ReactioNet: Learning High-order Facial Behavior from Universal Stimulus-Reaction by Dyadic Relation Reasoning.
Proceedings of the IEEE/CVF International Conference on Computer Vision (ICCV)(2023)
摘要
Diverse visual stimuli can evoke various human affective states, which are usually manifested in an individual’s muscular actions and facial expressions. In lab-controlled emotion datasets, such a critical component (i.e., stimulus) was commonly designed in a limited way, making researchers incapable of generalizing the universal correlation and causation of stimulus-reaction as well as predicting possible emotions from context, timing, and relation. In this paper, we collected a large-scale spontaneous facial behavior database ReactioNet, which contains 1.1 million coupled stimulus-reaction tuples (visual/audio/caption from both stimuli and subjects). We introduce a new facial behavior detection scenario, Dyadic Relation Reasoning (DRR), which aims to detect facial actions by reasoning their relations with stimuli. By aggregating the dyadic information, our method essentially forms a relation prototype Universal Stimulus Reaction (U-SR), which encodes the low-order and high-order relationships between stimulus agents and facial reactions. A framework with both non-graph and graph modules is further developed to evaluate DRR-based facial action unit detection, facial expression recognition, and scene classification. Specifically, to learn "what" arouses a facial reaction, the non-graph module associates and projects the fine-grained stimulus-reaction features into common subspaces using cross-domain contrastive learning. To learn "how" stimulus-reaction pairs are mutually related, the graph module adopts Graph Convolution Network to represent, converge, and infer the dyadic U-SR relation under two relation assumptions (i.e., homophily and heterophily [68]). Extensive experiments demonstrate the effectiveness of the proposed work. The new dataset will be available for the research community.
更多查看译文
AI 理解论文
溯源树
样例
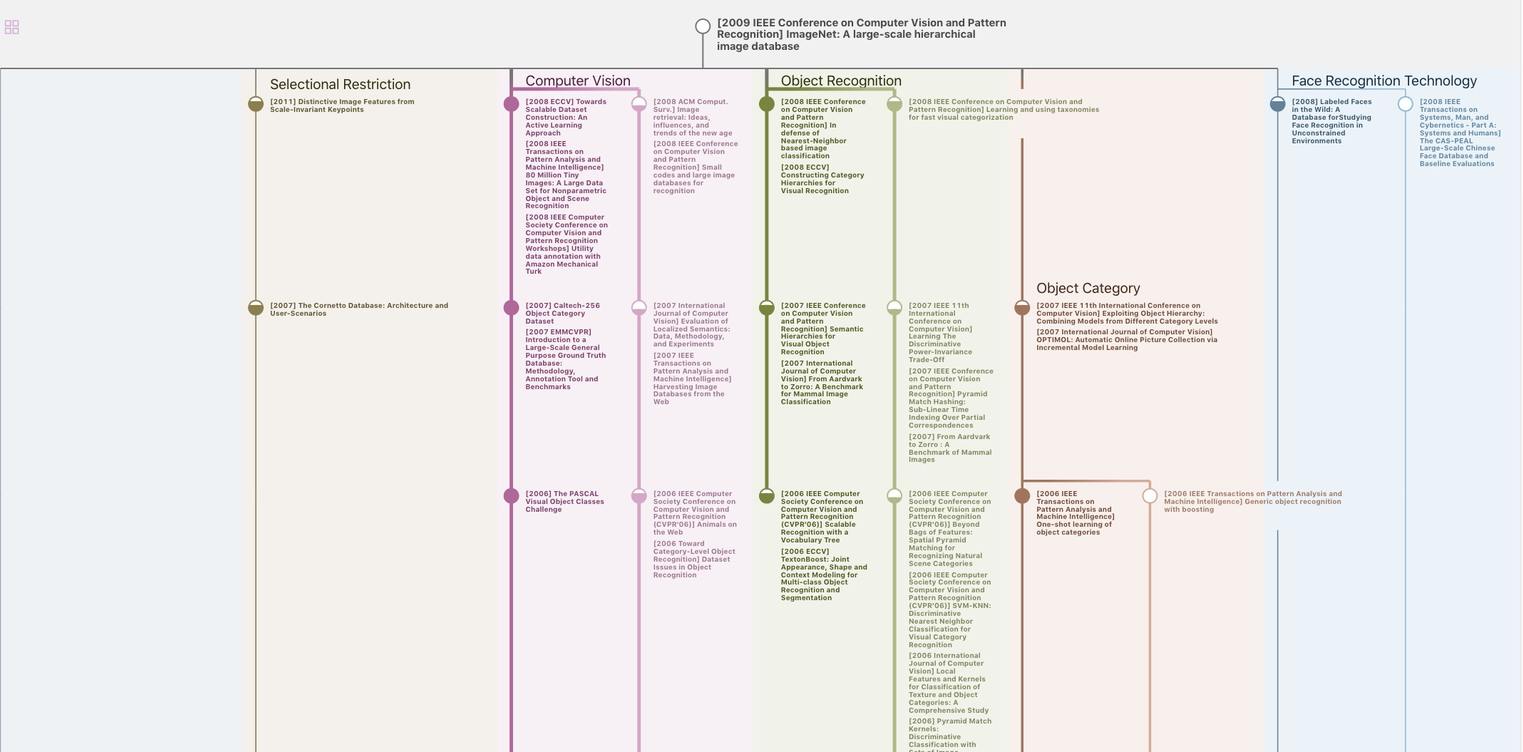
生成溯源树,研究论文发展脉络
Chat Paper
正在生成论文摘要