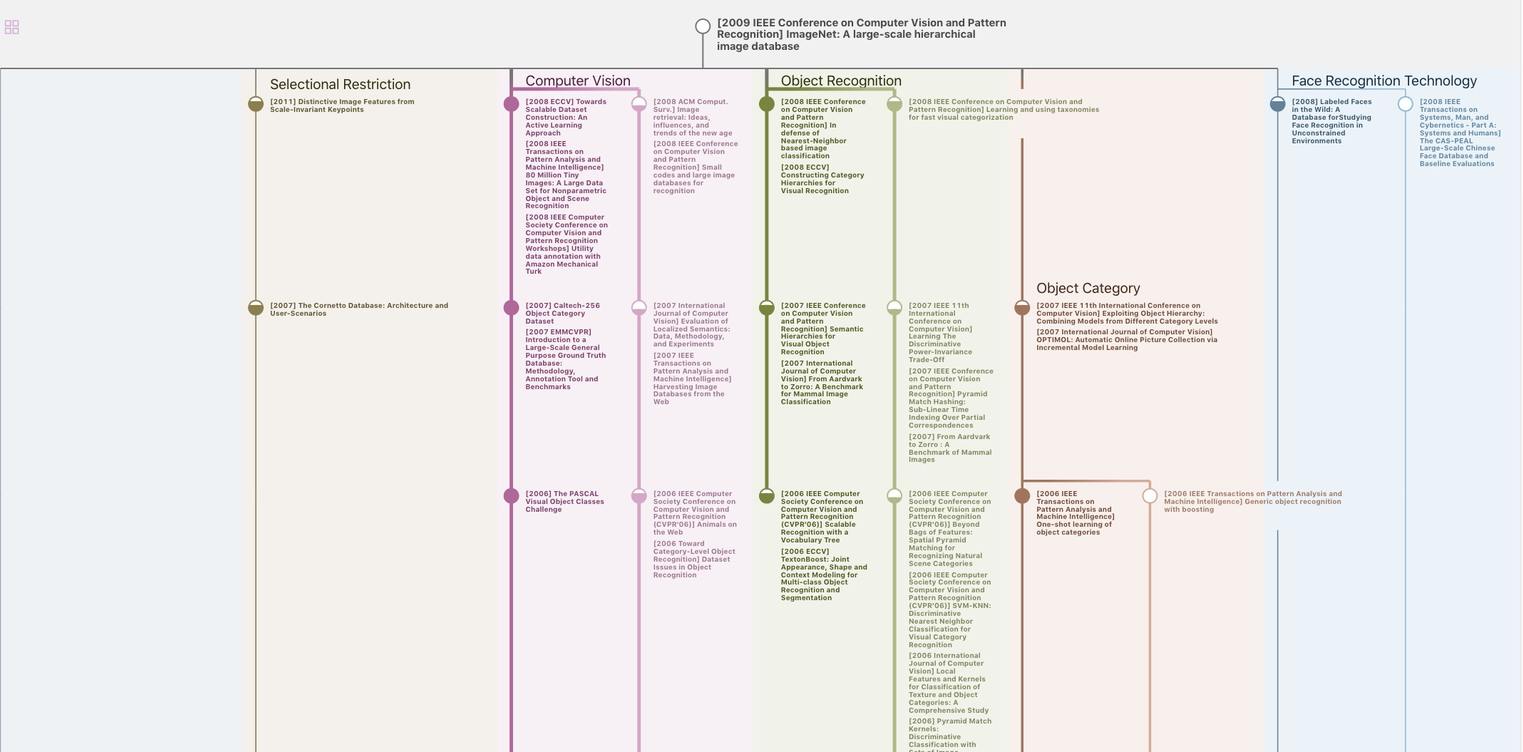
生成溯源树,研究论文发展脉络
Chat Paper
正在生成论文摘要