Hierarchical multi-agent reinforcement learning for cooperative tasks with sparse rewards in continuous domain
NEURAL COMPUTING & APPLICATIONS(2024)
摘要
The sparse reward problem has long been one of the most challenging topics in the application of reinforcement learning (RL), especially in complex multi-agent systems. In this paper, a hierarchical multi-agent RL architecture is developed to address the sparse reward problem of cooperative tasks in continuous domain. The proposed architecture is divided into two levels: the higher-level meta-agent implements state transitions on a larger time scale to alleviate the sparse reward problem, which receives global observation as spatial information and formulates sub-goals for the lower-level agents; the lower-level agent receives local observation and sub-goal and completes the cooperative tasks. In addition, to improve the stability of the higher-level policy, a channel is built to transmit the lower-level policy to the meta-agent as temporal information, and then a two-stream structure is adopted in the actor-critic networks of the meta-agent to process spatial and temporal information. Simulation experiments on different tasks demonstrate that the proposed algorithm effectively alleviates the sparse reward problem, so as to learn desired cooperative policies.
更多查看译文
关键词
Reinforcement learning,Sparse reward,Cooperative multi-agent systems,Hierarchical framework,Two-stream structure
AI 理解论文
溯源树
样例
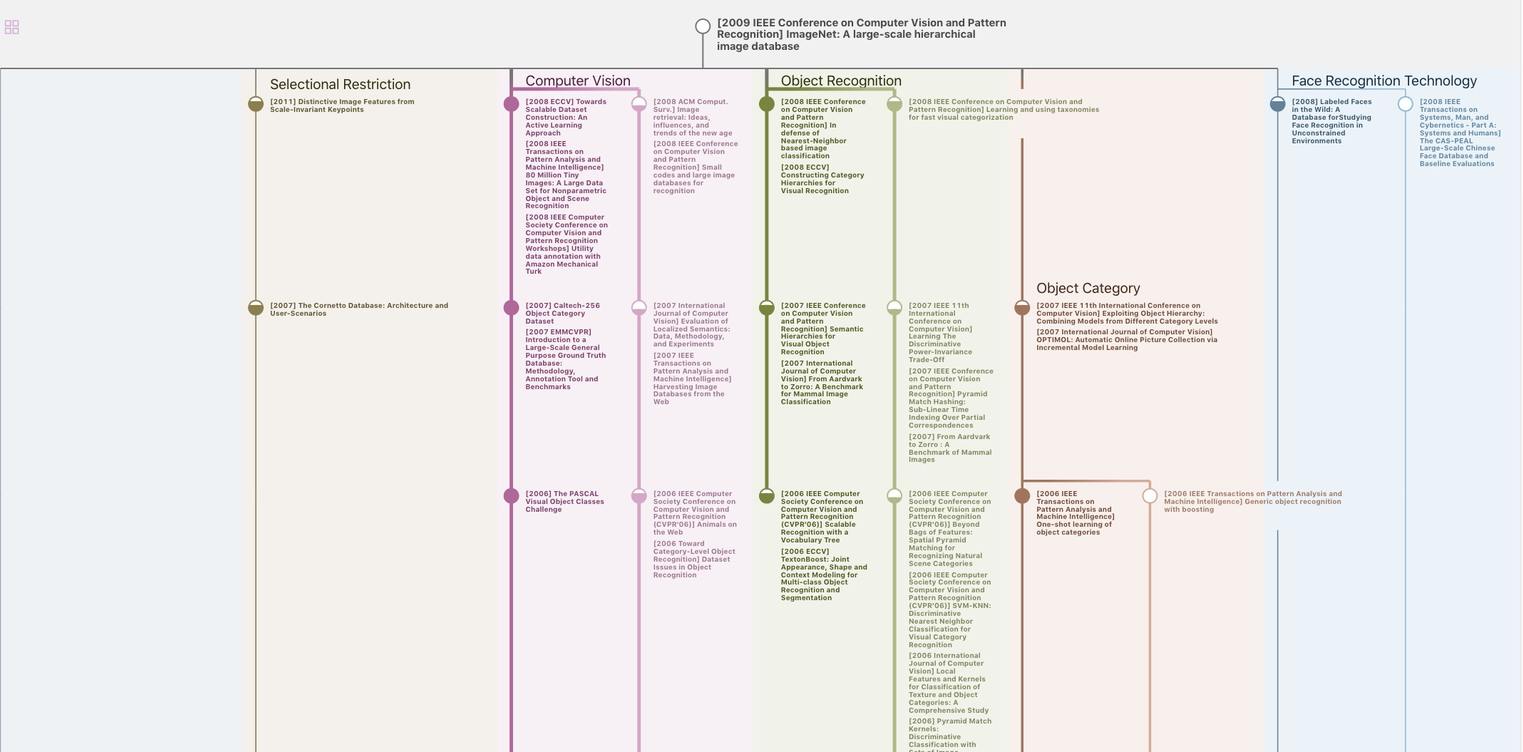
生成溯源树,研究论文发展脉络
Chat Paper
正在生成论文摘要