A forward modeling approach to analyzing galaxy clustering with SIMBIG
PROCEEDINGS OF THE NATIONAL ACADEMY OF SCIENCES OF THE UNITED STATES OF AMERICA(2023)
摘要
We present cosmological constraints from a simulation-based inference (SBI) analysis of galaxy clustering from the SIMBIG forward modeling framework. SIMBIG leverages the predictive power of high-fidelity simulations and provides an inference framework that can extract cosmological information on small nonlinear scales. In this work, we apply SIMBIG to the Baryon Oscillation Spectroscopic Survey (BOSS) CMASS galaxy sample and analyze the power spectrum, P-l(k), to k(max) = 0.5 h=Mpc. We construct 20,000 simulated galaxy samples using our forward model, which is based on 2,000 high-resolution QUIJOTE N-body simulations and includes detailed survey realism for a more complete treatment of observational systematics. Wethen conduct SBI by training normalizing flows using the simulated samples and infer the posterior distribution of Lambda CDM cosmological parameters: Omega(m), Omega(b), h, n(s), sigma(8). We derive significant constraints on Omega(m) and sigma(8), which are consistent with previous works. Our constraint on sigma(8) is 27% more precise than standard P-l analyses because we exploit additional cosmological information on nonlinear scales beyond the limit of current analytic models, k > 0:25 h/Mpc. This improvement is equivalent to the statistical gain expected from a standard P-l analysis of galaxy sample similar to 60% larger than CMASS. While we focus on P-l in this work for validation and comparison to the literature, SIMBIG provides a framework for analyzing galaxy clustering using any summary statistic. We expect further improvements on cosmological constraints from subsequent SIMBIG analyses of summary statistics beyond P-l.
更多查看译文
关键词
cosmology,machine learning,galaxies,simulations
AI 理解论文
溯源树
样例
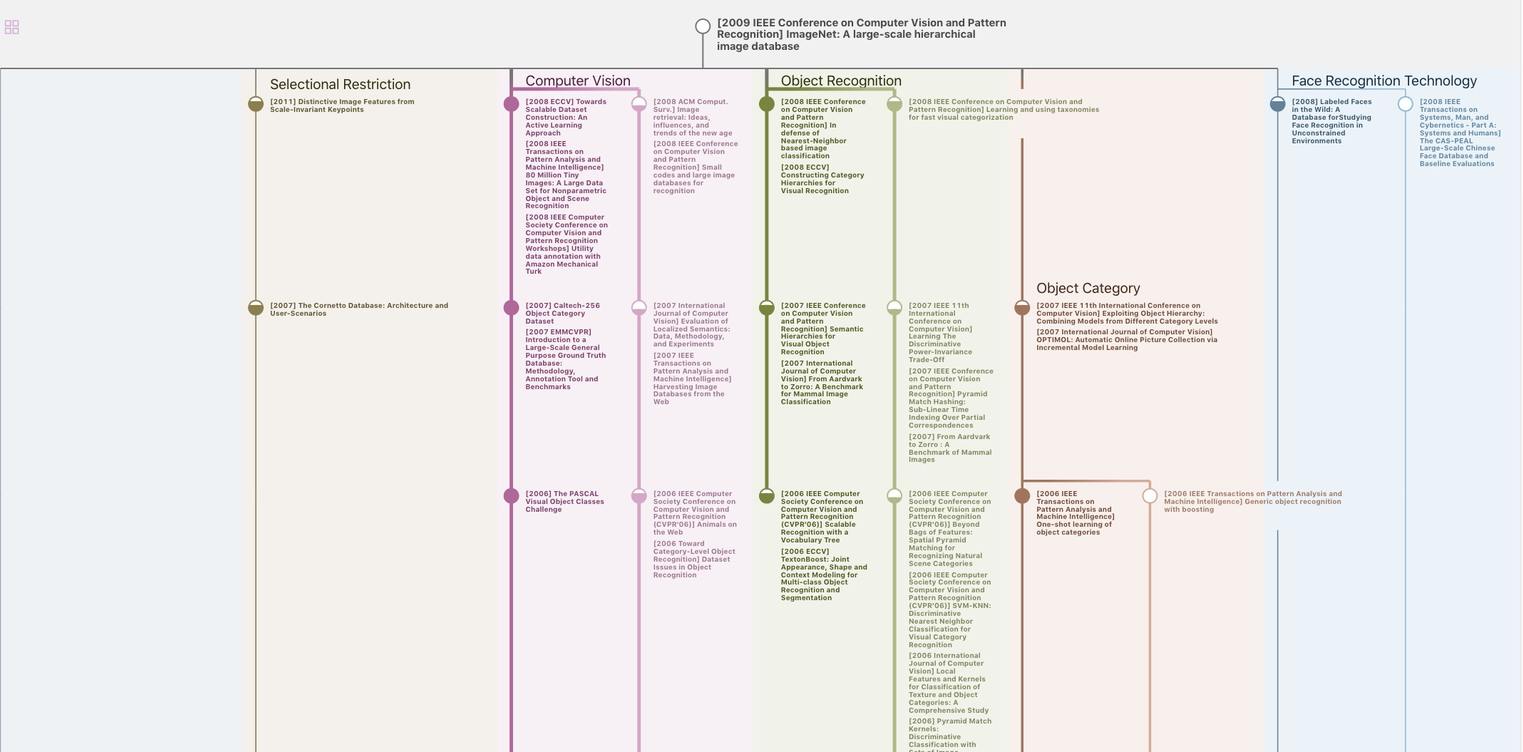
生成溯源树,研究论文发展脉络
Chat Paper
正在生成论文摘要