Enhancing the diagnosis of autism spectrum disorder using phenotypic, structural, and functional MRI data
INNOVATIONS IN SYSTEMS AND SOFTWARE ENGINEERING(2023)
摘要
Autism spectrum disorder (ASD) is a complex and heterogeneous neurodevelopmental disorder. Machine learning and deep learning techniques have been playing an important role in automating the diagnosis of brain disorder, which is characterized by social deficits and repetitive behaviors. In this paper, we have proposed and implemented a machine learning model and convolution neural network (CNN) for classifying subjects with ASD. Data are from Autism Brain Imagining Data Exchange (ABIDE) repository by using phenotypic, sMRI, and fMRI data. For sMRI image dataset, the accuracy of the neural network is about 87%, whereas for fMRI image dataset the accuracy is 88%, which is suitable for real-time usage. We implemented a GUI called Gradio for visualizing the sMRI and fMRI data analysis. The different ML techniques used for phenotypic data were K-nearest neighbors, decision tree, random forest, SVM, and logistic regression. The work also interpreted the different machine learning (ML) models for the clinical data for ASD screening of children (toddlers), which are available in the UCI repository; different ML techniques—K-nearest neighbors, decision tree, random forest, and Naïve Bayes—are used. The proposed methodology can detect and diagnose ASD at early stage. An automated system helps in faster diagnosis, and even minute things are identified and observed. Sometimes, humans can fail in identifying such minute things in the sample while diagnosing. To build such a system, deep learning models such as CNN models are trained on the sMRI and fMRI images to classify them into ASD and non-ASD. The classification capability of the developed system was measured using the performance metrics such as accuracy, ROC (receiver operating characteristic) curve, and AUC (area under the curve). The automated system can detect whether the given image is ASD or normal. Doctors can use this automated system very easily and do the needful. The novelty of our work is that we have considered the 3 modalities, for predicting the diseases. As a future work, we can do a fusion to give more accurate result combining the 3 modalities.
更多查看译文
关键词
Autism spectrum disorder (ASD),ABIDE,ASD screening,Convolution neural network (CNN),Deep learning (DL),GUI,Gradio,Magnetic resonance imaging (MRI),Machine Learning (ML),Phenotypic data,fMRI,sMRI
AI 理解论文
溯源树
样例
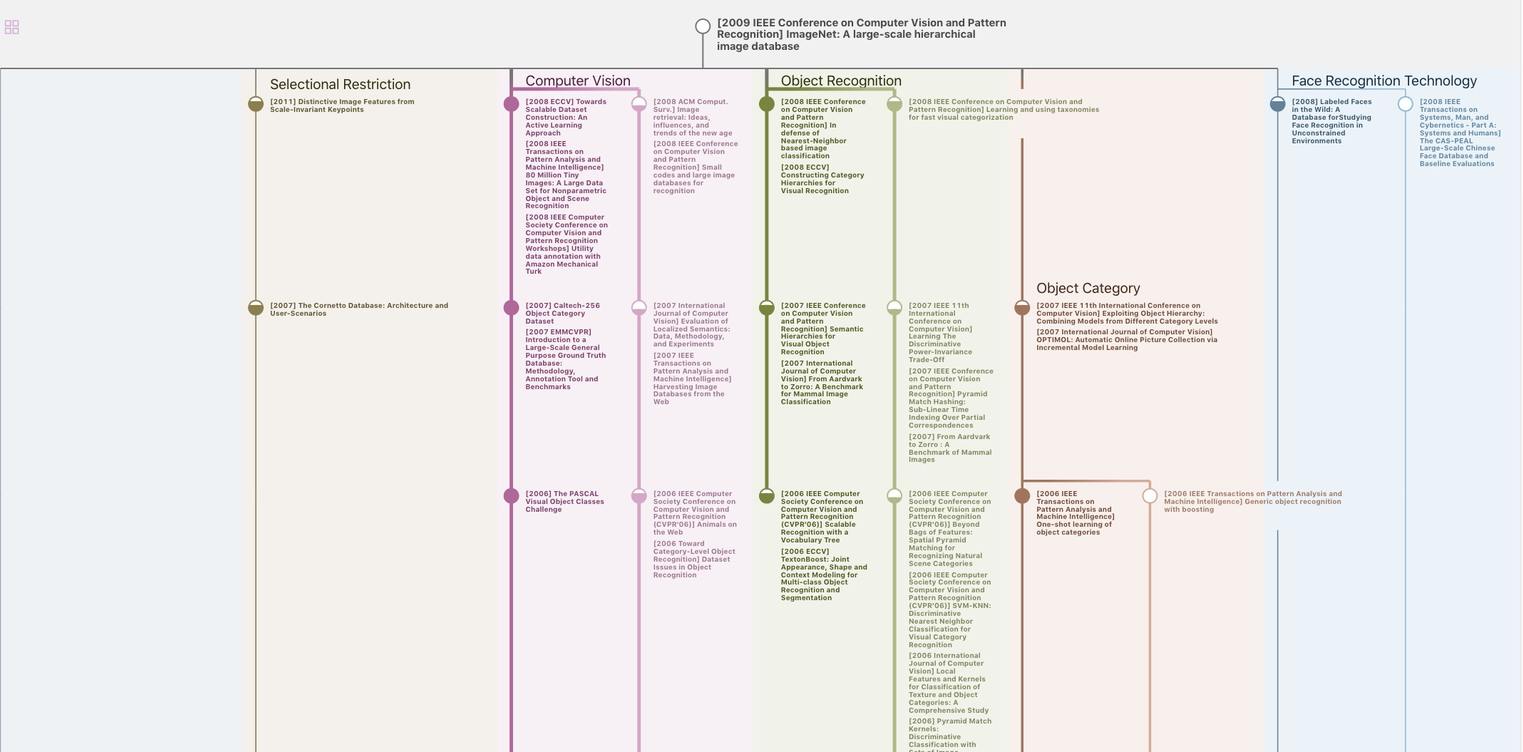
生成溯源树,研究论文发展脉络
Chat Paper
正在生成论文摘要