Task-aware Adaptive Learning for Cross-domain Few-shot Learning.
ICCV(2023)
摘要
Although existing few-shot learning works yield promising results for in-domain queries, they still suffer from weak cross-domain generalization. Limited support data requires effective knowledge transfer, but domain-shift makes this harder. Towards this emerging challenge, researchers improved adaptation by introducing task-specific parameters, which are directly optimized and estimated for each task. However, adding a fixed number of additional parameters fails to consider the diverse domain shifts between target tasks and the source domain, limiting efficacy. In this paper, we first observe the dependence of task-specific parameter configuration on the target task. Abundant task-specific parameters may over-fit, and insufficient task-specific parameters may result in under-adaptation – but the optimal task-specific configuration varies for different test tasks. Based on these findings, we propose the Task-aware Adaptive Network (TA
2
-Net), which is trained by reinforcement learning to adaptively estimate the optimal task-specific parameter configuration for each test task. It learns, for example, that tasks with significant domain-shift usually have a larger need for task-specific parameters for adaptation. We evaluate our model on Meta-dataset. Empirical results show that our model outperforms existing state-of-the-art methods. Our code is available at https://github.com/PRIS-CV/TA2-Net.
更多查看译文
AI 理解论文
溯源树
样例
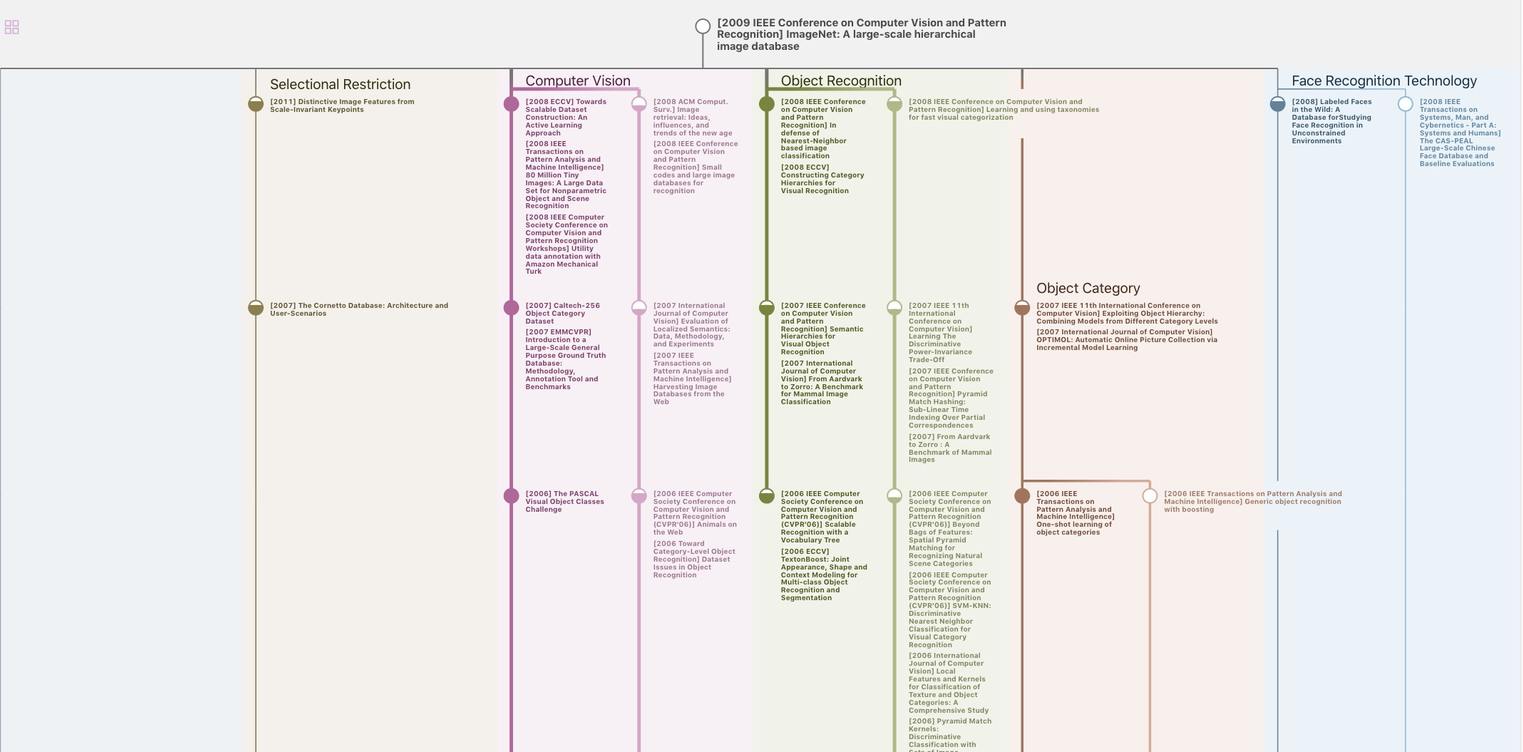
生成溯源树,研究论文发展脉络
Chat Paper
正在生成论文摘要