Generating Dynamic Kernels via Transformers for Lane Detection.
Proceedings of the IEEE/CVF International Conference on Computer Vision (ICCV)(2023)
摘要
State-of-the-art lane detection methods often rely on specific knowledge about lanes – such as straight lines and parametric curves – to detect lane lines. While the specific knowledge can ease the modeling process, it poses challenges in handling lane lines with complex topologies (e.g., dense, forked, curved, etc.). Recently, dynamic convolution-based methods have shown promising performance by utilizing the features from some key locations of a lane line, such as the starting point, as convolutional kernels, and convoluting them with the whole feature map to detect lane lines. While such methods reduce the reliance on specific knowledge, the kernels computed from the key locations fail to capture the lane line’s global structure due to its long and thin structure, leading to inaccurate detection of lane lines with complex topologies. In addition, the kernels resulting from the key locations are sensitive to occlusion and lane intersections. To overcome these limitations, we propose a transformer-based dynamic kernel generation architecture for lane detection. It utilizes a transformer to generate dynamic convolutional kernels for each lane line in the input image, and then detect these lane lines with dynamic convolution. Compared to the kernels generated from the key locations of a lane line, the kernels generated with the transformer can capture the lane line’s global structure from the whole feature map, enabling them to effectively handle occlusions and lane lines with complex topologies. We evaluate our method on three lane detection benchmarks, and the results demonstrate its state-of-the-art performance. Specifically, our method achieves an F1 score of 63.40 on OpenLane and 88.47 on CurveLanes, surpassing the state of the art by 4.30 and 2.37 points, respectively.
更多查看译文
AI 理解论文
溯源树
样例
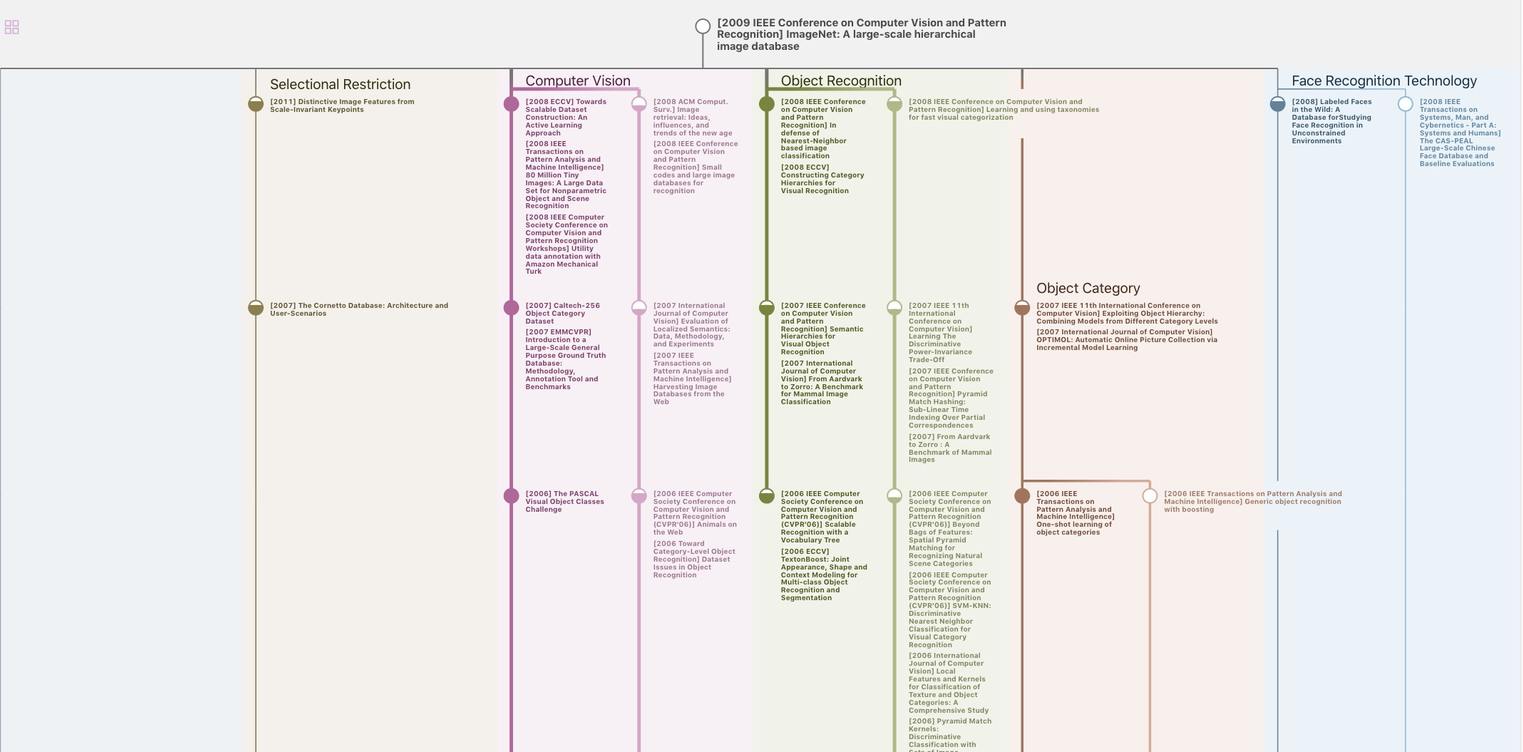
生成溯源树,研究论文发展脉络
Chat Paper
正在生成论文摘要