Partially Observable Stochastic Games with Neural Perception Mechanisms
CoRR(2023)
摘要
Stochastic games are a well established model for multi-agent sequential decision making under uncertainty. In reality, though, agents have only partial observability of their environment, which makes the problem computationally challenging, even in the single-agent setting of partially observable Markov decision processes. Furthermore, in practice, agents increasingly perceive their environment using data-driven approaches such as neural networks trained on continuous data. To tackle this problem, we propose the model of neuro-symbolic partially-observable stochastic games (NS-POSGs), a variant of continuous-space concurrent stochastic games that explicitly incorporates perception mechanisms. We focus on a one-sided setting, comprising a partially-informed agent with discrete, data-driven observations and a fully-informed agent with continuous observations. We present a new point-based method, called one-sided NS-HSVI, for approximating values of one-sided NS-POSGs and implement it based on the popular particle-based beliefs, showing that it has closed forms for computing values of interest. We provide experimental results to demonstrate the practical applicability of our method for neural networks whose preimage is in polyhedral form.
更多查看译文
关键词
observable stochastic games,perception
AI 理解论文
溯源树
样例
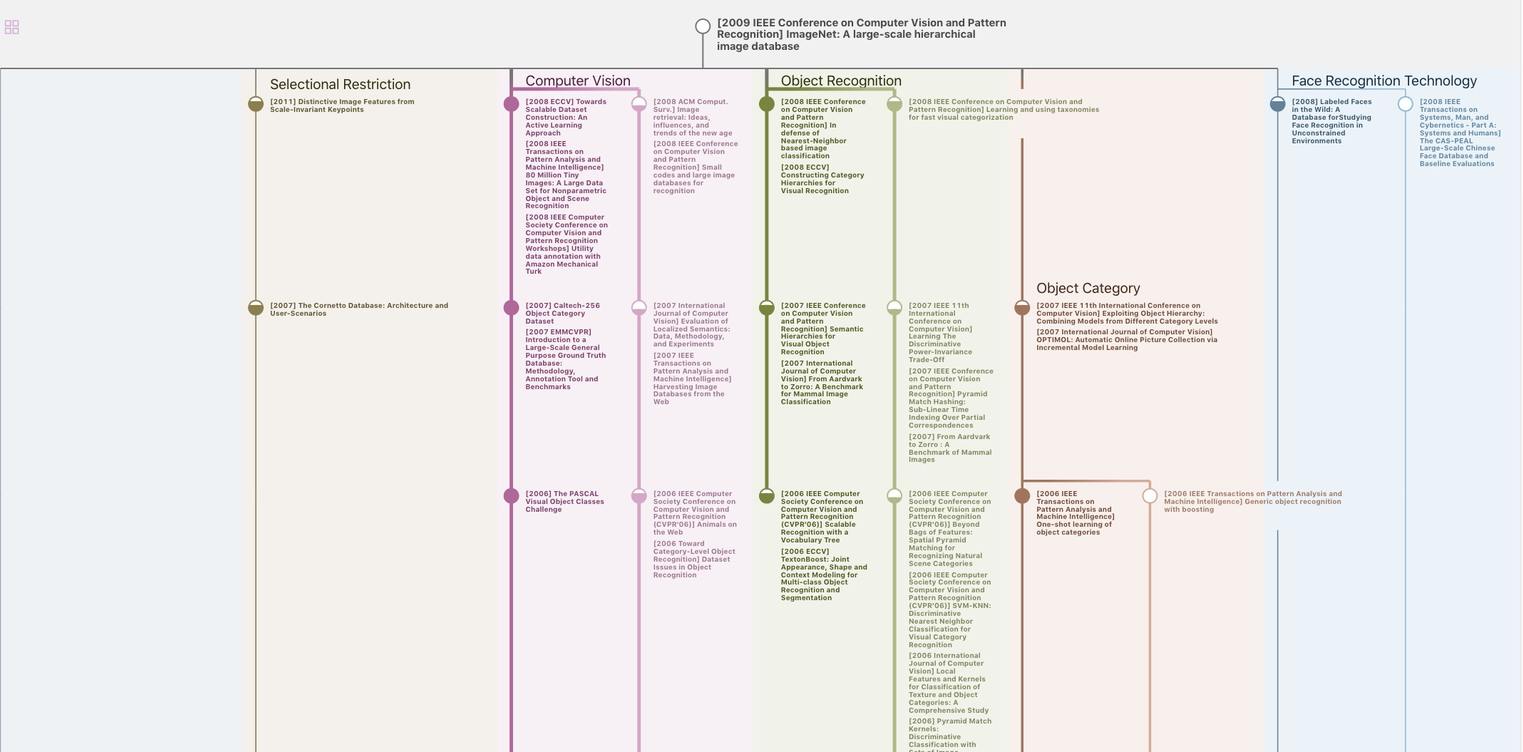
生成溯源树,研究论文发展脉络
Chat Paper
正在生成论文摘要