Groundwater Level Prediction with Machine Learning to Support Sustainable Irrigation in Water Scarcity Regions
Water(2023)
摘要
Predicting groundwater levels is challenging, especially in regions of water scarcity where data availability is often limited. However, these regions have substantial water needs and require cost-effective groundwater utilization strategies. This study uses artificial intelligence to predict groundwater levels to provide guidance for drilling shallow boreholes for subsistence irrigation. The Bilate watershed, located 80 km north of Arba Minch in southern Ethiopia and covering just over 5250 km2, was selected as the study area. Bilate is typical of areas in Africa with high demand for water and limited availability of well data. Using a non-time series database of 75 boreholes, machine learning models, including multiple linear regression, multivariate adaptive regression splines, artificial neural networks, random forest regression, and gradient boosting regression (GBR), were constructed to predict the depth to the water table. The study considered 20 independent variables, including elevation, soil type, and seasonal data (spanning three seasons) for precipitation, specific humidity, wind speed, land surface temperature during day and night, and Normalized Difference Vegetation Index (NDVI). GBR performed the best of the approaches, with an average 0.77 R-squared value and a 19 m median absolute error on testing data. Finally, a map of predicted water levels in the Bilate watershed was created based on the best model, with water levels ranging from 1.6 to 245.9 m. With the limited set of borehole data, the results show a clear signal that can provide guidance for borehole drilling decisions for sustainable irrigation with additional implications for drinking water.
更多查看译文
关键词
machine learning,groundwater table,ground water level,sustainable irrigation,drinking water,water-scarcity regions
AI 理解论文
溯源树
样例
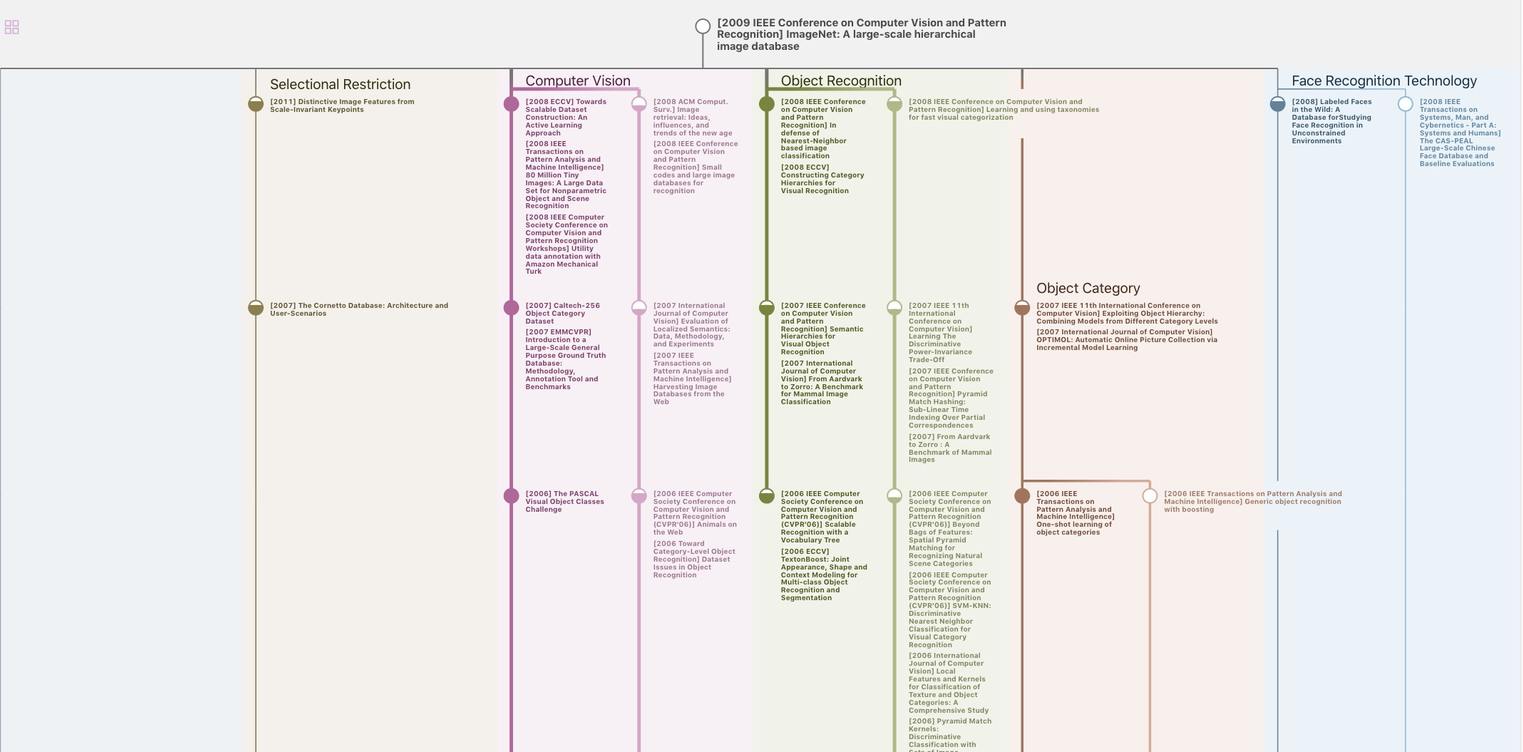
生成溯源树,研究论文发展脉络
Chat Paper
正在生成论文摘要