Semi-Supervised Semantic Segmentation under Label Noise via Diverse Learning Groups.
ICCV(2023)
摘要
Semi-supervised semantic segmentation methods use a small amount of clean pixel-level annotations to guide the interpretation of a larger quantity of unlabelled image data. The challenges of providing pixel-accurate annotations at scale mean that the labels are typically noisy, and this contaminates the final results. In this work, we propose an approach that is robust to label noise in the annotated data. The method uses two diverse learning groups with different network architectures to effectively handle both label noise and unlabelled images. Each learning group consists of a teacher network, a student network and a novel filter module. The filter module of each learning group utilizes pixel-level features from the teacher network to detect incorrectly labelled pixels. To reduce confirmation bias, we employ the labels cleaned by the filter module from one learning group to train the other learning group. Experimental results on two different benchmarks and settings demonstrate the superiority of our method over state-of-the-art approaches.
更多查看译文
AI 理解论文
溯源树
样例
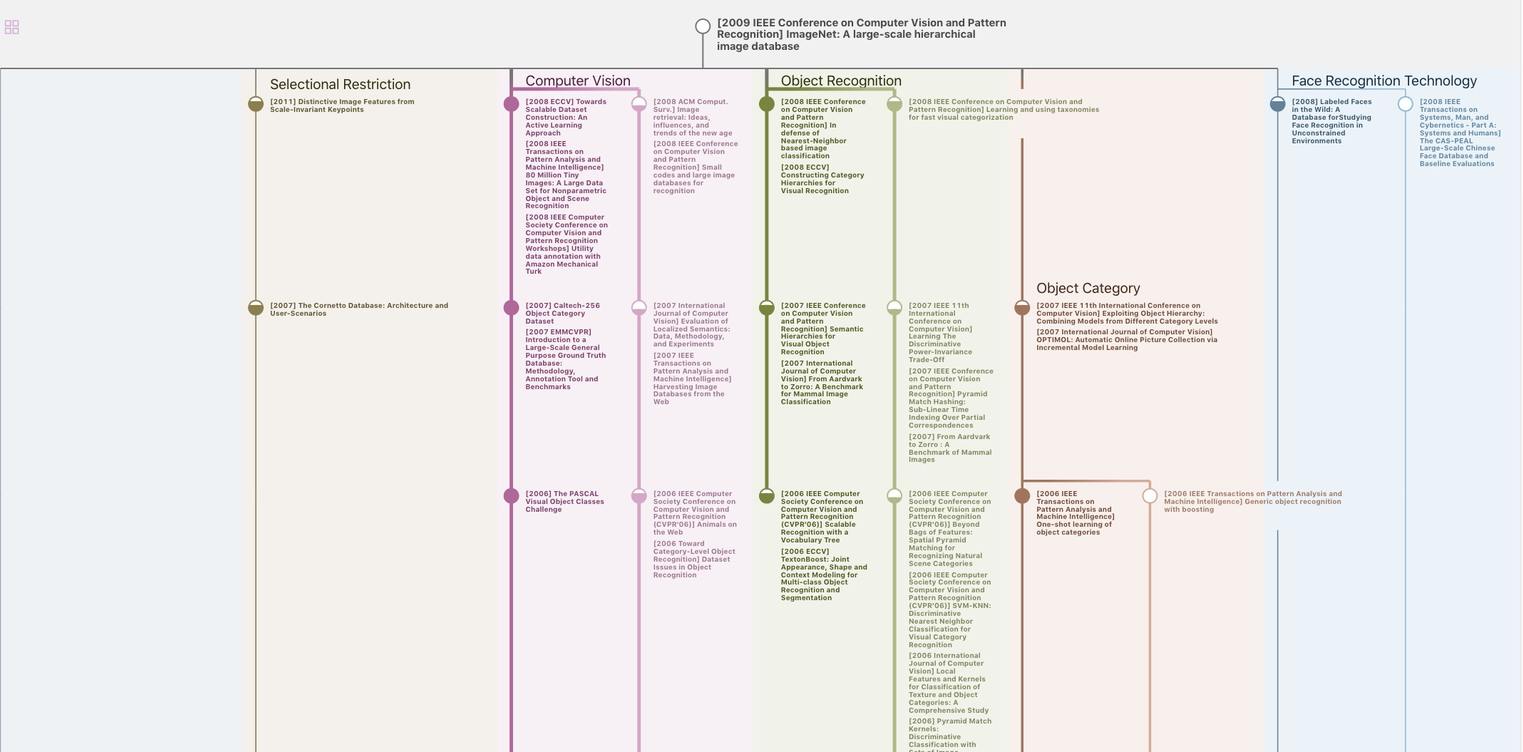
生成溯源树,研究论文发展脉络
Chat Paper
正在生成论文摘要