Enhancing Conversational Search: Large Language Model-Aided Informative Query Rewriting.
CoRR(2023)
摘要
Query rewriting plays a vital role in enhancing conversational search by transforming context-dependent user queries into standalone forms. Existing approaches primarily leverage human-rewritten queries as labels to train query rewriting models. However, human rewrites may lack sufficient information for optimal retrieval performance. To overcome this limitation, we propose utilizing large language models (LLMs) as query rewriters, enabling the generation of informative query rewrites through well-designed instructions. We define four essential properties for well-formed rewrites and incorporate all of them into the instruction. In addition, we introduce the role of rewrite editors for LLMs when initial query rewrites are available, forming a ``rewrite-then-edit'' process. Furthermore, we propose distilling the rewriting capabilities of LLMs into smaller models to reduce rewriting latency. Our experimental evaluation on the QReCC dataset demonstrates that informative query rewrites can yield substantially improved retrieval performance compared to human rewrites, especially with sparse retrievers.
更多查看译文
关键词
conversational search,query,model-aided
AI 理解论文
溯源树
样例
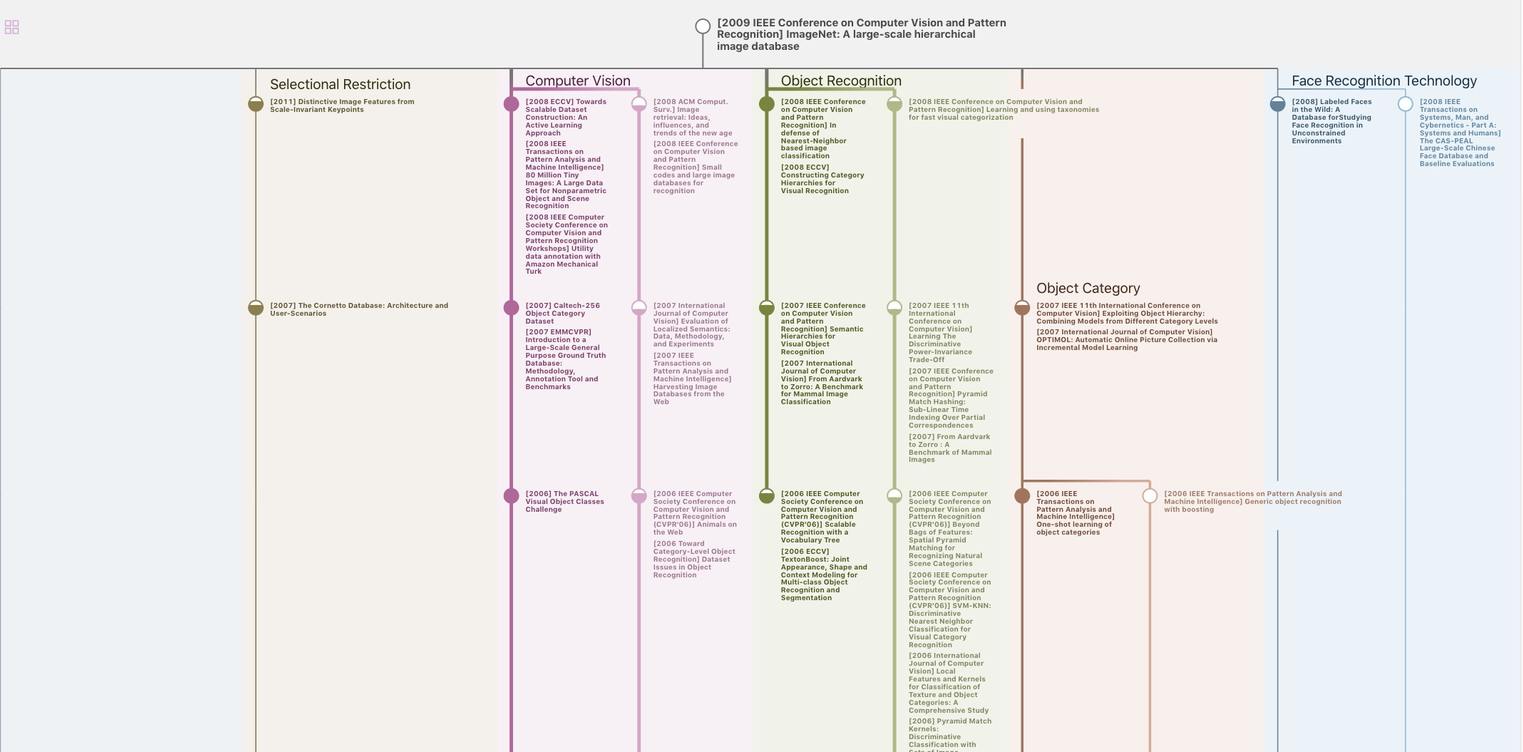
生成溯源树,研究论文发展脉络
Chat Paper
正在生成论文摘要