Unsupervised Discovery of Interpretable Directions in h-space of Pre-trained Diffusion Models
CoRR(2023)
摘要
We propose the first unsupervised and learning-based method to identify interpretable directions in the h-space of pre-trained diffusion models. Our method is derived from an existing technique that operates on the GAN latent space. In a nutshell, we employ a shift control module for pre-trained diffusion models to manipulate a sample into a shifted version of itself, followed by a reconstructor to reproduce both the type and the strength of the manipulation. By jointly optimizing them, the model will spontaneously discover disentangled and interpretable directions. To prevent the discovery of meaningless and destructive directions, we employ a discriminator to maintain the fidelity of shifted sample. Due to the iterative generative process of diffusion models, our training requires a substantial amount of GPU VRAM to store numerous intermediate tensors for back-propagating gradient. To address this issue, we first propose a general VRAM-efficient training algorithm based on gradient checkpointing technique to back-propagate any gradient through the whole generative process, with acceptable occupancy of VRAM and sacrifice of training efficiency. Compared with existing related works on diffusion models, our method inherently identifies global and scalable directions, without necessitating any other complicated procedures. Extensive experiments on various datasets demonstrate the effectiveness of our method.
更多查看译文
关键词
diffusion models,interpretable directions,h-space,pre-trained
AI 理解论文
溯源树
样例
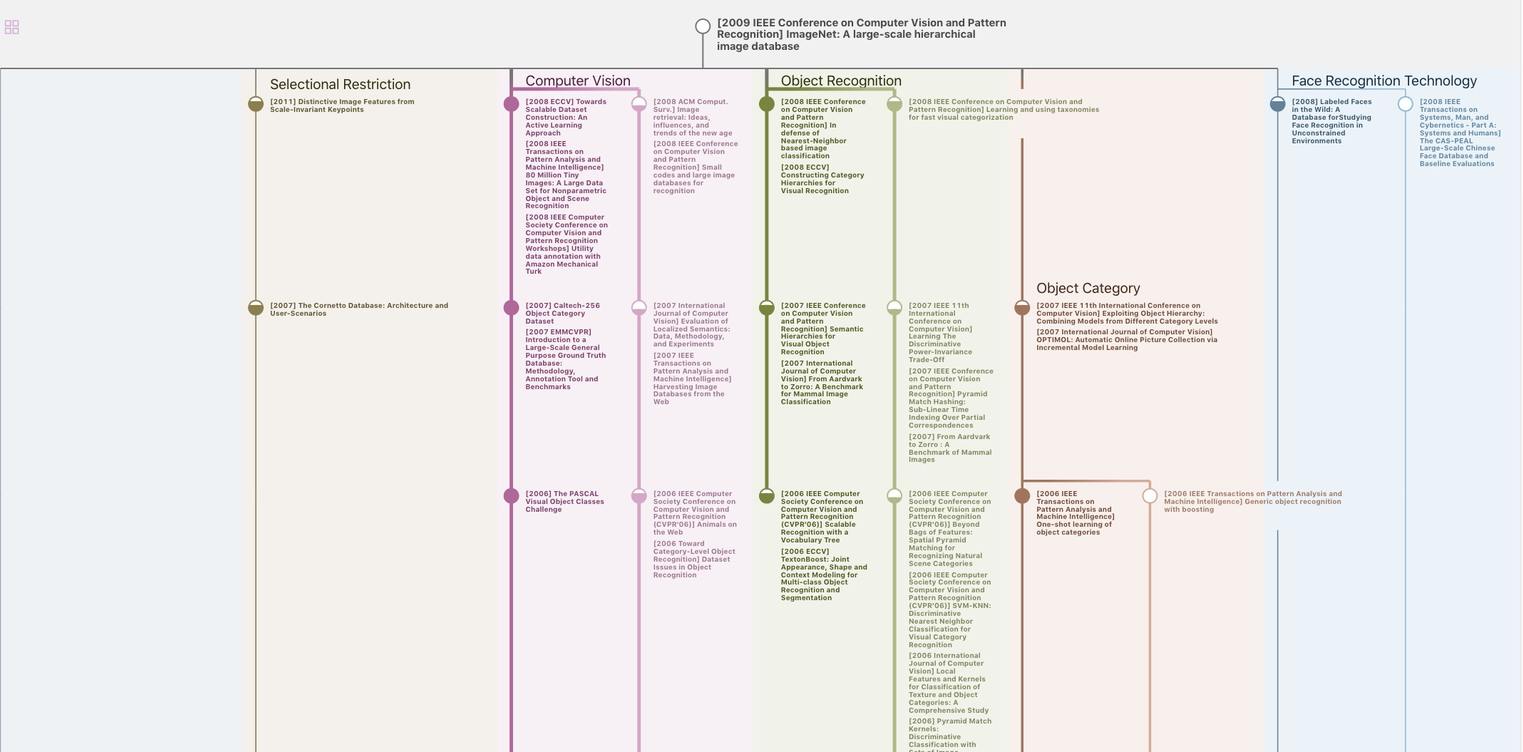
生成溯源树,研究论文发展脉络
Chat Paper
正在生成论文摘要