TDG4MSF: A temporal decomposition enhanced graph neural network for multivariate time series forecasting
Applied Intelligence(2023)
摘要
Multivariate time series forecasting is an important issue in industries, agriculture, finance, and other applications. There are many challenging problems in it such as non-linear and complicated relationships among the series. The entanglement of latent multiple different sequence patterns maybe one of the most reasons for the time series complex behavior, and decomposition can help reveal the hidden evolution law. Graph is a good modelling tool for multiple entities and graph neural network has showed better learning ability for spatial dependence, but its high memory consumption requires valid solutions. Inspired by the above two points, we propose a Temporal Decomposition enhanced Graph neural network for Multivariate time Series Forecasting, namely TDG4MSF, which mainly consists of four components: temporal decomposition enhanced representation learning, graph structure learning, gated GNNML-based representation learning and MLP-based forecasting. A progressive quadratic decomposition architecture is designed in the temporal decomposition enhanced representation learning that extracts the different periodic patterns of time series. Graph structure learning is used to construct adjacency matrix to represent the spatial topological structure. The temporal decomposition enhanced representation and adjacency matrix are fed into gated GNNML to integrating temporal and spatial information between variables. A MLP-based forecasting is utilized to make multivariate time series prediction. Experimental results show the effectiveness of our model in short- and medium-term prediction scenarios are superior to the state-of-the-art methods, which help make exact decisions timely. Code is available at: https://github.com/TYUT-Theta/MHZN.git .
更多查看译文
关键词
Multivariate time series,Temporal decomposition,Graph neural networks,Short- and medium-term forecasting
AI 理解论文
溯源树
样例
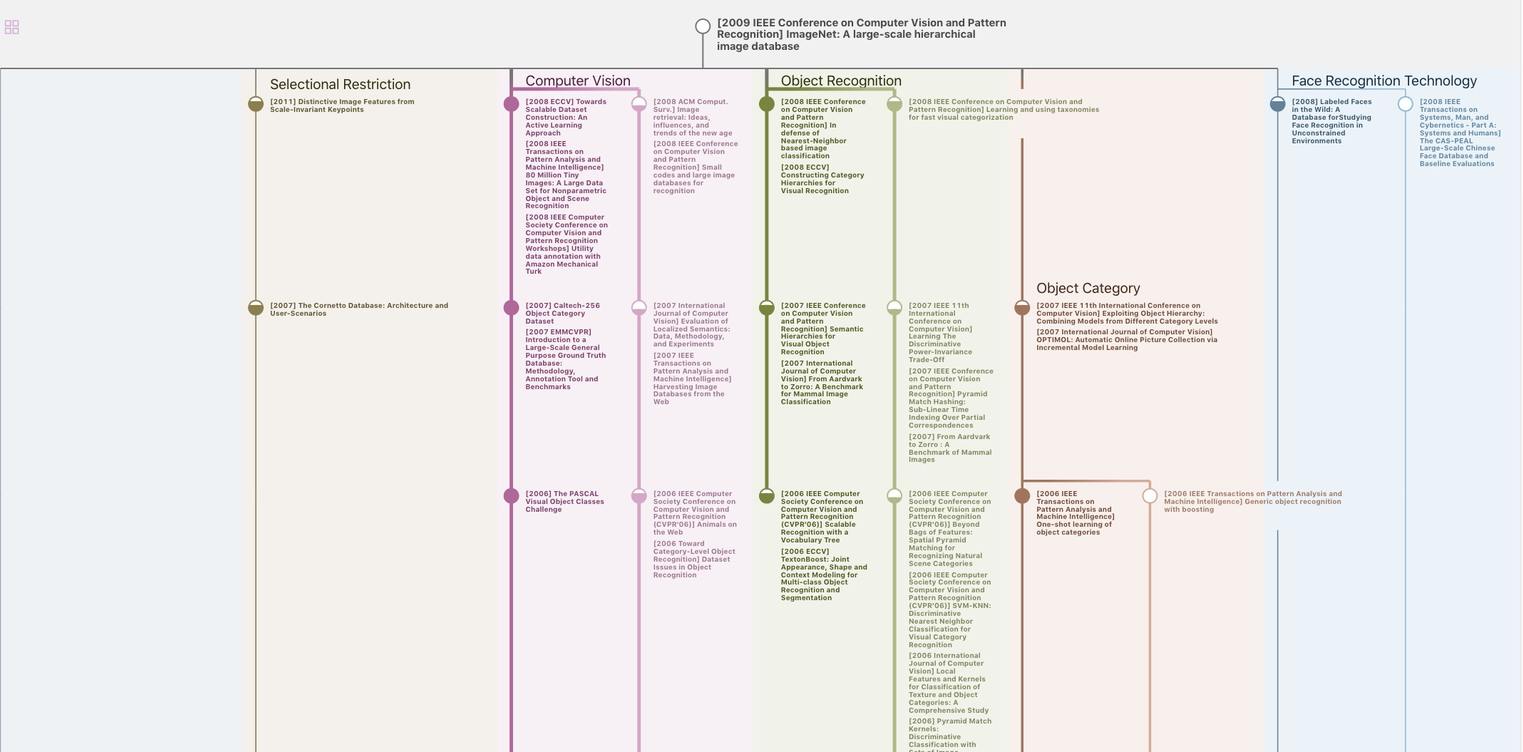
生成溯源树,研究论文发展脉络
Chat Paper
正在生成论文摘要