GLoRE: Evaluating Logical Reasoning of Large Language Models
CoRR(2023)
摘要
Recently, large language models (LLMs), including notable models such as GPT-4 and burgeoning community models, have showcased significant general language understanding abilities. However, there has been a scarcity of attempts to assess the logical reasoning capacities of these LLMs, an essential facet of natural language understanding. To encourage further investigation in this area, we introduce GLoRE, a meticulously assembled General Logical Reasoning Evaluation benchmark comprised of 12 datasets that span three different types of tasks. Our experimental results show that compared to the performance of human and supervised fine-tuning, the logical reasoning capabilities of open LLM models necessitate additional improvement; ChatGPT and GPT-4 show a strong capability of logical reasoning, with GPT-4 surpassing ChatGPT by a large margin. We propose a self-consistency probing method to enhance the accuracy of ChatGPT and a fine-tuned method to boost the performance of an open LLM. We release the datasets and evaluation programs to facilitate future research.
更多查看译文
关键词
logical reasoning,language,models
AI 理解论文
溯源树
样例
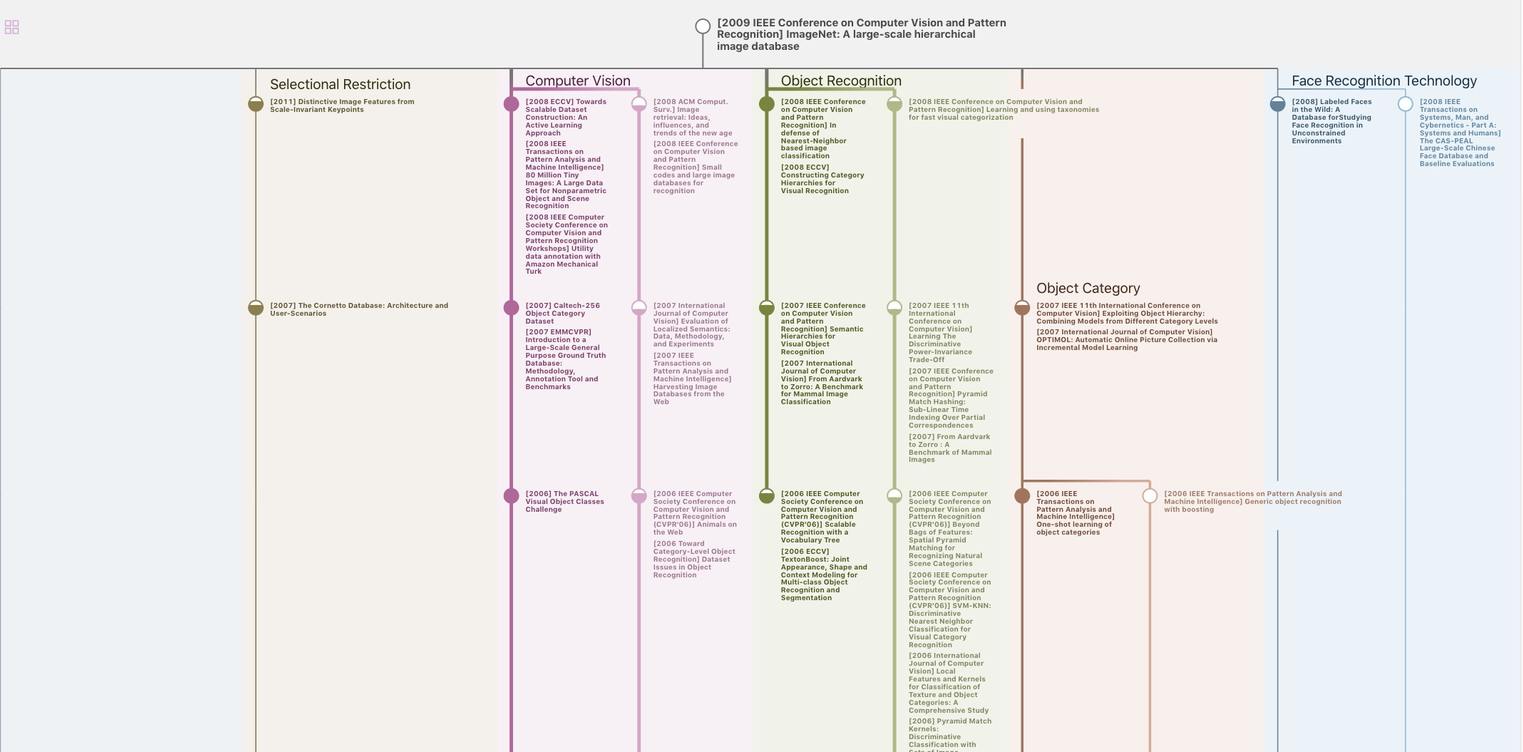
生成溯源树,研究论文发展脉络
Chat Paper
正在生成论文摘要